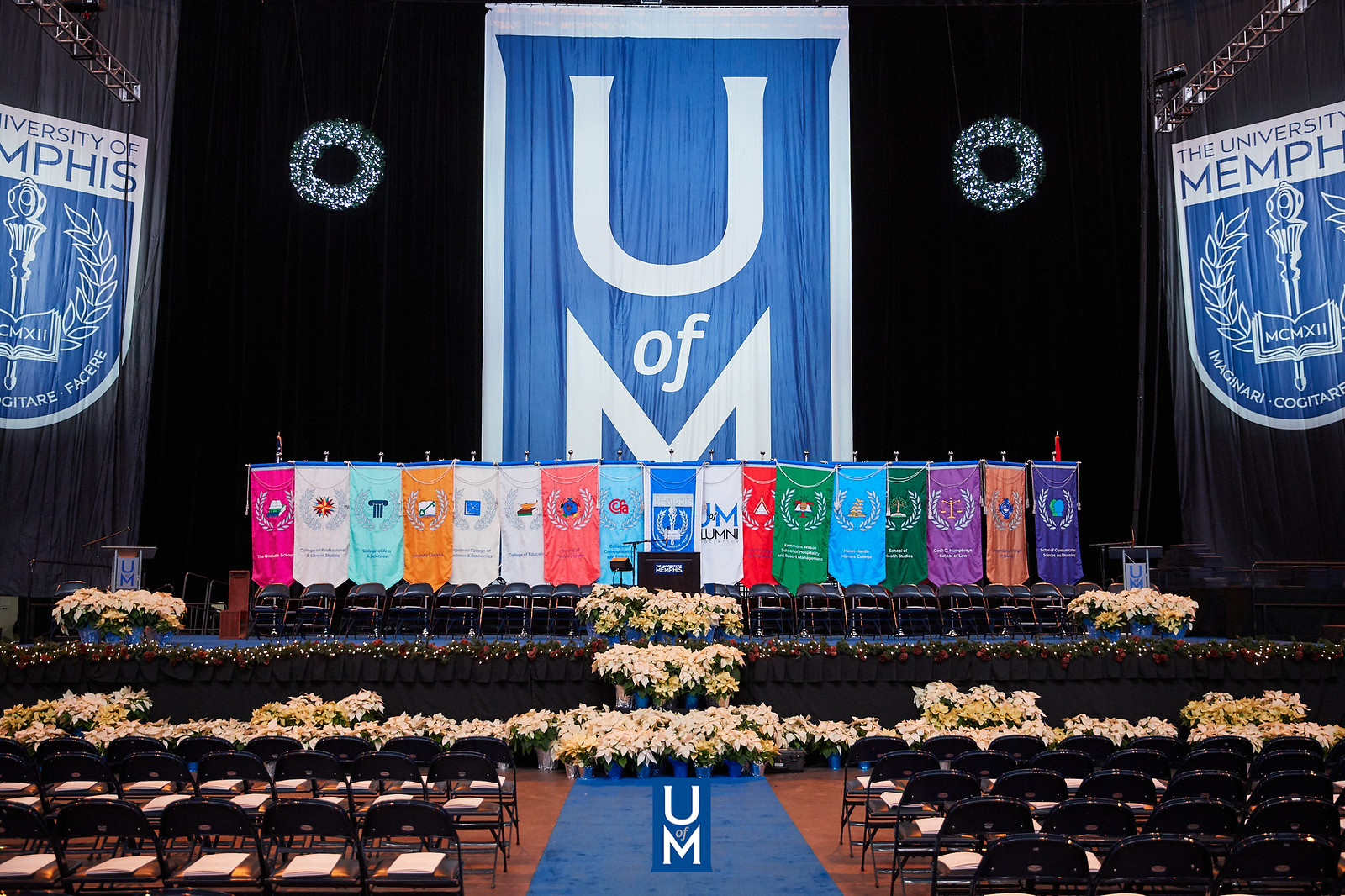
Electronic Theses and Dissertations
Identifier
101
Date
2010
Document Type
Thesis (Access Restricted)
Degree Name
Master of Science
Major
Computer Science
Committee Chair
Dipankar Dasgupta
Committee Member
King-Ip Lin
Committee Member
Vinhthuy Phan
Abstract
Machine learning based classification techniques are being used in a growing number of security applications for anomaly detection related purposes. This is due to the adjusting capability and novel anomaly detection ability of machine learning. Previous works have shown that very simple classification techniques like linear classifiers can easily be learned and attacked by the adversary. This work addresses the adversarial learning possibility of nonlinear classifiers. It has been illustrated in this work that, nonlinear classifiers in general cannot be learned. However, special cases of nonlinearity have been addressed in which adversarial learning has been considered with some heuristic approaches.Another kind of attack on machine learning systems is the adversarial influence on learning. The adversary may change the distribution of data that is used in learning. In this work, the performance of a particular learning system has been studied against such kind of influence.
Library Comment
Dissertation or thesis originally submitted to the local University of Memphis Electronic Theses & dissertation (ETD) Repository.
Recommended Citation
Saha, Sudip, "Use of Linear and Nonlinear Classifiers against Adversarial Learning and Influence" (2010). Electronic Theses and Dissertations. 2301.
https://digitalcommons.memphis.edu/etd/2301
Comments
Data is provided by the student.