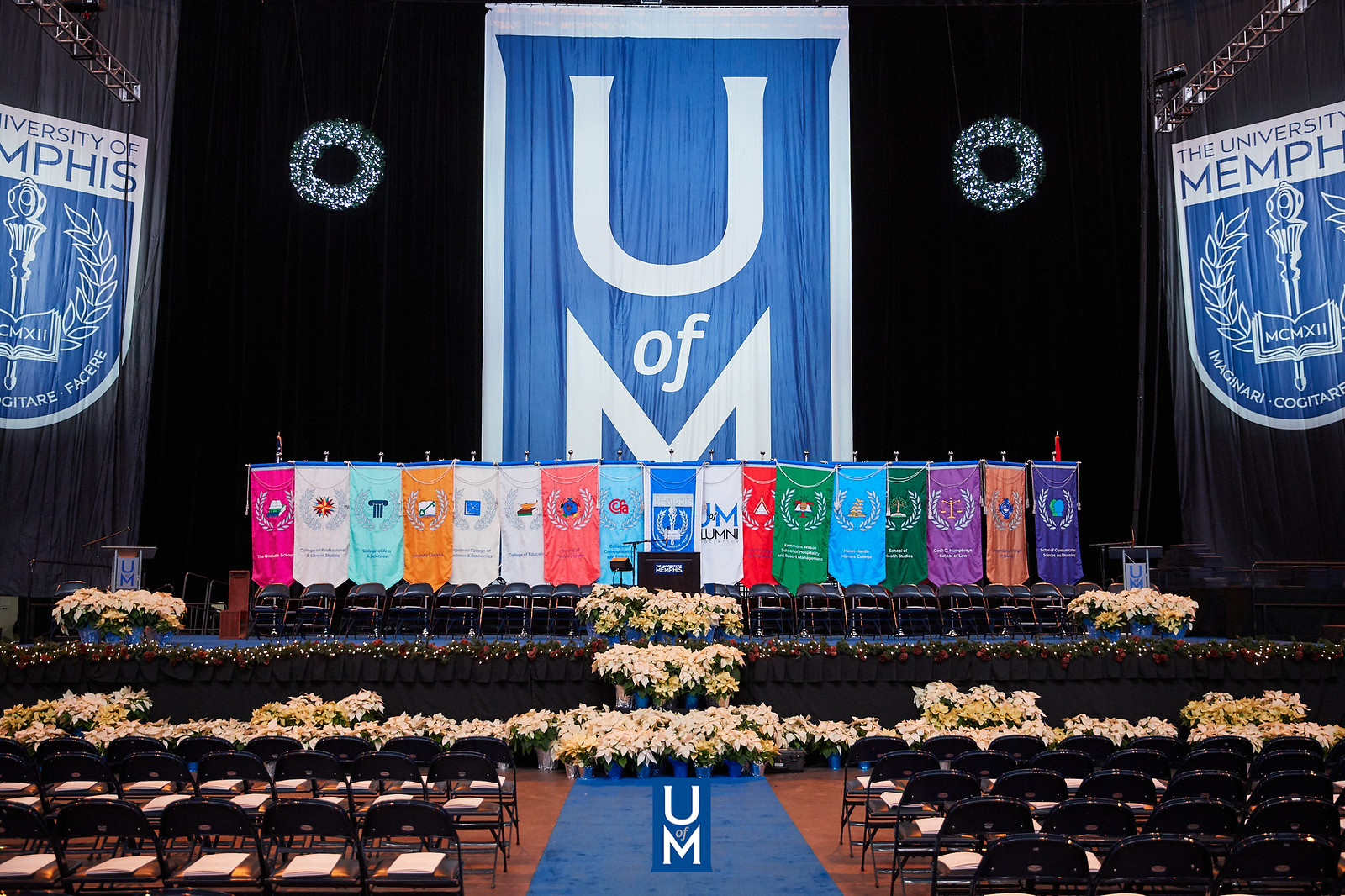
Electronic Theses and Dissertations
Identifier
6001
Date
2017
Document Type
Thesis
Degree Name
Master of Science
Major
Mathematical Sciences
Concentration
Statistics
Committee Chair
Dale Bowman
Committee Member
Saunak Sen
Committee Member
Su Chen
Abstract
Topic modeling is a technique for reducing dimensionality of large corpuses of text. Latent Dirichlet allocation (LDA), the most prevalent form of topic modeling, improved upon earlier methods by introducing Bayesian iterative updates, providing a sound theoretical basis for modeling by iteration. Yet a piece of the modeling puzzle remains unsolved; the number of topics to model, K, is an as yet unanswered question. This number of topics may also be called the dimensionality of the model. With this is an integrally related puzzle; how to determine when a model has been best fit. Presented here are a brief history of the development of topic modeling from its inception preceding LDA to the present; and a comparison of methods for determining what is a best-fit topic model, in pursuit of the most appropriate K.
Library Comment
Dissertation or thesis originally submitted to the local University of Memphis Electronic Theses & dissertation (ETD) Repository.
Recommended Citation
Goedecke, Patricia Jean, "Comparison of Methods for Choosing an Appropriate Number of Topics in an LDA Model" (2017). Electronic Theses and Dissertations. 1695.
https://digitalcommons.memphis.edu/etd/1695
Comments
Data is provided by the student.