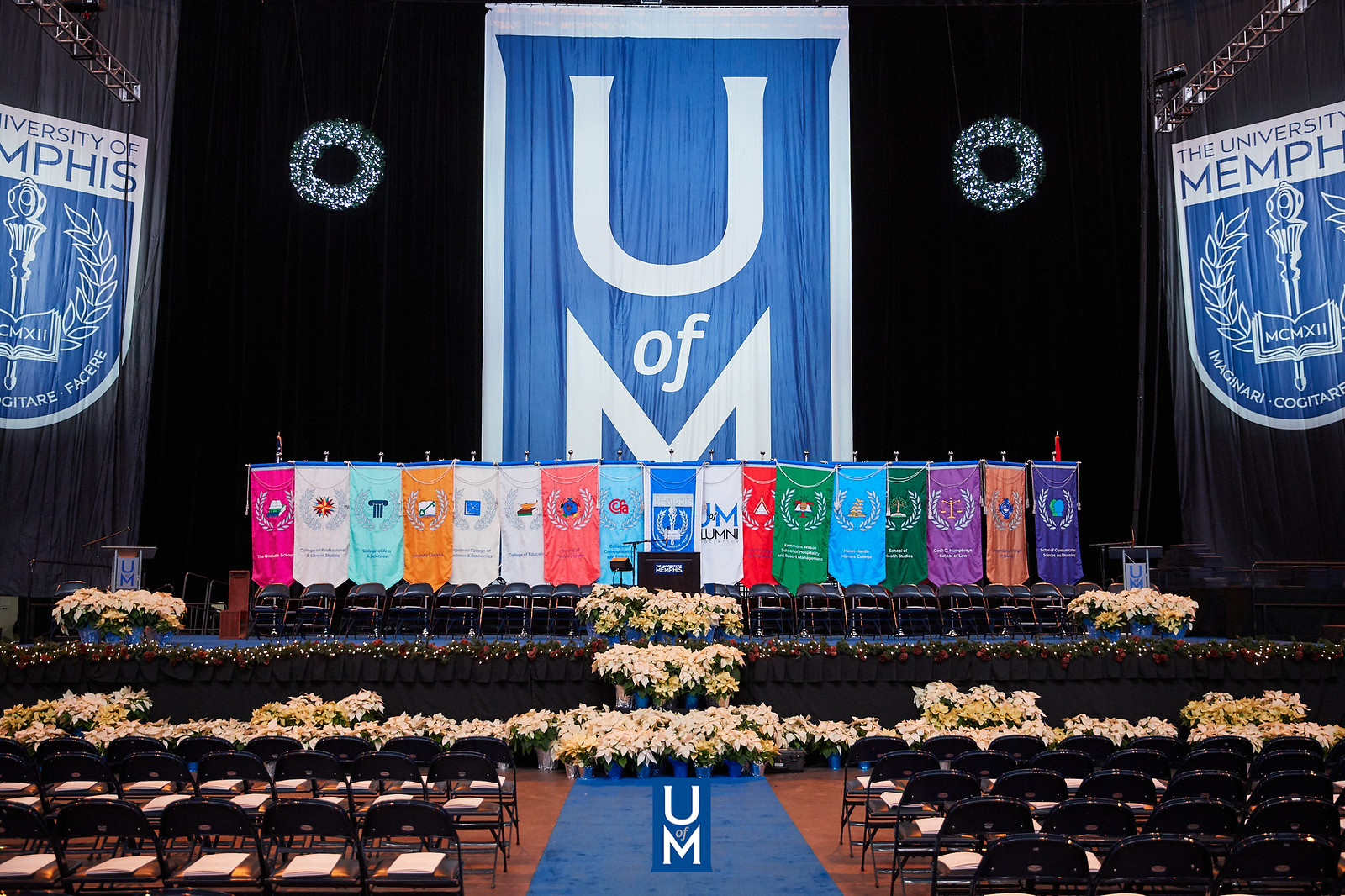
Electronic Theses and Dissertations
Identifier
195
Date
2010
Document Type
Thesis
Degree Name
Master of Science
Major
Computer Science
Committee Chair
Santosh Kumar
Committee Member
King-Ip Lin
Committee Member
Lan Wang
Abstract
Most of the existing real-time asset tracking systems involves frequent communication of a device placed on the object to be tracked with a fixed infrastructure, which rapidly depletes the battery of the tag, due to the high energy demand of communication. This thesis presents emove, a system implemented on a low cost tag node that can be attached to assets. An emove tag autonomously detects and classifies movement of assets while being in deep sleep mode for most of its life. It communicates with the infrastructure only when it confirms the movement detected is of interest. Our design emphasizes energy-efficient and compliance free usage. We conducted experiments to evaluate the performance of emove in real-life. We found that the accuracy of the classifier for an asset tracking application is 98%; the average time to detect a movement is 22.2 seconds. The average lifetime of the tag is increased from few months to more than a year. We obtained similar results by applying emove to a theft detection application.
Library Comment
Dissertation or thesis originally submitted to the local University of Memphis Electronic Theses & dissertation (ETD) Repository.
Recommended Citation
Krishna, Bhagavathy, "emove: Movement Detection and Classification for Tagged Assets on Embedded Platforms" (2010). Electronic Theses and Dissertations. 145.
https://digitalcommons.memphis.edu/etd/145
Comments
Data is provided by the student.