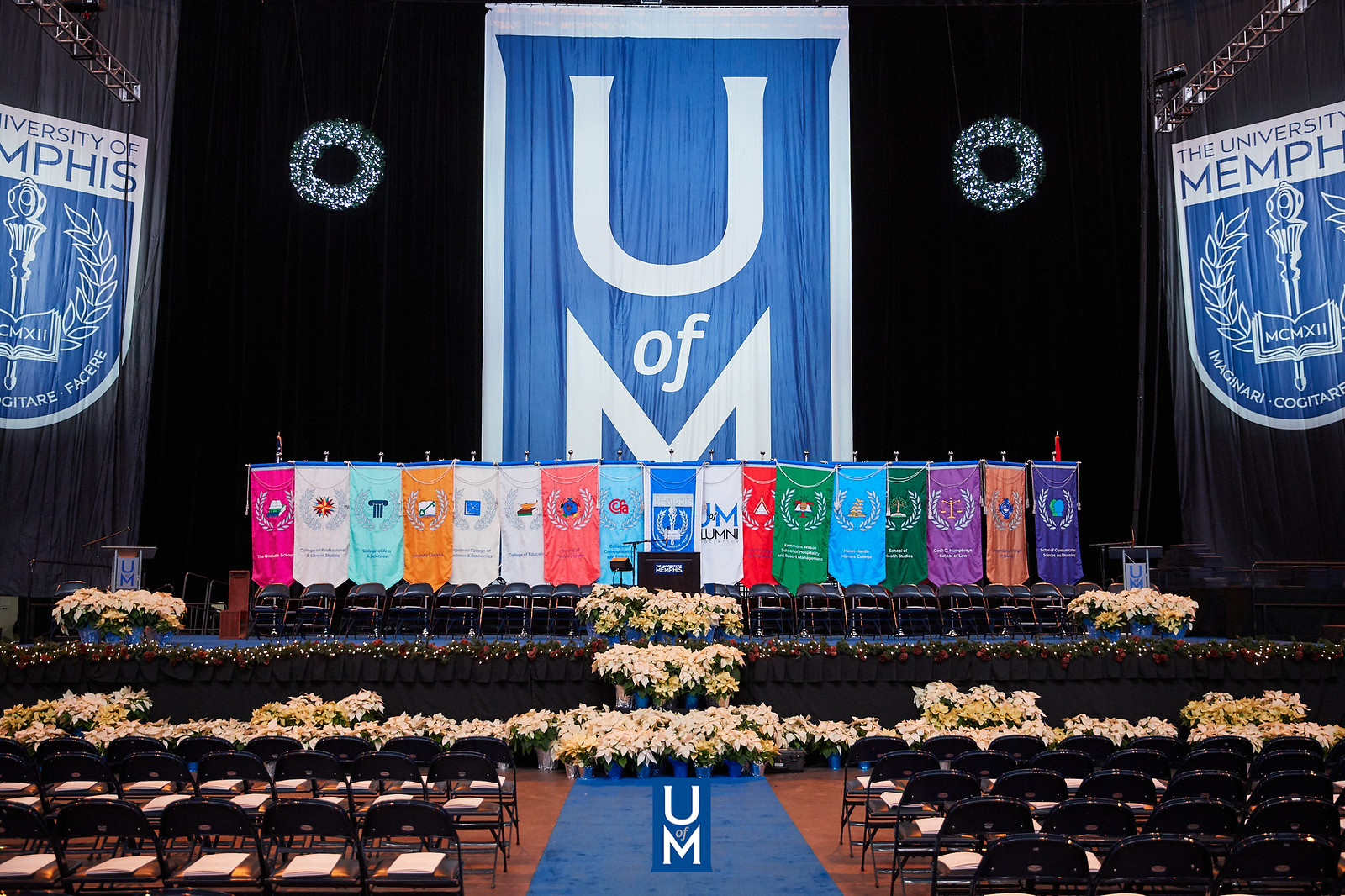
Electronic Theses and Dissertations
Identifier
6630
Date
2020
Document Type
Thesis
Degree Name
Master of Science
Major
Computer Science
Committee Chair
Nirman Kumar
Committee Member
Aaryani Tipirneni-Sajja
Committee Member
Deepak Venugopal
Abstract
Hepatic steatosis is the accumulation of fat in the liver, affecting about 25% of the world population. Steatosis can cause lipo-toxicity and eventually lead to fibrosis, cirrhosis and ultimately liver failure if timely interventions are not provided. So, early diagnosis and disease monitoring of steatosis is crucial to reduce morbidity and mortality.Chemical shift based Magnetic Resonance Imaging (MRI) techniques using single and dual R2*(transverse relaxation rate) models have been reported to quantify fat fraction (FF) for assessment of steatosis. However, there is no common consensus between these two models and current data is limited for which model is accurate to quantify FF. Fully characterizing the behavior of the modelsover the entire clinical range of hepatic steatosis is essential to determine the limits of each of the models. However, performing a systematic investigation of the R2*models in patient population is infeasible. This thesis presents a computational approach by building a Monte Carlo based model as an alternative way to examine the R2*-MRI models. A 3D liver volume with impenetrable fat spheres was simulated to mimic hepatic steatosis. The simulation of steatosis was done using realistic data obtained from automatic segmentation and characterization of fat droplets using liver biopsy images. MRI signals were synthesized in the virtual liver volume using Monte Carlo modeling approach. Finally, the R2*behavior was analyzed using both the single and dual R2*models and they were compared against in-vivo calibration to determine their accuracy. Predicted R2*values were within confidence bounds of the published in vivo calibration and single R2*modelshowed higher accuracy than dual R2*model to estimate FF. In conclusion, this research developed a computational framework for creating realistic hepatic steatosis model and synthesizing MRI signal and analyzing R2*behavior in the presence of fat. The developed computational methods will also be generalizable to create other tissue-specific models and study R2*behavior at higher field strengths, for testing new MRI pulse sequences and in presence of other co-existing pathologies such as hepatic iron overload.
Library Comment
Dissertation or thesis originally submitted to the local University of Memphis Electronic Theses & dissertation (ETD) Repository.
Recommended Citation
Shrestha, Utsav, "Quantitative Analysis and Monte Carlo Modeling of Fat-Mediated MRI Relaxation" (2020). Electronic Theses and Dissertations. 2123.
https://digitalcommons.memphis.edu/etd/2123
Comments
Data is provided by the student.