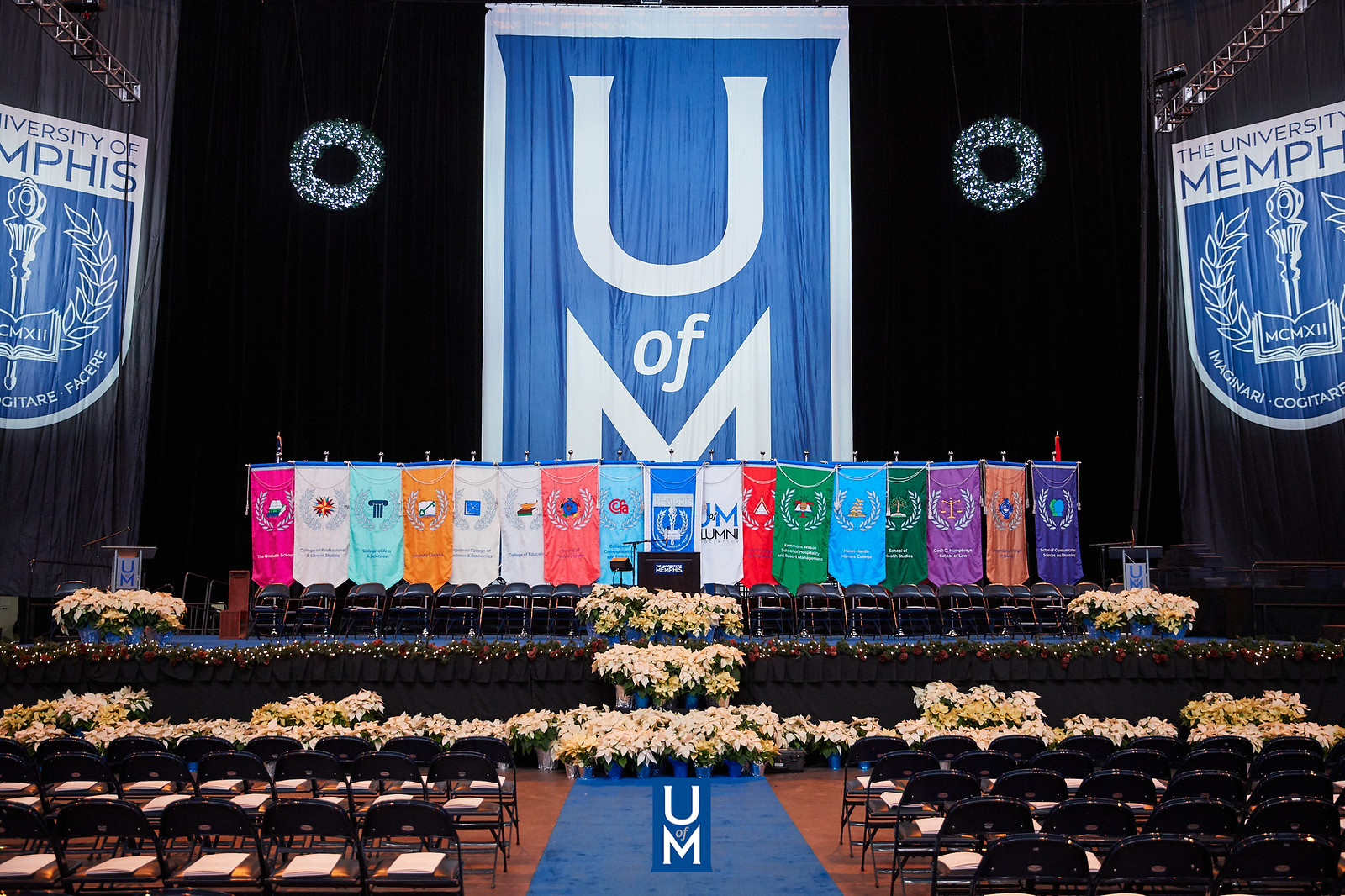
Electronic Theses and Dissertations
Date
2022
Document Type
Dissertation
Degree Name
Doctor of Philosophy
Department
Chemistry
Committee Chair
Abby Parrill
Committee Member
Daniel Baker
Committee Member
Judith A Cole
Committee Member
Nathan J DeYonker
Committee Member
Bernie J Daigle
Abstract
G protein-coupled receptors (GPCR) are integral membrane proteins mediating responses from extracellular effectors that regulate a diverse set of physiological functions. Consequently, GPCR are the targets of ~34% of current FDA-approved drugs.3 Although it is clear that GPCR are therapeutically significant, discovery of novel drugs for these receptors is often impeded by a lack of known ligands and/or experimentally determined structures for potential drug targets. However, computational techniques have provided paths to overcome these obstacles. As such, this work discusses the development and application of novel computational methods and workflows for GPCR ligand discovery. Chapter 1 provides an overview of current obstacles faced in GPCR ligand discovery and defines ligand- and structure-based computational methods of overcoming these obstacles. Furthermore, chapter 1 outlines methods of hit list generation and refinement and provides a GPCR ligand discovery workflow incorporating computational techniques. In chapter 2, a workflow for modeling GPCR structure incorporating template selection via local sequence similarity and refinement of the structurally variable extracellular loop 2 (ECL2) region is benchmarked. Overall, findings in chapter 2 support the use of local template homology modeling in combination with de novo ECL2 modeling in the presence of a ligand from the template crystal structure to generate GPCR models intended to study ligand binding interactions. Chapter 3 details a method of generating structure-based pharmacophore models via the random selection of functional group fragments placed with Multiple Copy Simultaneous Search (MCSS) that is benchmarked in the context of 8 GPCR targets. When pharmacophore model performance was assessed with enrichment factor (EF) and goodness-of-hit (GH) scoring metrics, pharmacophore models possessing the theoretical maximum EF value were produced in both resolved structures (8 of 8 cases) and homology models (7 of 8 cases). Lastly, chapter 4 details a method of structure-based pharmacophore model generation using MCSS that is applicable to targets with no known ligands. Additionally, a method of pharmacophore model selection via machine learning is discussed. Overall, the work in chapter 4 led to the development of pharmacophore models exhibiting high EF values that were able to be accurately selected with machine learning classifiers.
Library Comment
Dissertation or thesis originally submitted to ProQuest
Notes
Open Access
Recommended Citation
Szwabowski, Gregory Leon, "Benchmarking and Developing Novel Methods for G Protein-coupled Receptor Ligand Discovery" (2022). Electronic Theses and Dissertations. 3188.
https://digitalcommons.memphis.edu/etd/3188
Comments
Data is provided by the student.