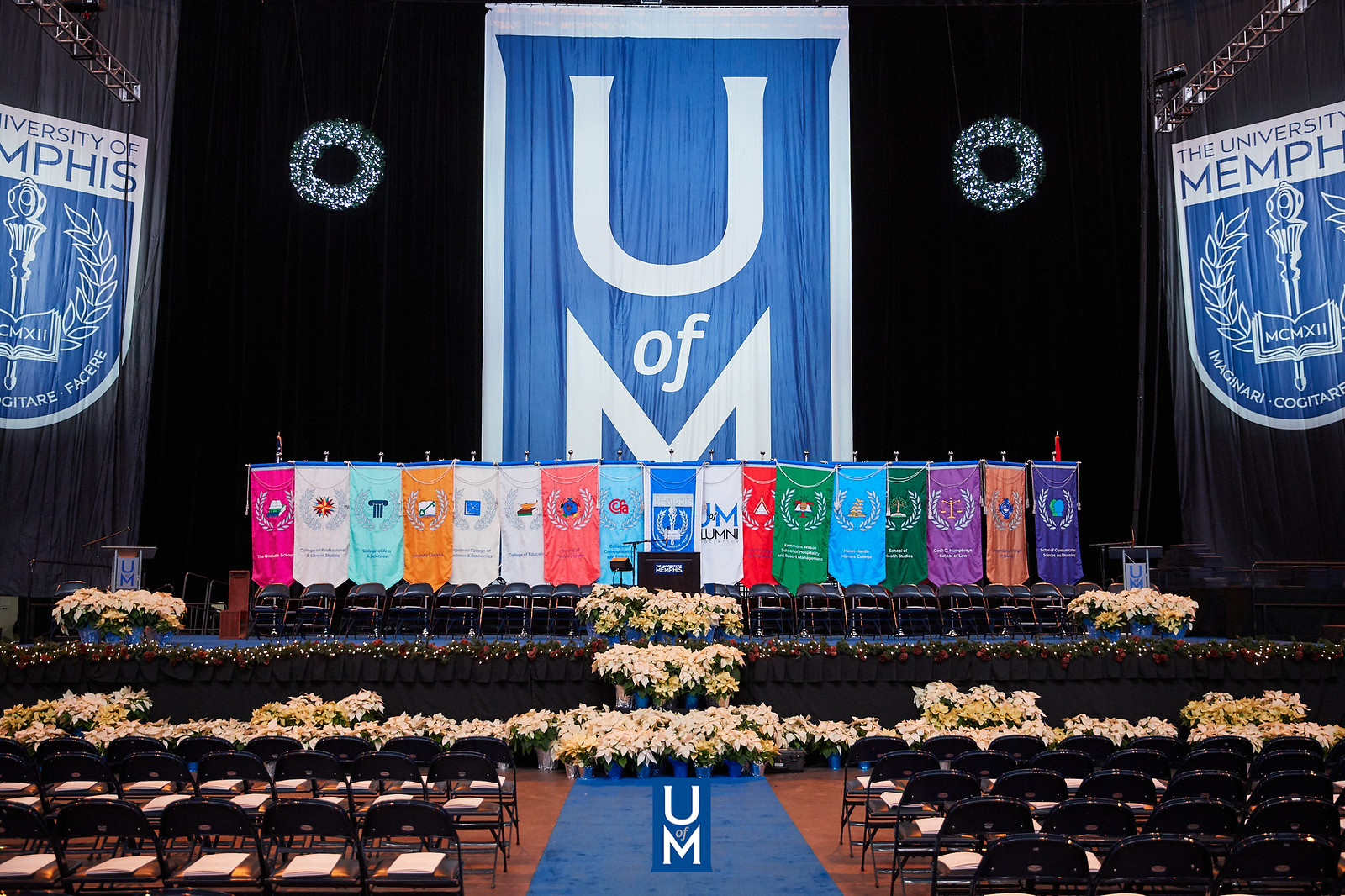
Electronic Theses and Dissertations
Identifier
2589
Date
2016
Document Type
Dissertation
Degree Name
Doctor of Philosophy
Major
Electrical and Computer Engr
Concentration
Computer Engineering
Committee Chair
Bashir Morshed
Committee Member
Russell Deaton
Committee Member
Eddie Jacobs
Committee Member
Gavin M Bidelman
Abstract
Body sensor network (BSN) is a promising technology to monitor neurophysiological data in naturalistic settings. Existing BSNs with wearable Electroencephalogram (EEG) to record neurological activities have limitations in terms of modularity, scalability, and flexibility in deployment. Also, EEG signals are often contaminated with ocular artifacts (OA) like eye-blinks that must be cleaned prior to the signal analysis. The current OA denoising techniques require human supervision that limits automation. The key objectives of this research were: (1) to design an unsupervised and fully-automatic algorithm for denoising eye-blinks in multi-channel EEG, (2) to investigate EEG modularization by eliminating the requirement of driven-right-leg (DRL) circuit for the battery-operated EEG and investigation of consequential effects, and (3) to develop a scalable and reconfigurable architecture for BSN with modular EEG nodes that can be deployed in a Lego-like fashion.A robust algorithm to denoise eye-blink artifacts was used which uses modified multiscale sample entropy and kurtosis to automatically identify the independent eye-blink artifactual components and subsequently denoise these components using wavelet decomposition. To evaluate the DRL effects, a single-channel battery-powered EEG with a new analog front end (AFE) was designed that can record neural signals with and without DRL. Furthermore, sensor-level modular EEG nodes with in situ AFE are prototyped and integrated into the network via digital (I2C) interface. These prototypes were validated against two commercially available EEG.The reconstructed signals with the proposed unsupervised algorithm achieve improved performance of mutual information, correlation coefficient, and spectral coherence compared to the conventional ICA and wavelet-ICA denoising techniques. The prototyped EEG with a novel analog front end, with and without DRL, offers a comparable common-mode rejection ratio of 72.98 dB and 71.74 dB, respectively, at 60 Hz. This led us to develop an architecture that can configure up to 39 EEG nodes at the time of deployment in a Lego-like fashion. With the automated eye-blink removal of EEG signals and eliminated hardware limitations of DRL, the envisioned body-worn reconfigurable architecture of integrated network sensors (BRAINsens), a fully-reconfigurable BSN with modular EEG, is promising for use in non-clinical settings. Such a BSN can play important roles for monitoring patients with neurological disorders and in emergency care where the locations of EEG electrodes need to suit the individual’s need.
Library Comment
Dissertation or thesis originally submitted to the local University of Memphis Electronic Theses & dissertation (ETD) Repository.
Recommended Citation
Mahajan, Ruhi, "BRAINsens: Body-worn Reconfigurable Architecture of Integrated Network Sensors" (2016). Electronic Theses and Dissertations. 1337.
https://digitalcommons.memphis.edu/etd/1337
Comments
Data is provided by the student.