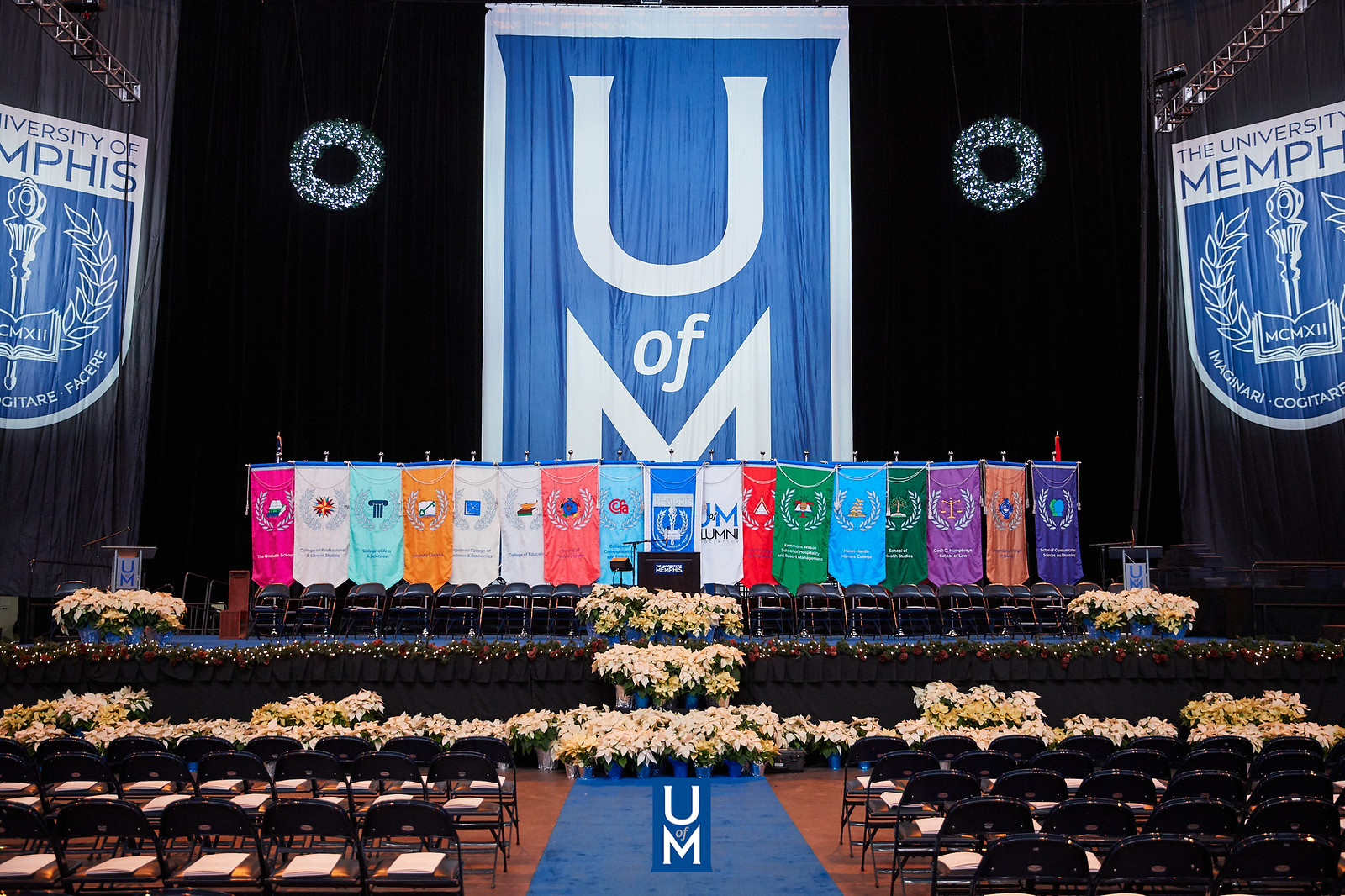
Electronic Theses and Dissertations
Date
2022
Document Type
Thesis
Degree Name
Master of Science
Department
Electrical & Computer Engineering
Committee Chair
Mohd. Hasan Ali
Committee Member
Madhusudhanan Balasubramanian
Committee Member
Sajjan G. Shiva
Committee Member
Bradford D. Pendley
Abstract
Sudden Cardiac Arrest (SCA) is one of the global health issues causing high mortality. Hence, timely and agile detection of such arrests and immediate defibrillation support to the victim is of the utmost importance. An Automated External Defibrillator (AED) is a medical device that provides defibrillation support to patients suffering from SCA. AED is typically used to restore a normal heart rhythm during cardiac arrest. AED implements the machine learning (ML) or deep learning (DL)-based approach to detect whether the patient needs an electric shock or not and then automates the electric shock to the SCA victims through the chest if needed. However, the effectiveness of these models has relied on the availability of large and balanced datasets. Collecting sufficient data is more challenging in the medical domain, mainly due to data privacy. We, therefore, propose a novel External Classifier - Wasserstein Conditional Generative Adversarial Network (EC-WCGAN) to detect the shockable rhythms in AED on an imbalanced ECG dataset. The EC-WCGAN comprises a deep neural network (DNN) as an external classifier to classify heart rhythms into shockable or non-shockable rhythms and WCGAN with gradient penalty as a synthetic tabular data generator for the low-class to overcome the class imbalance problem during the training. Our experiments demonstrate that the classifier trained with real and generated data via the EC-WCGAN provides significant classification performance on the imbalanced dataset. Also, the GAN-based sampling method for generating low sample data is far better than standard sampling techniques. In addition, our proposed model is superior to other existing models in this field in terms of high sensitivity and specificity (more than 99%) and low balanced error rate (0.005) on the 4-second segmented public holter databases, meeting the America Health Association criteria for AED. Overall, the AED built on the EC-WCGAN model can detect shockable rhythms with more than 99% detection accuracy, making it safe and effective for SCA victims.
Library Comment
Dissertation or thesis originally submitted to ProQuest.
Notes
Open access
Recommended Citation
Dahal, Kamana, "AUTOMATIC DETECTION OF SHOCKABLE RHYTHMS IN AED FROM IMBALANCED ECG DATASET USING EC-WCGAN" (2022). Electronic Theses and Dissertations. 3437.
https://digitalcommons.memphis.edu/etd/3437
Comments
Data is provided by the student.