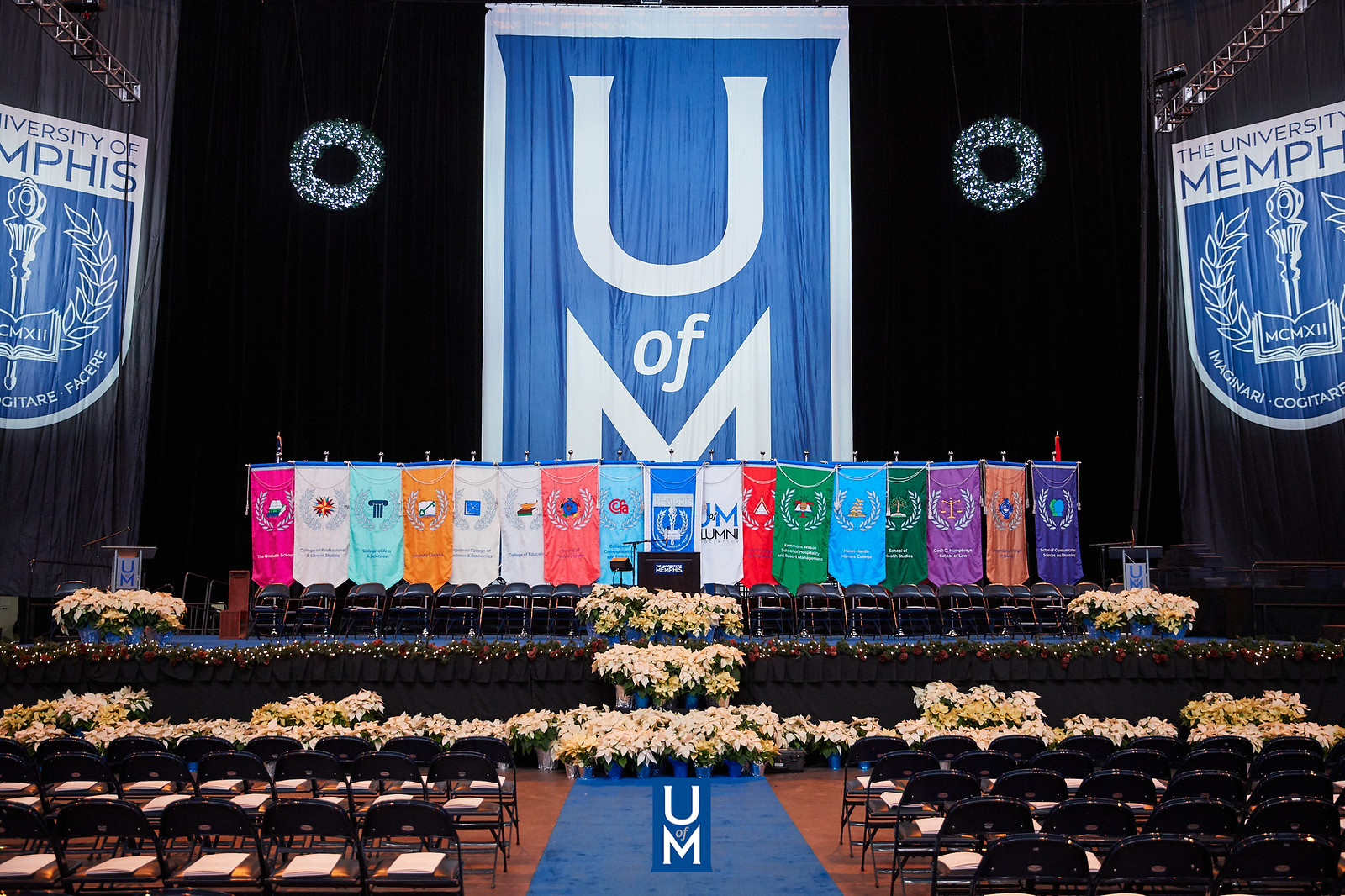
Electronic Theses and Dissertations
Identifier
4811
Date
2016
Document Type
Dissertation
Degree Name
Doctor of Philosophy
Major
Mathematical Sciences
Concentration
Applied Statistics
Committee Chair
Ebenezer Olusegun George
Committee Member
Su Chen
Committee Member
Albert Okunade
Abstract
The development of exact, non-asymptotic, procedures for analyzing clustereddiscrete data has remained a challenging problem for research statisticians due tothe dearth of tractable probability models for describing complex dependencestructures of discrete data points within clusters. Under an assumption of deFinetti's definition of infinite exchangeability, several models have beendeveloped. However, these procedures are not valid when data comes fromintrinsically finite exchangeable population. Moreover, the procedures invariablyconduct inference based on asymptotic distribution of estimators or test statistics.For example, for testing association between the rows and columns of a contingencytable the asymptotic distribution of the likelihood ratio statistic under nullhypothesis of independence is widely used in many applications. Such asymptoticdistributions are known to be inaccurate when sample size is moderate or when dataare unbalanced or sparse. The goal of this dissertation is to develop andimplement exact conditional nonparametric testing procedures for sparse,unbalanced correlated clustered binary data under an assumption of finiteexchangeability. The methods proposed in this thesis takes advantage of theincreasing availability of computational power and the development of efficientprocedures for enumerating tables, such as the network algorithm andPatefield's algorithm . These methods have facilitated the computation of exactp-value in many cases. We show that for fixed cluster sizes, the distribution ofmarginal sums of responses can be modeled as a multinomial distribution and thatthese marginal sums are jointly sufficient statistics . We construct an exact testby conditioning on the sufficient statistics of the multinomial distribution, therebyeliminating all nuisance parameters. By conditioning on the cluster sizes, we develop an exact nonparametric test for linear a trend. We illustrate theperformance of the tests proposed in comparison to those based on unconditionaland asymptotic methods by using Monte-Carlo and other stochastic simulations.Our research demonstrates that the exact tests that we have developed can havesignificantly different p-values, and in many cases superior power, when comparedto those using large sample theory approximation when data are sparse or clustersizes are unbalanced.
Library Comment
Dissertation or thesis originally submitted to the local University of Memphis Electronic Theses & dissertation (ETD) Repository.
Recommended Citation
Boateng, Nana Akwasi Abayie, "On Some Exact Nonparametric Conditional Test for Clustered Binary Data" (2016). Electronic Theses and Dissertations. 1534.
https://digitalcommons.memphis.edu/etd/1534
Comments
Data is provided by the student.