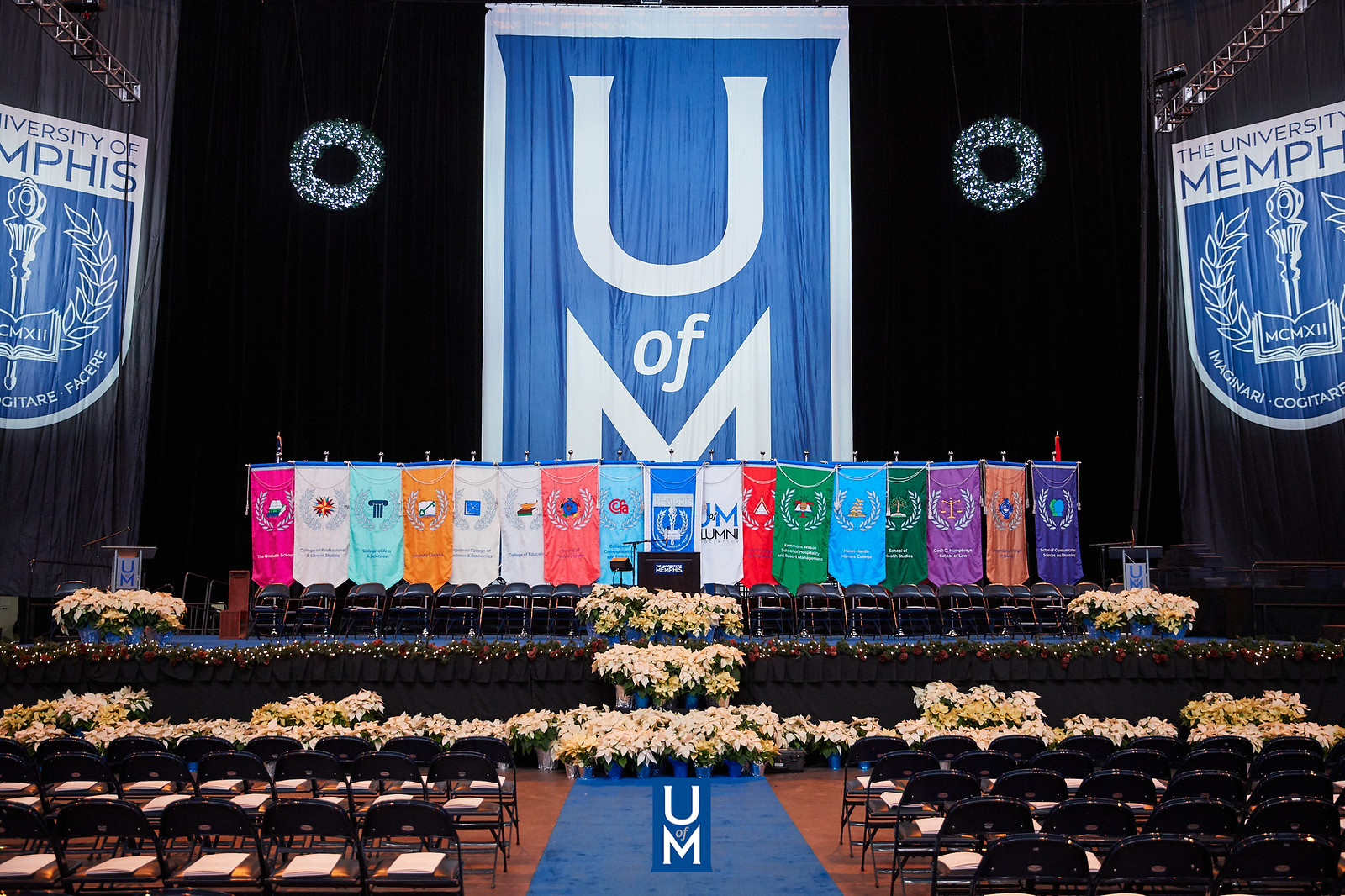
Electronic Theses and Dissertations
Date
2023
Document Type
Dissertation
Degree Name
Doctor of Philosophy
Department
Business Administration
Committee Chair
Huigang Liang
Committee Member
Mark Gillenson
Committee Member
Yinan Yu
Committee Member
Joseph Zhang
Abstract
Artificial Intelligence (AI) has generated significant interest due to its potential to augment human intelligence. However, user attitudes towards AI are diverse, with some individuals embracing it enthusiastically while others harbor concerns and actively avoid its use. This two essays' dissertation explores the reasons behind user aversion to AI. In the first essay, I develop a concise research model to explain users' AI aversion based on the theory of effective use and the adaptive structuration theory. I then employ an online experiment to test my hypotheses empirically. The multigroup analysis by Structural Equation Modeling shows that users' perceptions of human dissimilarity, AI bias, and social influence strongly drive AI aversion. Moreover, I find a significant difference between the simple and the complex task groups. This study reveals why users avert using AI by systematically examining the factors related to technology, user, task, and environment, thus making a significant contribution to the emerging field of AI aversion research. Next, while trust and distrust have been recognized as influential factors shaping users' attitudes towards IT artifacts, their intricate relationship with task characteristics and their impact on AI aversion remains largely unexplored. In my second essay, I conduct an online randomized controlled experiment on Amazon Mechanical Turk to bridge this critical research gap. My comprehensive analytic approach, including structural equation modeling (SEM), ANOVA, and PROCESS conditional analysis, allowed me to shed light on the intricate web of factors influencing users' AI aversion. I discovered that distrust and trust mediate between task complexity and AI aversion. Moreover, this study unveiled intriguing differences in these mediated relationships between subjective and objective task groups. Specifically, my findings demonstrate that, for objective tasks, task complexity can significantly increase aversion by reducing trust and significantly decrease aversion by reducing distrust. In contrast, for subjective tasks, task complexity only significantly increases aversion by enhancing distrust. By considering various task characteristics and recognizing trust and distrust as vital mediators, my research not only pushes the boundaries of the human-AI literature but also significantly contributes to the field of AI aversion.
Library Comment
Dissertation or thesis originally submitted to ProQuest
Notes
Embargoed until 12/28/2023
Recommended Citation
Rahman, Md Jabir, "Unveiling AI Aversion: Understanding Antecedents and Task Complexity Effects" (2023). Electronic Theses and Dissertations. 3068.
https://digitalcommons.memphis.edu/etd/3068
Comments
Data is provided by the student.