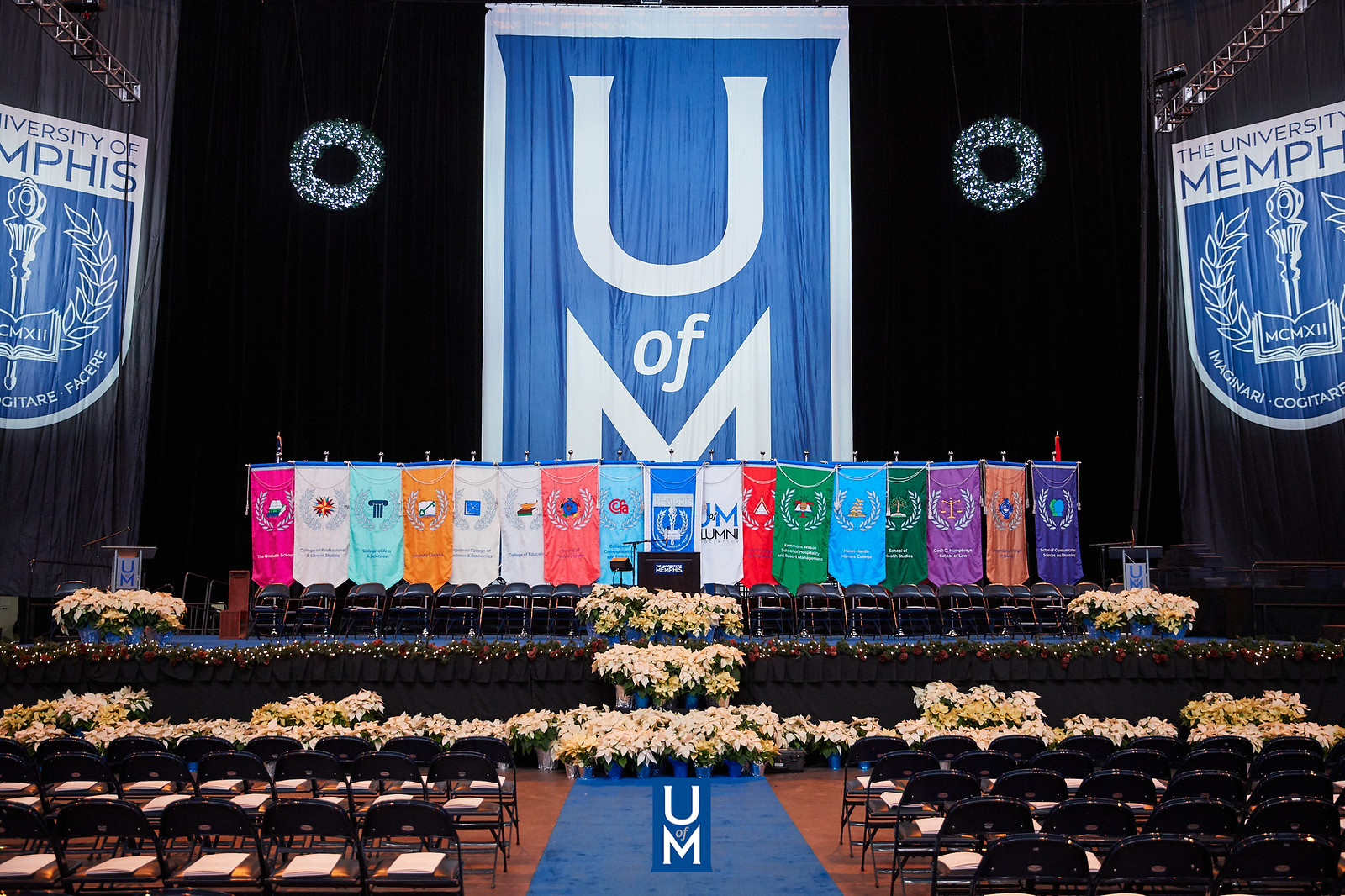
Electronic Theses and Dissertations
Date
2023
Document Type
Dissertation
Degree Name
Doctor of Philosophy
Department
Computer Science
Committee Chair
Santosh Kumar
Committee Member
Myounggyu MW Won
Committee Member
Vivek VS Shetty
Committee Member
Weizi WL Li
Abstract
Continuous advancements in wrist-worn sensors have opened up exciting possibilities for real-time monitoring of individuals' daily behaviors, with the aim of promoting healthier, more organized, and efficient lives. Understanding the duration of specific daily behaviors has become of interest to individuals seeking to optimize their lifestyles. However, there is still a research gap when it comes to monitoring short-duration behaviors that have a significant impact on health using wrist-worn inertial sensors in natural environments. These behaviors often involve repetitive micro-events that last only a few seconds or even microseconds, making their detection and analysis challenging. Furthermore, these micro-events are often surrounded by non-repetitive boundary events, further complicating the identification process. Effective detection and timely intervention during these short-duration behaviors are crucial for designing personalized interventions that can positively impact individuals' lifestyles. To address these challenges, this dissertation introduces three models: mORAL, mTeeth, and Brushing Prompt. These models leverage wrist-worn inertial sensors to accurately infer short-duration behaviors, identify repetitive micro-behaviors, and provide timely interventions related to oral hygiene. The dissertation's contributions extend beyond the development of these models. Firstly, precise and detailed labels for each brief and micro-repetitive behavior are acquired to train and validate the models effectively. This involved meticulous marking of the exact start and end times of each event, including any intervening pauses, at a second-level granularity. A comprehensive scientific research study was conducted to collect such data from participants in their free-living natural environments. Secondly, a solution is proposed to address the issue of sensor placement variability. Given the different positions of the sensor within a wristband and variations in wristband placement on the wrist, the model needs to determine the relative configuration of the inertial sensor accurately. Accurately determining the relative positioning of the