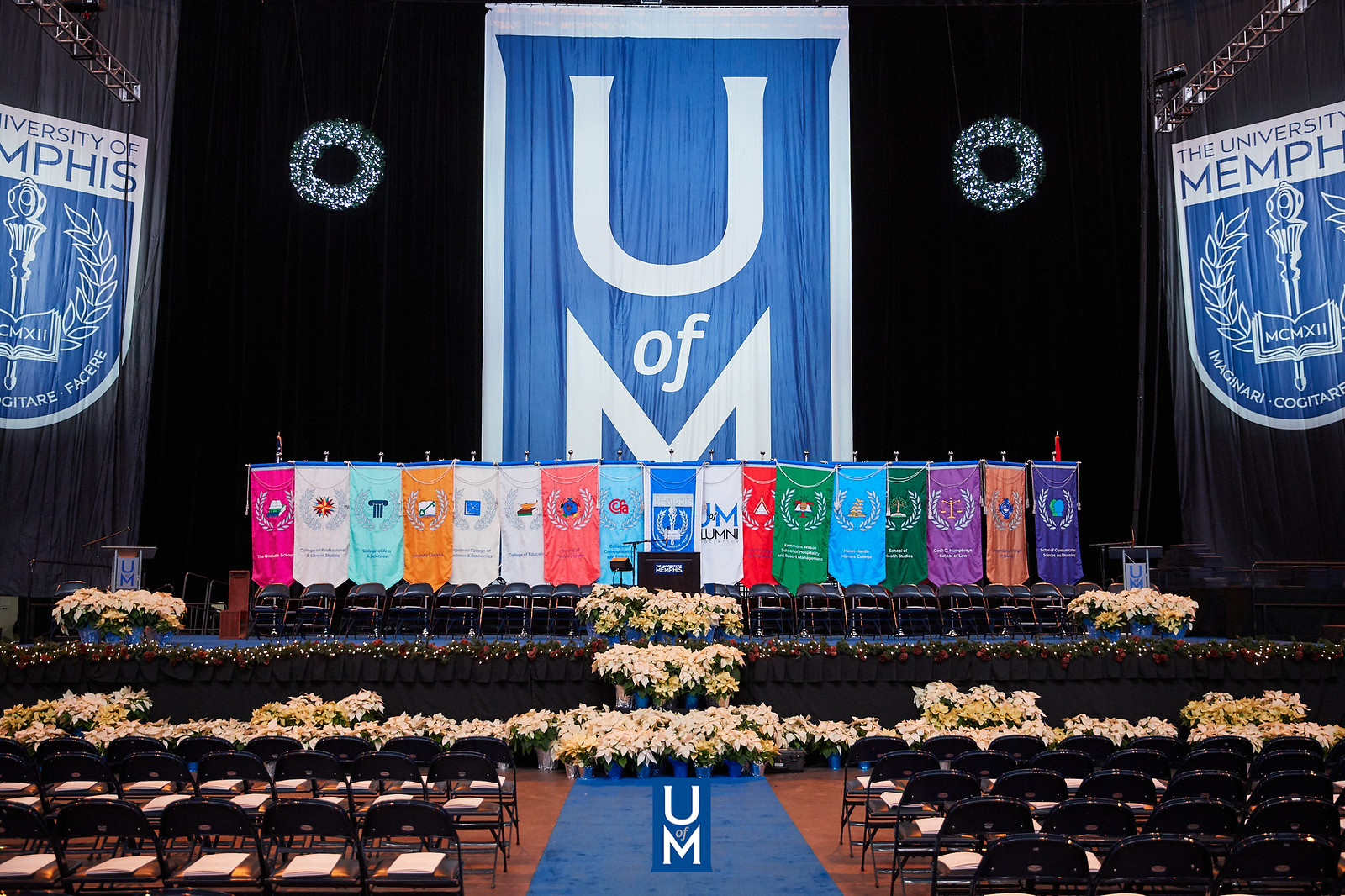
Electronic Theses and Dissertations
Date
2019
Document Type
Dissertation
Degree Name
Doctor of Philosophy
Department
Civil Engineering
Committee Chair
Charles Camp
Committee Member
Shahram Pezeshk
Committee Member
Adel Abdelnaby
Committee Member
Mihalis Gkolias
Abstract
The need for developing an economical and efficient quality assessment system for pave-ment motivates this study to take advantage of available new technologies and provide a novel approach to address this need. In this study, the utility of using Google Street View (GSV) for evaluating the quality of pavement is investigated. GSV is a technology featured in Google Maps and Google Earth that provides interactive panoramas along many streets throughout the world. This technology provides a large data set of pavement images that can be used for pavement evaluation. Advanced deep learning algorithms are utilized to automate the pavement assessment process of these GSV images. These algorithms autonomously learn to find the important fea-tures in a data set to perform a particular task. A convolutional neural network (CNN) is one of the deep learning algorithms that has been shown to be very effective in learning from digital im-ages. Several CNNs are used in this study to perform image classification on GSV pavement im-ages. Training an effective CNN with many learning parameters requires a large image data set. To provide the required data for training a CNN, a large number of pavement images are ex-tracted from GSV are then divided to smaller image patches to form a larger data set. Each image patch is visually classified into different categories of pavement cracks based on the standard practice. A comparative study of pavement quality assessment is conducted between the results of the CNN classified images patches obtained from GSV and those from a sophisticated com-mercial visual inspection company. The result of the comparison indicates the feasibility and ef-fectiveness of using GSV images for pavement evaluation. An effective CNN is designed and trained on the image data set to automate the crack detection process. The trained network is then tested on a new data set. The results of this study show that the designed CNN is effective in classifying the pavement images into different defined crack categories.EP LEARNING-BASED VISUAL CRACK DETECTION USING GOOGLE STREET VIEW IMAGES
Library Comment
Dissertation or thesis originally submitted to ProQuest
Recommended Citation
Maniat, Mohsen, "DEEP LEARNING-BASED VISUAL CRACK DETECTION USING GOOGLE STREET VIEW IMAGES" (2019). Electronic Theses and Dissertations. 2660.
https://digitalcommons.memphis.edu/etd/2660
Comments
Data is provided by the student.