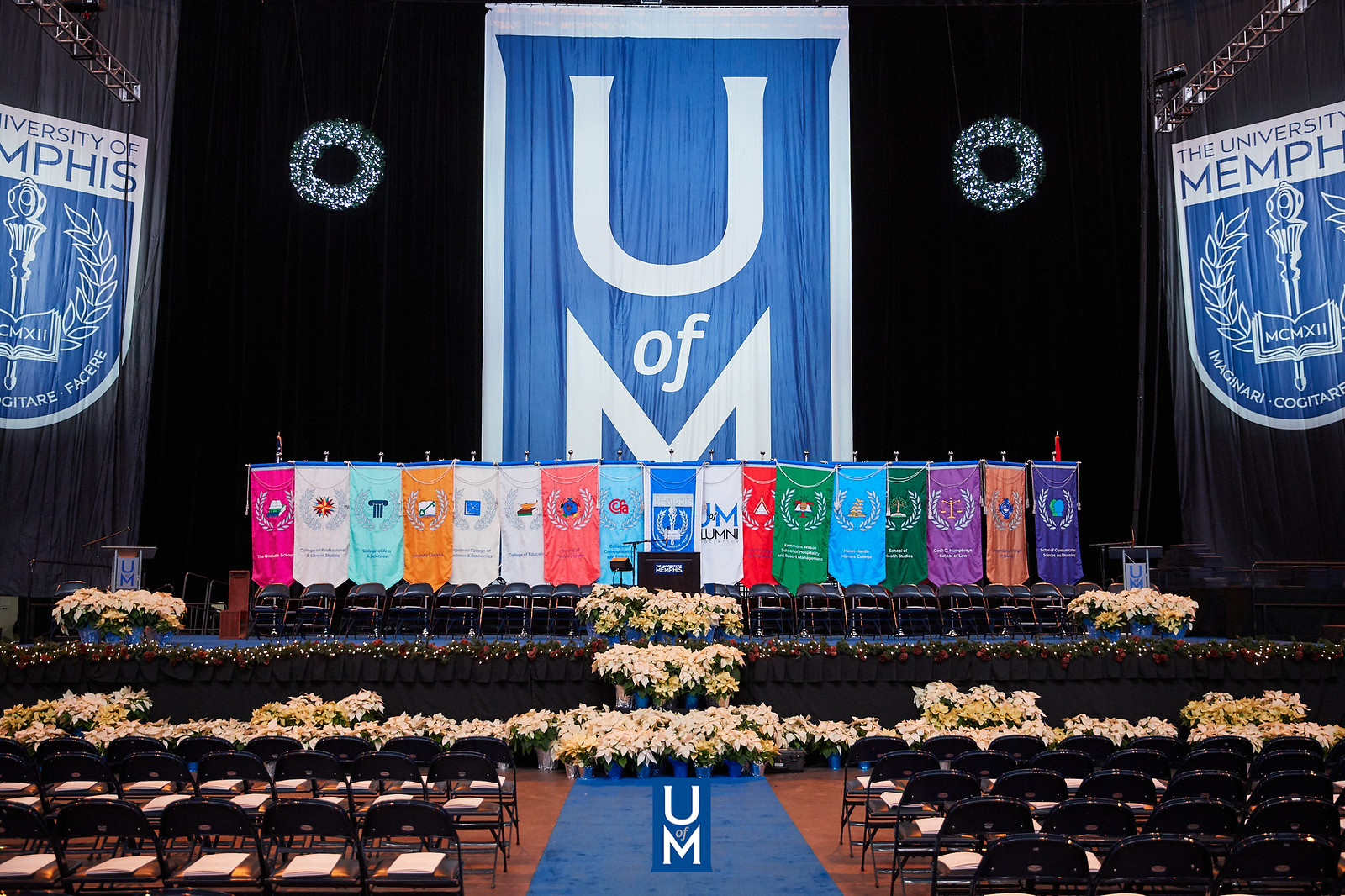
Electronic Theses and Dissertations
Date
2023
Document Type
Dissertation
Degree Name
Doctor of Philosophy
Department
Computer Science
Committee Chair
Santosh Kumar
Committee Member
Nirman Kumar
Committee Member
Myounggyu Won
Committee Member
Xiaolei Huang
Abstract
Estimating the imminent risk of adverse health behaviors provides opportunities for developing effective behavioral intervention mechanisms to prevent the occurrence of the target behavior. One of the key goals is to find opportune moments for intervention by passively detecting the rising risk of an imminent adverse behavior. Significant progress in mobile health research and the ability to continuously sense internal and external states of individual health and behavior has paved the way for detecting diverse risk factors from mobile sensor data. The next frontier in this research is to account for the combined effects of these risk factors to produce a composite risk score of adverse behaviors using wearable sensors convenient for daily use. Developing a machine learning-based model for assessing the risk of smoking lapse in the natural environment faces significant outstanding challenges requiring the development of novel and unique methodologies for each of them. The first challenge is coming up with an accurate representation of noisy and incomplete sensor data to encode the present and historical influence of behavioral cues, mental states, and the interactions of individuals with their ever-changing environment. The next noteworthy challenge is the absence of confirmed negative labels of low-risk states and adequate precise annotations of high-risk states. Finally, the model should work on convenient wearable devices to facilitate widespread adoption in research and practice. In this dissertation, we develop methods that account for the multi-faceted nature of smoking lapse behavior to train and evaluate a machine learning model capable of estimating composite risk scores in the natural environment. We first develop mRisk, which combines the effects of various mHealth biomarkers such as stress, physical activity, and location history in producing the risk of smoking lapse using sequential deep neural networks. We propose an event-based encoding of sensor data to reduce the effect of noises and then present an approach to