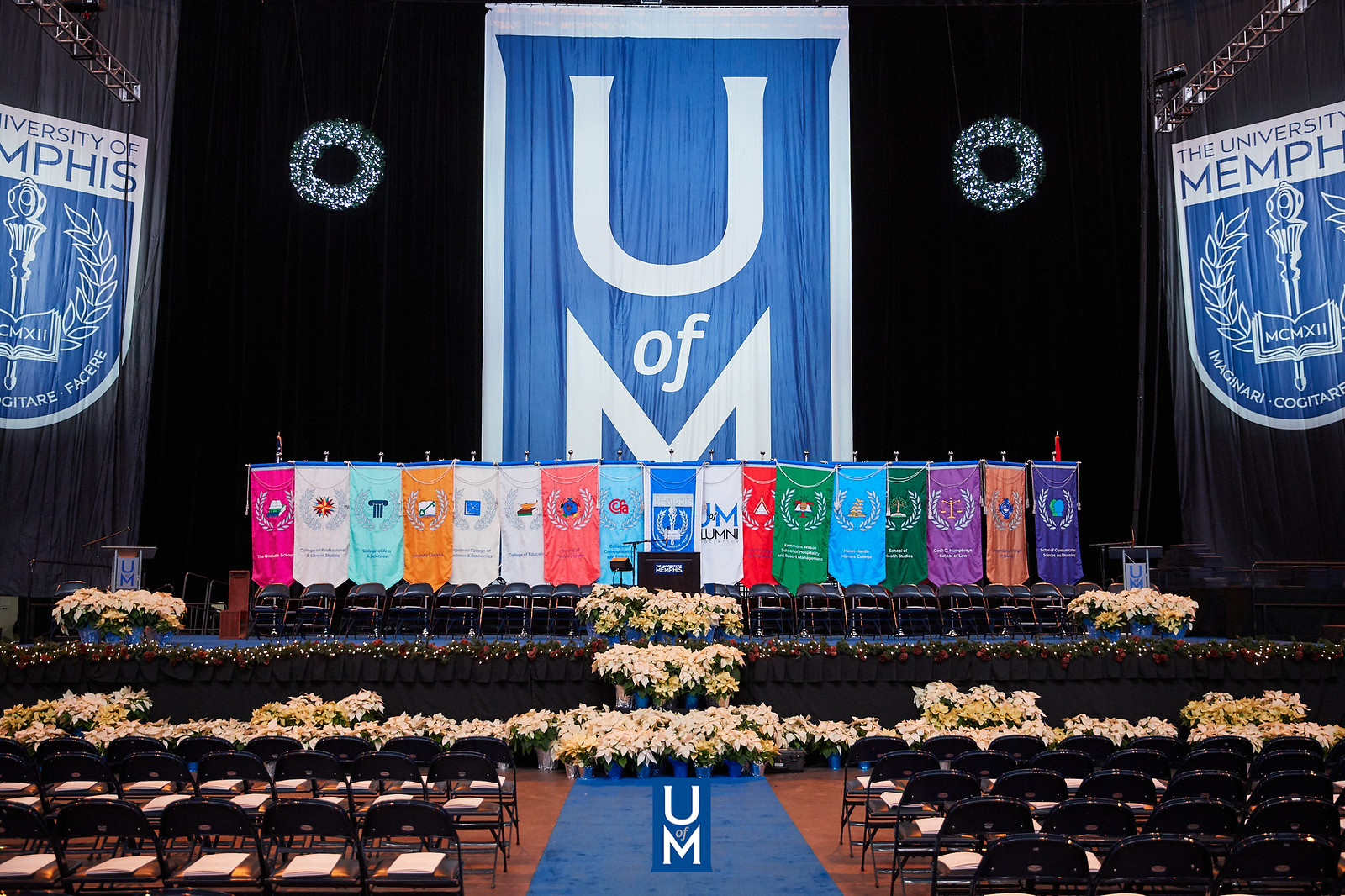
Electronic Theses and Dissertations
Date
2023
Document Type
Dissertation
Degree Name
Doctor of Philosophy
Department
Civil Engineering
Committee Chair
Sabyasachee Mishra
Committee Member
Mihalis Golias
Committee Member
Charles Camp
Committee Member
Weizi Li
Abstract
The world is urbanizing at an unprecedented rate where urbanization goes from 39% in 1980 to 58% in 2019 (World Bank, 2019). This poses more and more transportation demand and pressure on the already at or over-capacity old transport infrastructure, especially in urban areas. Along the same timeline, more data generated as a byproduct of daily activity are being collected via the advancement of the internet of things, and computers are getting more and more powerful. These are shown by the statistics such as 90% of the world’s data is generated within the last two years and IBM’s computer is now processing at the speed of 120,000 GPS points per second. Thus, this dissertation discusses the challenges and opportunities arising from the growing demand for urban mobility, particularly in cities with outdated infrastructure, and how to capitalize on the unprecedented growth in data in solving these problems by ways of data-driven transportation-specific methodologies. The dissertation identifies three primary challenges and/or opportunities, which are (1) optimally locating dynamic wireless charging to promote the adoption of electric vehicles, (2) predicting dynamic traffic state using an enormously large dataset of taxi trips, and (3) improving the ride-hailing system with carpooling, smart dispatching, and preemptive repositioning. The dissertation presents potential solutions/methodologies that have become available only recently thanks to the extraordinary growth of data and computers with explosive power, and these methodologies are (1) bi-level optimization planning frameworks for locating dynamic wireless charging facilities, (2) Traffic Graph Convolutional Network for dynamic urban traffic state estimation, and (3) Graph Matching and Reinforcement Learning for the operation and management of mixed autonomous electric taxi fleets. These methodologies are then carefully calibrated, methodically scrutinized under various performance metrics and procedures, and validated with previous research and ground truth data, which is gathered directly from the real world. In order to bridge the gap between scientific discoveries and practical applications, the three methodologies are applied to the case study of (1) Montgomery County, MD, (2) the City of New York, and (3) the City of Chicago and from which, real-world implementation are suggested. This dissertation’s contribution via the provided methodologies, along with the continual increase in data, have the potential to significantly benefit urban mobility and work toward a sustainable transportation system.
Library Comment
Dissertation or thesis originally submitted to ProQuest
Notes
Open Access
Recommended Citation
Ngo, Huan H., "Data-driven Methodologies and Applications in Urban Mobility" (2023). Electronic Theses and Dissertations. 3133.
https://digitalcommons.memphis.edu/etd/3133
Comments
Data is provided by the student.