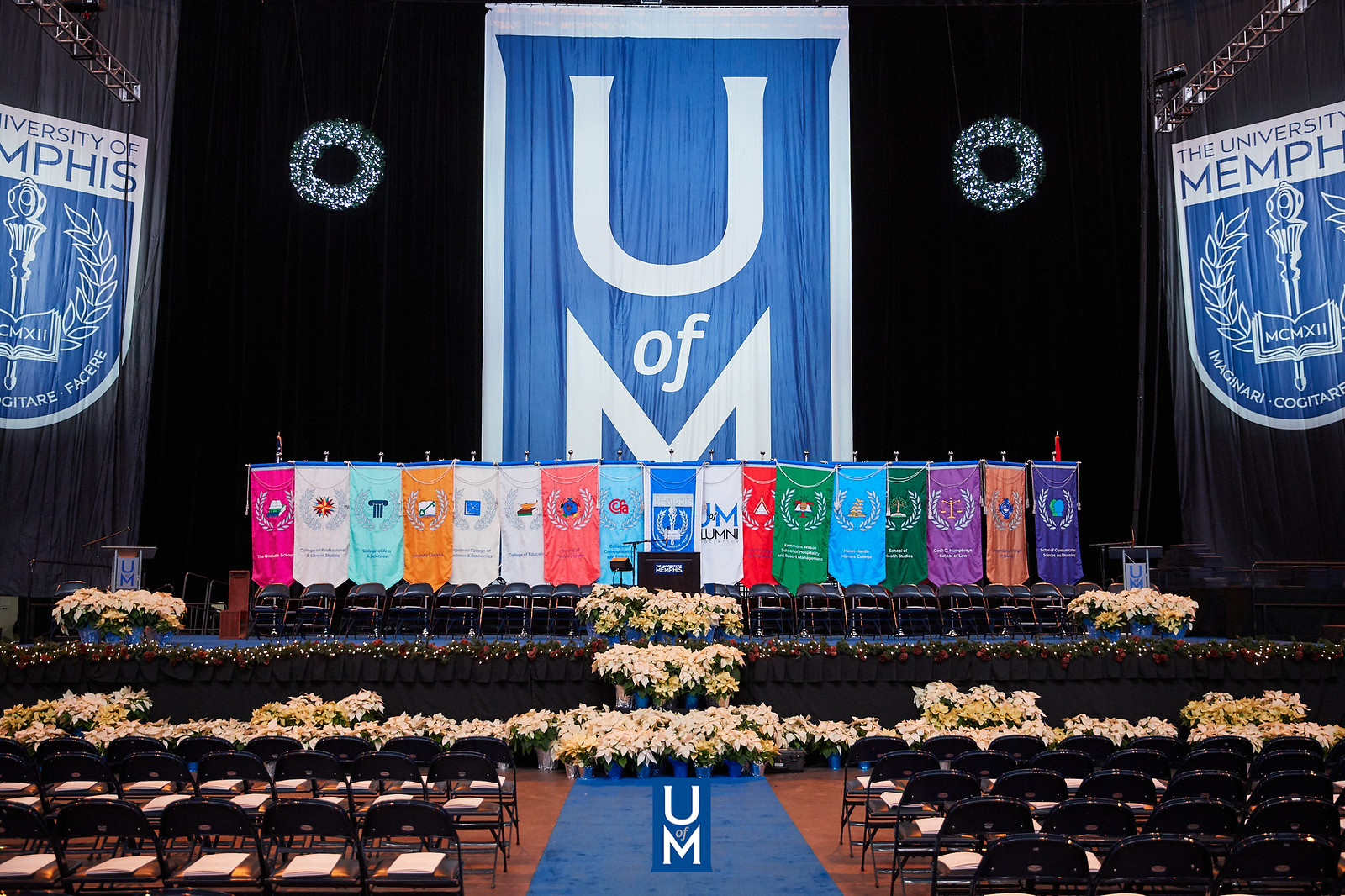
Electronic Theses and Dissertations
COMPUTATIONAL JOURNEY ACROSS MULTIPHYSICS AND MULTITEMPORAL SCALES: FROM SLOW TECTONIC MOVEMENT TO FAST EARTHQUAKE SLIP
Data is provided by the student.
Abstract
Understanding how mechanical and thermal energies are stored or dissipated in a tectonic system bears fundamental importance. However, the fact that mechanical deformation can directly impact the energy balance is often ignored, and thus potential effects on the evolution of many tectonic systems have not been quantified. In the first project, I derive an energy balance equation that accounts for deformations impact on temperature change. I then develop numerical models of normal fault evolution that take into account the deformation energetics. The modeling results show that temperature changes due to deformation promote the formation of secondary faults and an elongated core complex -exposures of deep crust exhumed in association with largely extension.Long-term tectonic activities like large-scale fault evolution occur over a period of millions of years but still can interact with short-term events like seconds- to minutes-long earthquakes. Understanding the interactions between them is crucial for better understanding the phenomena of respective time scales as stand-alone problems as well as in a unifying framework. As an effort to link properly rupture propagation with long-term tectonic processes, I investigate in the second project whether stress states and material properties from a tectonic model provide reasonable initial conditions and parameters for a rupture simulation. The results show that heterogeneous stress patterns along a fault are responsible for creating a high-velocity rupture around 5 km depth also produce complex slip pattern along the fault. Peak ground velocity is high on the hanging wall side while low on the footwall.In real geological systems, a fault is rough and geometrically complex. Numerous simulations are required in the trial and error approach to getting optimal parameters necessary for breaking such complex faults. This process is, however, computationally expensive. I develop two predictive models using two machine learning algorithms, to predict the rupture status. The models are so computationally efficient that they can predict 400 rupture status within a fraction of a second. Results show that both of the models can predict the rupture status with 81% accuracy.