efficiently model the historical influence of recent and past sensor-derived contexts on the likelihood of smoking lapse. To circumvent the lack of confirmed negative labels (i.e., annotated low-risk moments) and only a few positive labels (i.e., sensor-based detection of smoking lapse corroborated by self-reports), we propose a new loss function to accurately optimize the models. We build the mRisk models using biomarker (stress, physical activity) streams derived from chest-worn sensors. Adapting the models to work with less invasive and more convenient wrist-based sensors requires adapting the biomarker detection models to work with wrist-worn sensor data. To that end, we develop robust stress and activity inference methodologies from noisy wrist-sensor data. We first propose CQP, which quantifies wrist-sensor collected PPG data quality. Next, we show that integrating CQP within the inference pipeline improves accuracy-yield trade-offs associated with stress detection from wrist-worn PPG sensors in the natural environment. mRisk also requires sensor-based precise detection of smoking events and confirmation through self-reports to extract positive labels. Hence, we develop rSmoke, an orientation-invariant smoking detection model that is robust to the variations in sensor data resulting from orientation switches in the field. We train the proposed mRisk risk estimation models using the wrist-based inferences of lapse risk factors. To evaluate the utility of the risk models, we simulate the delivery of intelligent smoking interventions to at-risk participants as informed by the composite risk scores. Our results demonstrate the envisaged impact of machine learning-based models operating on wrist-worn wearable sensor data to output continuous smoking lapse risk scores. The novel methodologies we propose throughout this dissertation help instigate a new frontier in smoking research that can potentially improve the smoking abstinence rate in participants willing to quit.
Library Comment
Dissertation or thesis originally submitted to ProQuest
Notes
Open Access
Recommended Citation
Ullah, Md Azim, "Continuous Estimation of Smoking Lapse Risk from Noisy Wrist Sensor Data Using Sparse and Positive-Only Labels" (2023). Electronic Theses and Dissertations. 3083.
https://digitalcommons.memphis.edu/etd/3083
Comments
Data is provided by the student.