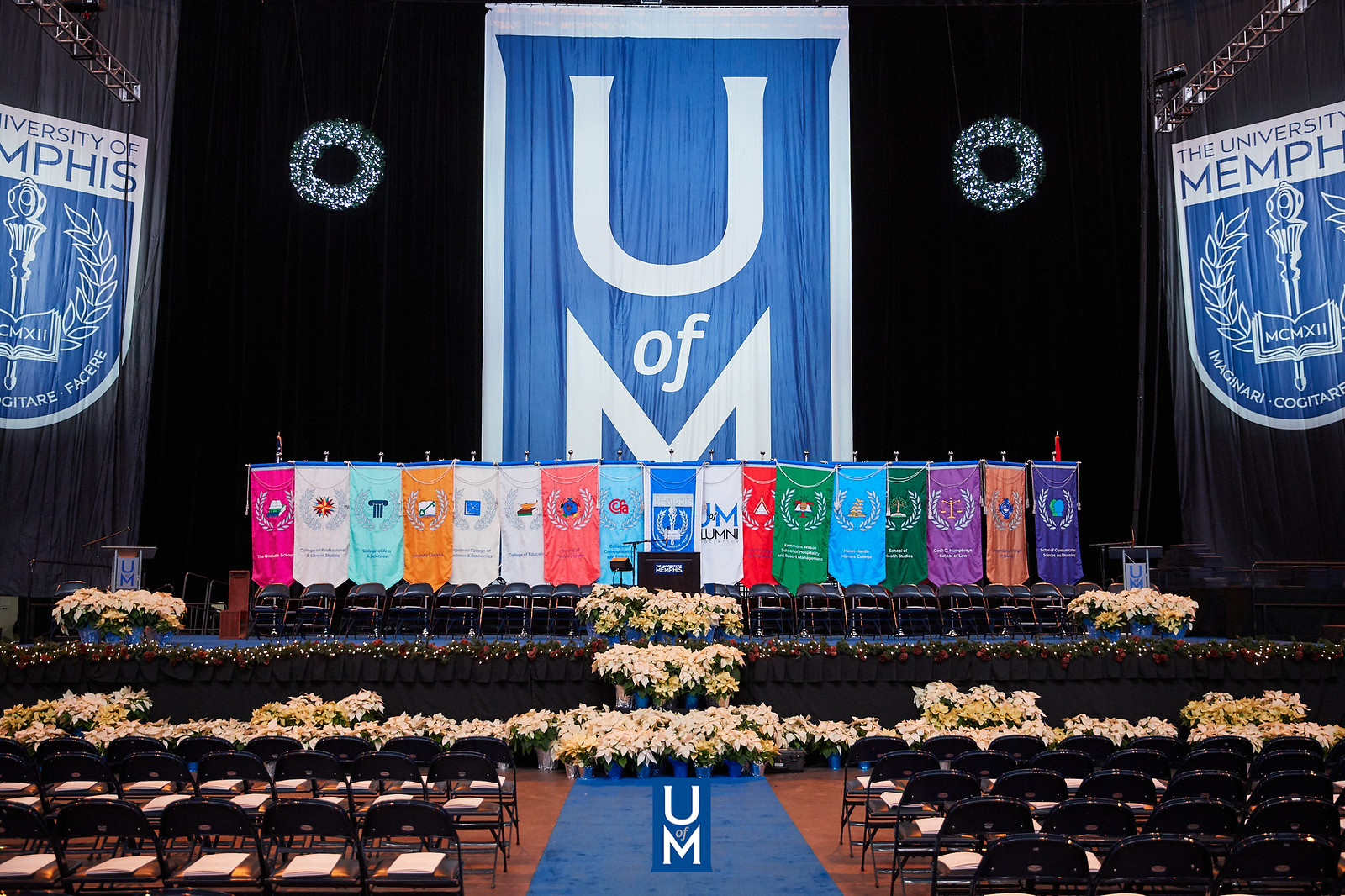
Electronic Theses and Dissertations
Date
2022
Document Type
Dissertation
Degree Name
Doctor of Philosophy
Department
Earth Sciences
Committee Chair
Youngsang Kwon
Committee Member
Mervin MB Bartholomew
Committee Member
Dorian DB Burnett
Committee Member
Deepak DV Venugopal
Committee Member
Peter PK Kedron
Abstract
Biodiversity is in decline and predicting species diversity is critically important if current trends are to be reversed. Tree species richness (TSR), the number of distinct tree species in an ecological community, has long been a key measure of biodiversity, however, accurately modeling TSR is critical to better predicting its spatially heterogeneous responses to the changing environment and to forecasting TSR under future climate scenarios. This dissertation takes a holistic approach to improve TSR modeling with 1) advanced and more interpretable machine learning techniques, 2) to extend predictors to anthropogenic factors, and 3) to leverage open-source online tree occurrences for TSR estimates in the continental United States. The machine learning (ML) approaches demonstrated the efficacy of artificial neural networks (ANN) and random forest (RF) to predict the spatial patterns of TSR examined by improved accuracy and less spatially clustered TSR residuals. In addition, the Interpretable Machine Learning (IML) approach, SHapley Additive exPlanations (SHAP), revealed the important contribution of forest area and several precipitation-related covariates as well as interaction effects among predictors to TSR. It highlighted the significance of conservation efforts of forest areas and importance of understanding precipitation-related stresses for preserving TSR. Landscape metrics as a new predictor group in XGBoost ML model indicated that landscape heterogeneity are relevant covariates for TSR. The most important landscape metrics are concerning shape and size of forest (core) area. Forest communities with large forest (core) areas of simple geometric shapes and connected forest patches are associated with a larger TSR. Lastly, tree occurrence records from the open-source databases of GBIF and BIEN present various quality and spatial coverage for the continental U.S. A series of quality checks were required to ensure the integration of GBIF and BIEN database with FIA. They showed great potential in predicting TSR estimates with increased spatial coverage and inclusiveness of tree species. This dissertation contributes to improve modeling and prediction of tree diversity while probing into the non-linear and complex interactions that exist between the multitudinous variables affecting tree diversity.
Library Comment
Dissertation or thesis originally submitted to ProQuest.
Notes
Open access
Recommended Citation
Brugere, Lian, "PREDICTING TREE SPECIES RICHNESS IN THE CONTINENTAL UNITED STATES: HIGHLIGHTS FROM MACHINE LEARNING AND OPEN DATA" (2022). Electronic Theses and Dissertations. 3404.
https://digitalcommons.memphis.edu/etd/3404
Comments
Data is provided by the student.