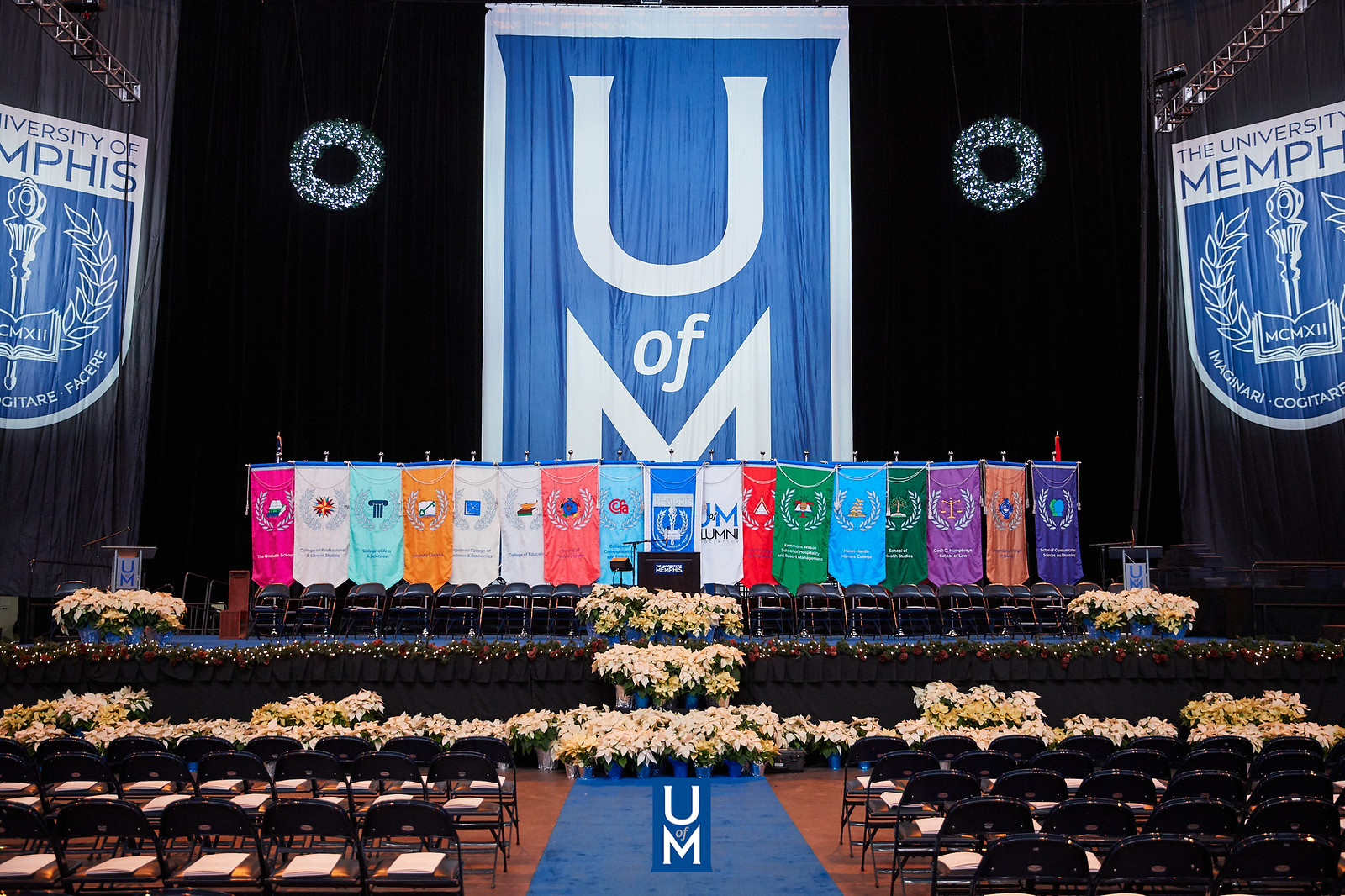
Electronic Theses and Dissertations
Identifier
6236
Date
2018
Document Type
Thesis
Degree Name
Master of Science
Major
Mathematical Sciences
Concentration
Statistics
Committee Chair
Meredith Ray
Committee Member
Dale Bowman
Committee Member
Su Chen
Abstract
This paper delves into the similarities and differences between two methods of exploratory cluster analysis, K-means and Vector in Partition. Known as the traditional clustering approach, K-means does have some limitations when dealing with clustering complex datasets, specifically datasets with variables of multidimensional vectors. This is the gap the Vector in Partition (VIP) algorithm aims to fill. As a novel approach for clustering multidimensional datasets of both continuous and categorical data, the VIP algorithm has preliminary results that support its ability to correctly cluster simulated datasets of the genetic factors, gene expression, DNA methylation, and single nucleotide polymorphisms. After explaining both the K-means algorithm and the VIP algorithm, an example will be presented of simulated genetic data containing variables with multidimensional vectors that will be analyzed with both algorithms. The results will then be summarized using accuracy, sensitivity, and specificity while highlighting the benefits and limitations of each clustering method.
Library Comment
Dissertation or thesis originally submitted to the local University of Memphis Electronic Theses & dissertation (ETD) Repository.
Recommended Citation
Sobral, Lauren, "Comparing and Contrasting Clustering Analysis Methods: K-means and Vector in Partition" (2018). Electronic Theses and Dissertations. 1863.
https://digitalcommons.memphis.edu/etd/1863
Comments
Data is provided by the student.