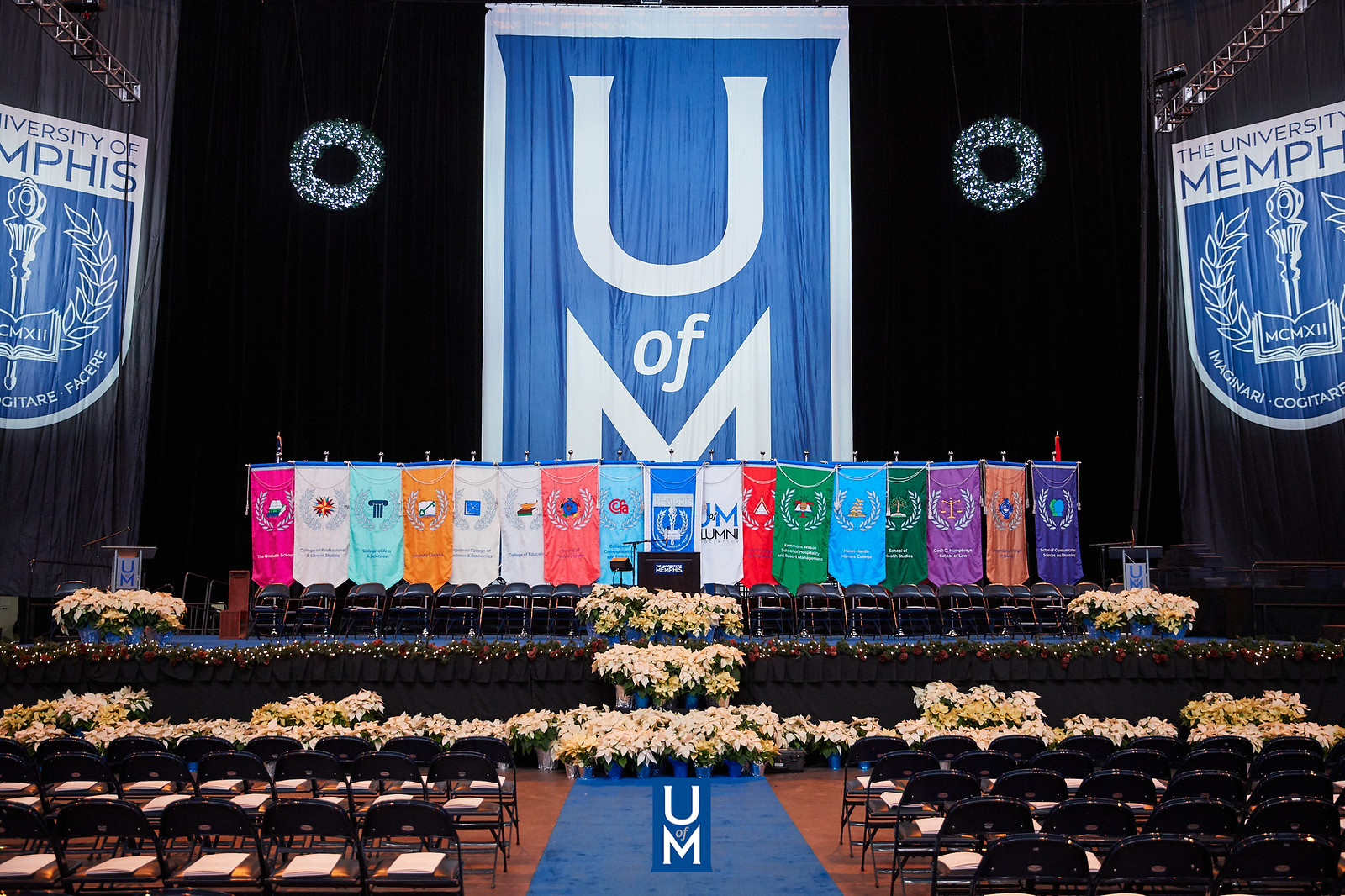
Electronic Theses and Dissertations
Identifier
4892
Date
2017
Document Type
Dissertation
Degree Name
Doctor of Education
Major
Leadership and Policy Studies
Concentration
Educational Leadership
Committee Chair
Charisse Atibagos Gulosino
Committee Member
Reginald Leon Green
Committee Member
Wendy Griswold
Abstract
This research investigated the effect of political, economic, racial, academic, geographic data, and the components of the Race to the Top grant against the Race to the Top grant awards. Data for all 50 states and the District of Columbia was compiled from the federal Race to the Top documents, the Georgetown University Stateminder dataset, and the U.S. Census Bureau to create a combined dataset. The combined dataset included a combination of the 2009 scores from Delaware and Tennessee and the 2010 scores of all other states. Since the scores from 2009 only resulted in two winners, and the 2010 scores resulted in seventeen winners, the 2010 data became the basis for the dataset while including the 2009 data for the two first-round winners. Predictor data for states that did not apply for Race to the Top also was included in the dataset for an extended analysis of self-exclusion of the non-applying states. The analysis used multiple comparison of means, a standard regression model and the Heckman selection regression model to determine if any of the predictors affected the Race to the Top awards. The comparison of means analyzed the differences in averages for Race to the Top Winners and Applicants. The means and the significance of the means for the predictor values, and the means of the scores of the Race to the Top components were calculated. A comparison of number and percentage of significant predictor occurrence, and the means of the Race to the Top components by geographic location was compiled. The standard regression was calculated using the 38 applying states. Since all states did not apply for Race to the Top, and the standard regression model did not account for these exclusions, a secondary regression model, The Heckman Selection Model, was used in conjunction with the standard regression to determine if the excluded states would influence predictor effect. The results demonstrated that several predictors did have a significant effect on Race to the Top application scores and, ultimately, the winning and losing states. Specifically, political affiliation of the state legislative process, race, and academics were significant.
Library Comment
Dissertation or thesis originally submitted to the local University of Memphis Electronic Theses & dissertation (ETD) Repository.
Recommended Citation
Brewer, Brian Wade, "An Inquiry into the Political, Socio-economic, Geographic, and Educational Factors that Predict Race to the Top Winners and Losers" (2017). Electronic Theses and Dissertations. 1598.
https://digitalcommons.memphis.edu/etd/1598
Comments
Data is provided by the student.