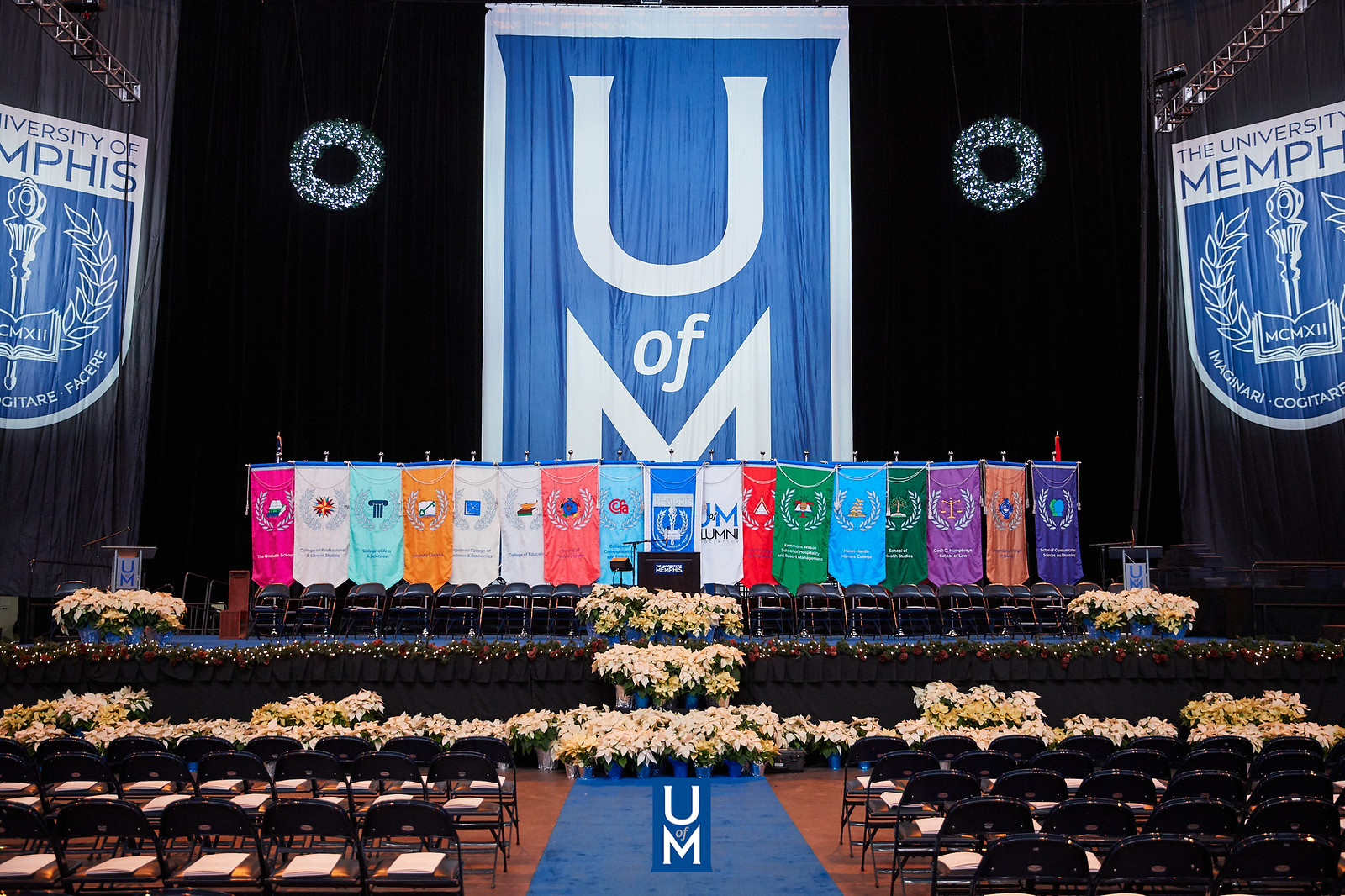
Electronic Theses and Dissertations
Identifier
5
Date
2010
Document Type
Dissertation
Degree Name
Doctor of Philosophy
Major
Computer Science
Committee Chair
Sajjan Shiva
Committee Member
Vasile Rus
Committee Member
Qishi Wu
Committee Member
Ebenezer George
Abstract
Customer churn can be described as the process by which consumers of goods and services discontinue the consumption of a product or service and switch over to a competitor.It is of great concern to many companies. Thus, decision support systems are needed to overcome this pressing issue and ensure good return on investments for organizations. Decision support systems use analytical models to provide the needed intelligence to analyze an integrated customer record database to predict customers that will churn and offer recommendations that will prevent them from churning. Customers churn prediction, unlike most conventional business intelligence techniques, deals with customer demographics, net worth-value, and market opportunities. It is used in determining customers who are likely to churn, those likely to remain loyal to the organization, and for prediction of future churn rates. Customer defection is naturally a slow rate event, and it is not easily detected by most business intelligent solutions available in the market; especially when data is skewed, large, and distinct. Thus, accurate and precise prediction methods are needed to detect the churning trend. In this study, a churn model that applies business intelligence techniques to detect the possibility that a customer will churn using churn trend analysis of customer records is proposed. The model applies clustering algorithms and enhanced SPRINT decision tree algorithms to explore customer record database, and identify the customer profile and behavior patterns. The Model then predicts the possibility that a customer will churn. Additionally, it offers solutions for retaining customers and making them loyal to a business entity by recommending customer-relationship management measures.
Library Comment
Dissertation or thesis originally submitted to the local University of Memphis Electronic Theses & dissertation (ETD) Repository.
Recommended Citation
Anyanwu, Matthew N., "ENHANCEMENT OF CHURN PREDICTION ALGORITHMS" (2010). Electronic Theses and Dissertations. 4.
https://digitalcommons.memphis.edu/etd/4
Comments
Data is provided by the student.