inertial sensor with respect to the wrist is crucial for the model to determine the orientation of the hand. Additionally, time synchronization errors between sensor data and associated video, despite both being collected on the same smartphone, are addressed through the development of an algorithm that tightly synchronizes the two data sources without relying on an explicit anchor event. Furthermore, an event-based approach is introduced to identify candidate segments of data for applying machine learning models, outperforming the traditional fixed window-based approach. These candidate segments enable reliable detection of brief daily behaviors in a computationally efficient manner suitable for real-time. The dissertation also presents a computationally lightweight method for identifying anchor events using wrist-worn inertial sensors. Anchor events play a vital role in assigning unambiguous labels in a fixed-length window-based approach to data segmentation and effectively demarcating transitions between micro-repetitive events. Significant features are extracted, and explainable machine learning models are developed to ensure reliable detection of brief daily and micro-repetitive behaviors. Lastly, the dissertation addresses the crucial factor of the opportune moment for intervention during brief daily behaviors using wrist-worn inertial sensors. By leveraging these sensors, users can receive timely and personalized interventions to enhance their performance and improve their lifestyles. Overall, this dissertation makes substantial contributions to the field of real-time monitoring of short-duration behaviors. It tackles various technical challenges, provides innovative solutions, and demonstrates the potential for wrist-worn sensors to facilitate effective interventions and promote healthier behaviors. By advancing our understanding of these behaviors and optimizing intervention strategies, this research has the potential to significantly impact individuals' well-being and contribute to the development of personalized health solutions.
Library Comment
Dissertation or thesis originally submitted to ProQuest
Notes
Open Access
Recommended Citation
Akther, Sayma, "AI Modeling Approaches for Detecting, Characterizing, and Predicting Brief Daily Behaviors such as Toothbrushing using Wrist Trackers." (2023). Electronic Theses and Dissertations. 2987.
https://digitalcommons.memphis.edu/etd/2987
Comments
Data is provided by the student.