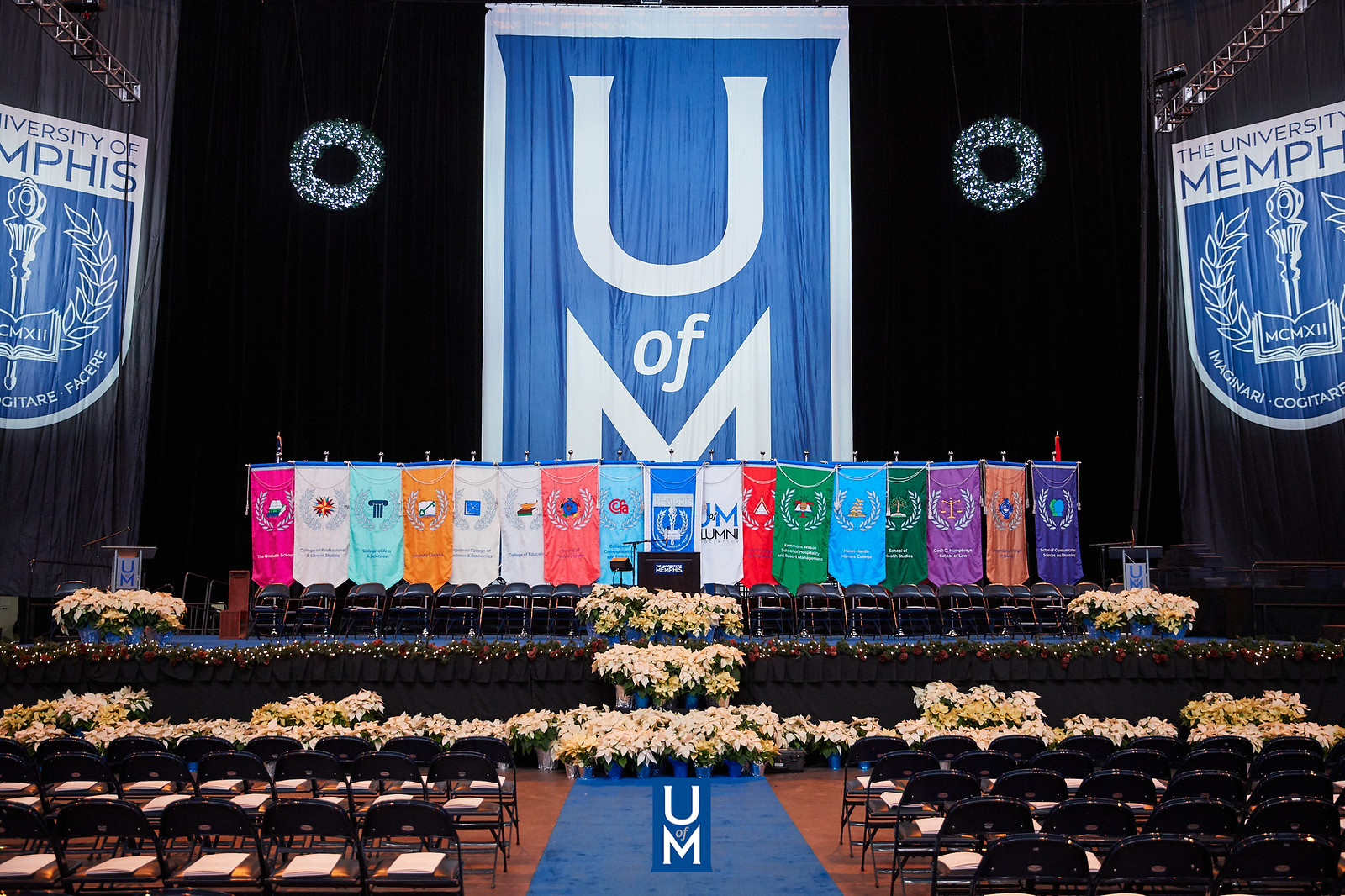
Electronic Theses and Dissertations
Identifier
1146
Date
2014
Document Type
Thesis
Degree Name
Master of Science
Major
Computer Science
Committee Chair
Santosh Kumar
Committee Member
King-Ip Lin
Committee Member
Vesile Rus
Abstract
Automated detection of social interactions in the natural environment has resulted in promising advances in organizational behavior, consumer behavior, and behavioral health. Progress, however, has been limited since the primary means of assessing social interactions today (i.e., audio recording) has several issue in field usage such as microphone occlusion, lack of speaker specificity, and high energy drain, in addition to significant privacy concerns.In this thesis, we present a new mobile-based system mConverse to infer conversation episodes from respiration measurements collected in field from an unobtrusively wearable Respiratory Inductive Plethysmograph (RIP) band worn around the user's chest. The measurements are wirelessly transmitted to a mobile phone, where they are used in a machine learning model to determine whetehr the wearer is speaking, listening, or quiet. This model incorporates several innovations to address issues that naturally arise in the noisy field environment such as confounding events, poor data quality due to sensor loosening and detachment, lossess in the wireless channel, etc. Our basic model obtains 83% accuracy for the three class classification. We formulate a Hidden Markov Model (HMM) to further improve the accuracy to 87%. Finally, we apply our model to data collected from 22 subjects who wore the sensor for 2 full days in the field to observe conversation behavior in daily life and find that people spend 25% of their day in conversation.
Library Comment
Dissertation or thesis originally submitted to the local University of Memphis Electronic Theses & dissertation (ETD) Repository.
Recommended Citation
Rahman, Md Mahbubur, "Inferring Conversation Episodes From Respiratory Measurements" (2014). Electronic Theses and Dissertations. 964.
https://digitalcommons.memphis.edu/etd/964
Comments
Data is provided by the student.