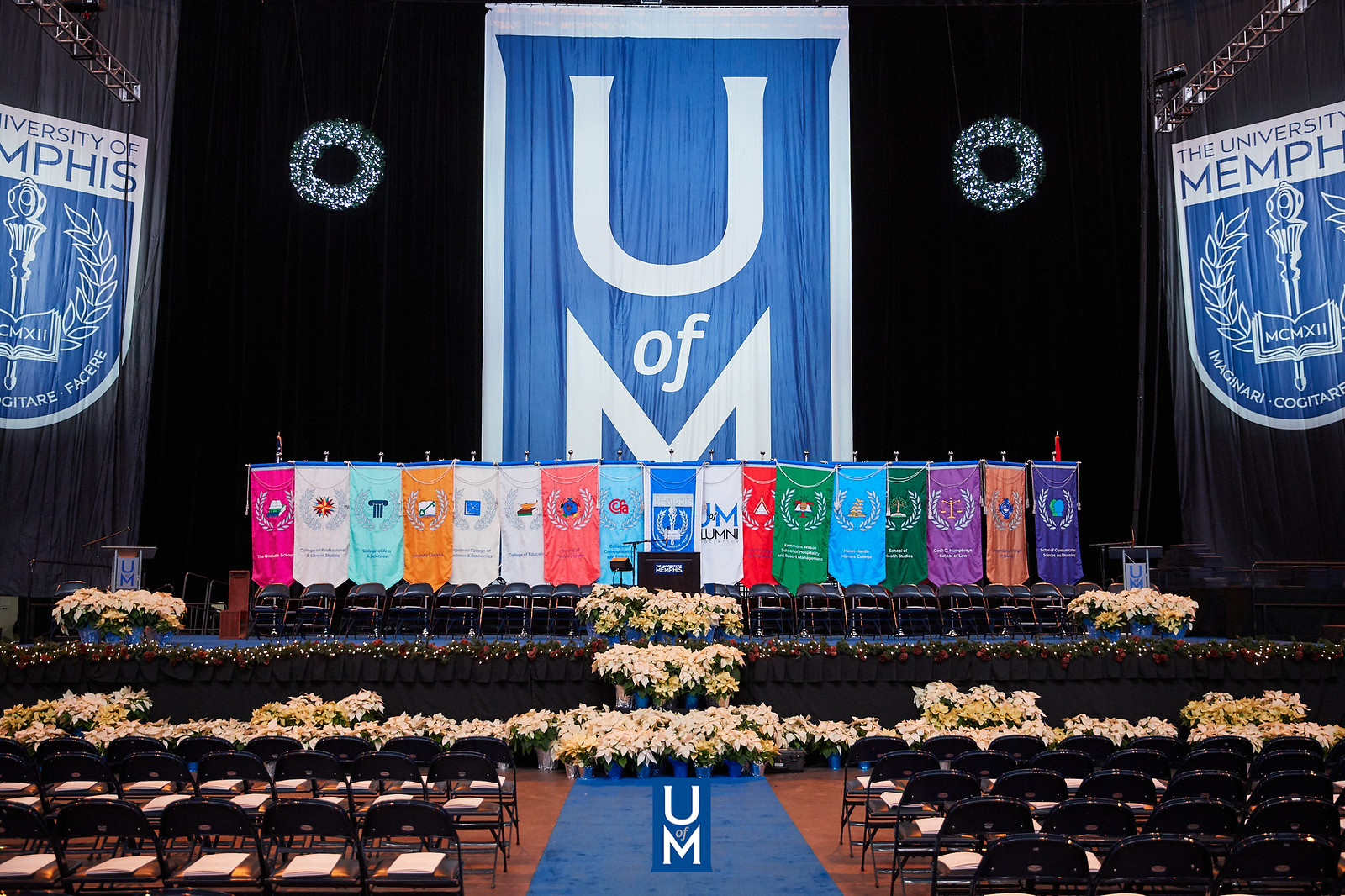
Electronic Theses and Dissertations
Identifier
6663
Date
2020
Document Type
Thesis
Degree Name
Master of Science
Major
Electrical and Computer Engr
Concentration
Computer Engineering
Committee Chair
Mohammed Yeasin
Committee Member
Gavin Bidelman
Committee Member
Faruk Ahmed
Abstract
Categorical perception (CP) of speech is a complex process reflecting individuals’ ability to perceive sound and is measured using response time (RT). The cognitive processes involved in mapping neural activities to behavioral response are stochastic and further compounded by individuality and variations. This thesis presents a data-driven approach and develops parameter optimized models to understand the relationship between cognitive events and behavioral response (e.g., RT). We introduce convolutional neural networks (CNN) to learn the representation from EEG recordings. In addition, we develop parameter optimized and interpretable models in decoding CP using two representations: 1) spatial-spectral topomaps and 2) evoked response potentials (ERP). We adopt state-of-the-art class discriminative visualization (GradCAM) tools to gain insights (as oppose to the’black box’ models) and building interpretable models. In addition, we develop a diverse set of models to account for the stochasticity and individual variations. We adopted weighted saliency scores of all models to quantify the learned representations’ effectiveness and utility in decoding CP manifested through behavioral response. Empirical analysis reveals that the γ band and early (∼ 0 - 200ms) and late (∼ 300 - 500ms) right hemisphere IFG engagement is critical in determining individuals’ RT. Our observations are consistent with prior findings, further validating the efficacy of our data-driven approach and optimized interpretable models.
Library Comment
Dissertation or thesis originally submitted to the local University of Memphis Electronic Theses & dissertation (ETD) Repository.
Recommended Citation
Moinuddin, Kazi Ashraf, "Decoding Perception of Speech from Behavioral Responses using Spatio-Temporal CNNs" (2020). Electronic Theses and Dissertations. 2147.
https://digitalcommons.memphis.edu/etd/2147
Comments
Data is provided by the student.