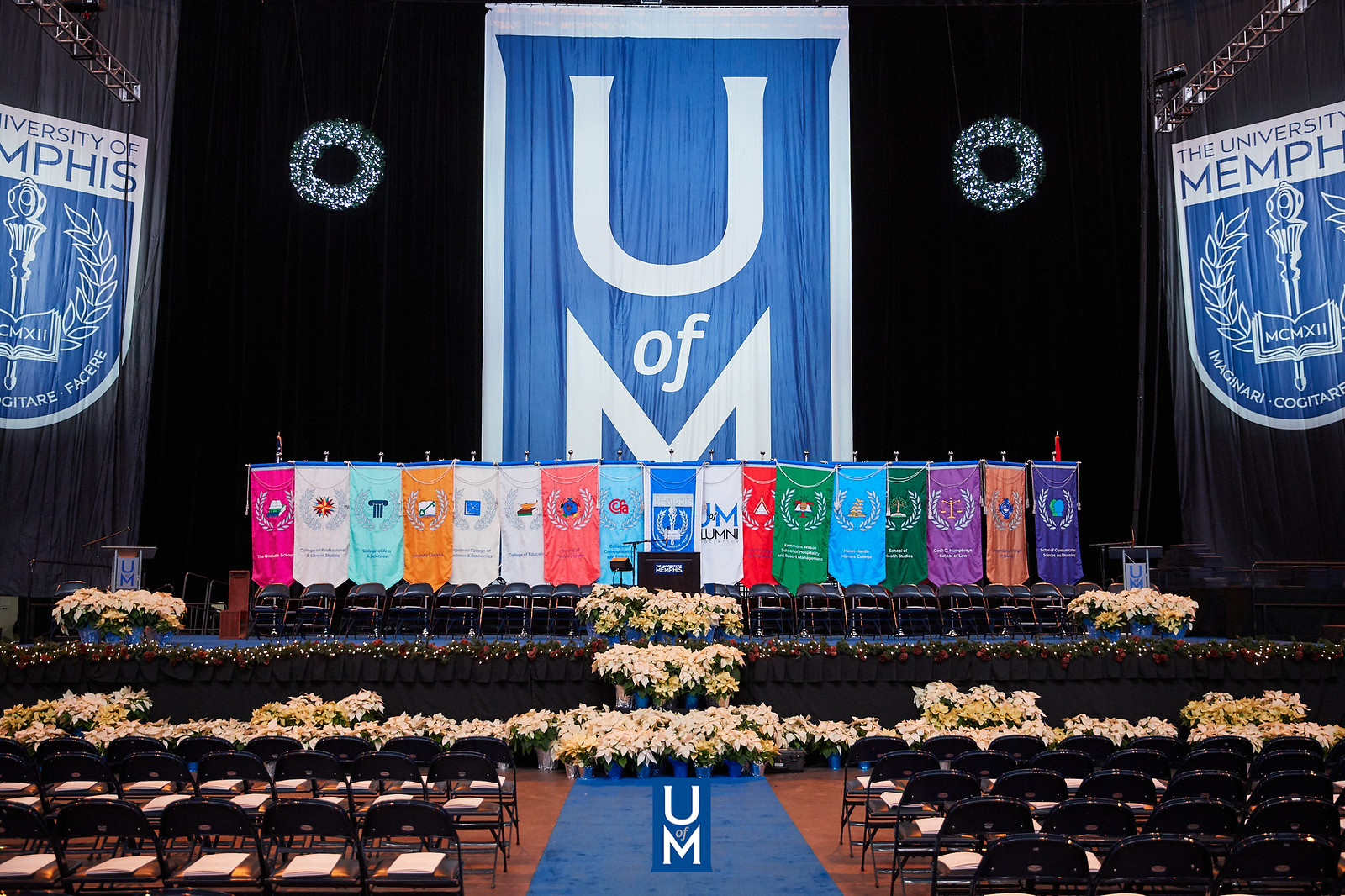
Electronic Theses and Dissertations
Identifier
1290
Date
2014
Document Type
Dissertation
Degree Name
Doctor of Philosophy
Major
Computer Science
Committee Chair
Santosh Kumar
Committee Member
Vasile Rus
Committee Member
Scott Fleming
Committee Member
Benjamin Marlin
Abstract
Smoking has been conclusively proved to be the leading cause of preventable deaths in the United States. Extensive research is conducted on developing effective smoking cessation programs. Most smoking cessation programs achieve low success rate because they are unable to intervene at the right moment. Identification of high-risk situations that may lead an abstinent smoker to relapse involves discovering the associations among various contexts that precede a smoking session or a smoking lapse. In the absence of an automated method, detection of smoking events still relies on subject self-report that is prone to failure to report and involves subject burden. Automated detection of smoking events in the natural environment can revolutionize smoking research and lead to effective intervention. We investigate the feasibility of automated detection smoking puff from measurement obtained from respiratory inductive plethysmography (RIP) sensor. We introduce several new features from respiration that can help classify individual respiration cycles into smoking puffs or non-puffs. We then propose supervised and semi-supervised support vector models to detect smoking puffs. We train our models on data collected from 10 daily smokers and show that our model can still identify smoking puffs with an accuracy of 86.7%. We further show accuracy of smoking puff detection can be improved by fusing measurements from RIP and inertial sensors. We use measurements obtained from wrist worn accelerometer and gyroscope to find segments when the hand is at mouth. The segments are used to identify respiration cycles that can be potentially puff cycles. A SVM classifier is trained using 40 hours of data collected from 6 participants. The 10-fold cross validation results show that at 90.3% true positive rate, respiration feature based classifier produces on average 43.8 false positives puff per hours which is reduced to 3.7 false positives per hour when both wrist and respiration features are used. We also perform leave one subject out cross validation and show that the method generalized well.
Library Comment
Dissertation or thesis originally submitted to the local University of Memphis Electronic Theses & dissertation (ETD) Repository.
Recommended Citation
Ali, Amin Ahsan, "Automated Detection of Cigarette Smoking Puffs from Mobile Sensors - A Multimodal Approach" (2014). Electronic Theses and Dissertations. 1085.
https://digitalcommons.memphis.edu/etd/1085
Comments
Data is provided by the student.