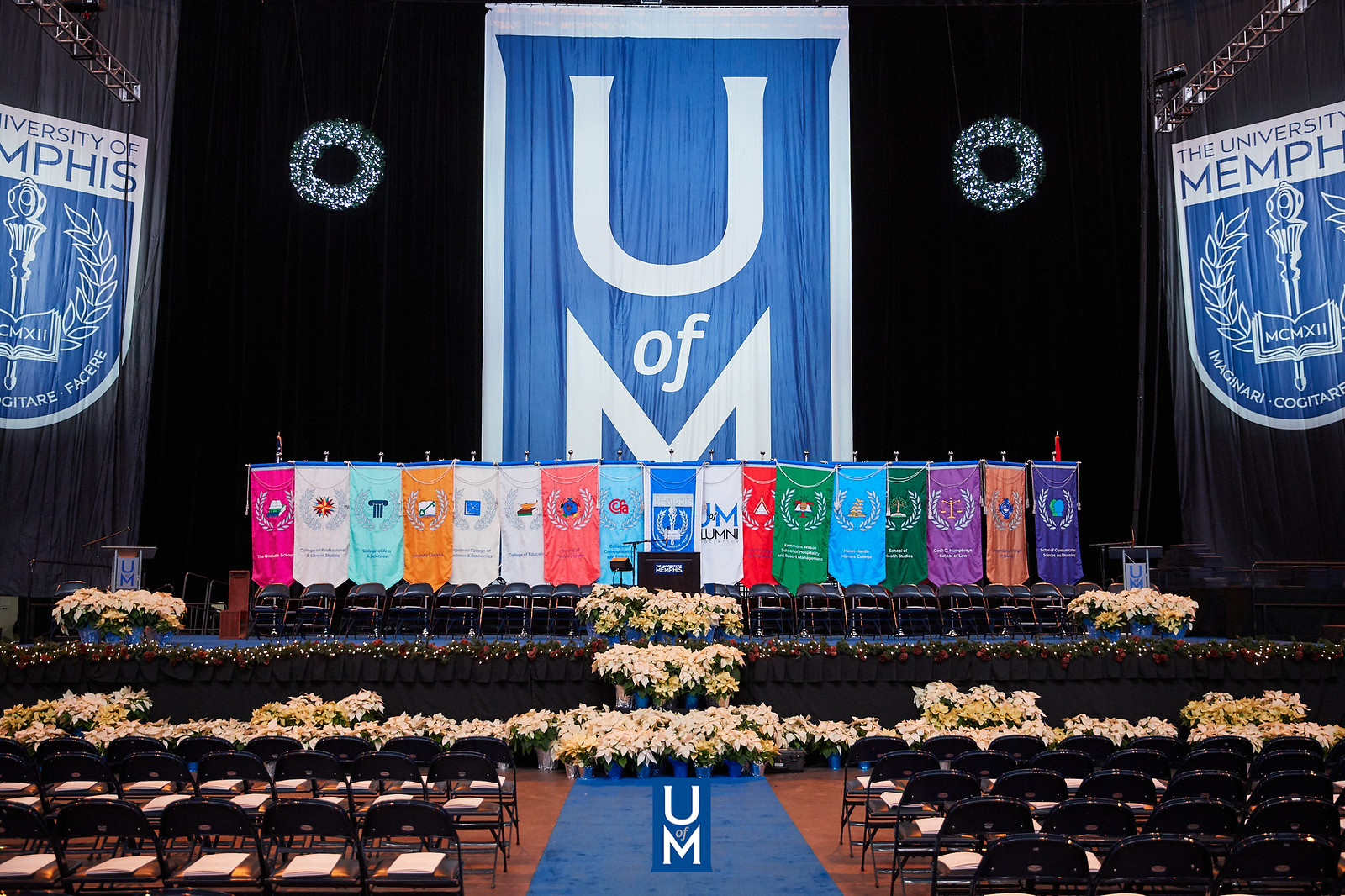
Electronic Theses and Dissertations
Date
2023
Document Type
Thesis
Degree Name
Master of Science
Department
Electrical & Computer Engineering
Committee Chair
Chrysanthe Preza
Committee Member
Mohammadreza Davoodi
Committee Member
Xiaofei Zhang
Abstract
Recent developments using deep learning (DL) super-resolution in structured-illumination microscopy (SIM), have improved speed in two-dimensional (2D) image restoration and minimized the impact of noise. We have extended this 2D DL technique to 3D by augmenting the 2D convolutional layers to 3D convolutional layers in a 3D U-Net DL network. We demonstrate experimentally that this extension improves lateral and axial resolution in the final 3D restoration compared to the resolution achieved by axially stacking the outputs of the 2D U-Net. To achieve this, we performed 3D processing on data acquired using 3D Structured Illumination Microscopy (3D-SIM) of subcellular biological samples. This is accomplished by splitting the data for training, validation, and testing followed by training a 3D reconstruction machine learning algorithm. We verify lateral and axial super-resolution improvement in 3D U-Net output by analyzing super-resolution quantitative performance metrics and intensity plots of our test data compared to ground truth images obtained from the traditional 3D-SIM restoration. These comparison results are consistent with those that have been reported using conventional techniques for 2D and 3D processing of a similar dataset.
Library Comment
Dissertation or thesis originally submitted to ProQuest
Notes
Open Access
Recommended Citation
Kebede, Bereket, "COMPARISON OF 2D AND 3D-BASED U-NET ARCHITECTURES FOR IMAGE RESTORATION IN STRUCTURED ILLUMINATION MICROSCOPY" (2023). Electronic Theses and Dissertations. 2989.
https://digitalcommons.memphis.edu/etd/2989
Comments
Data is provided by the student.