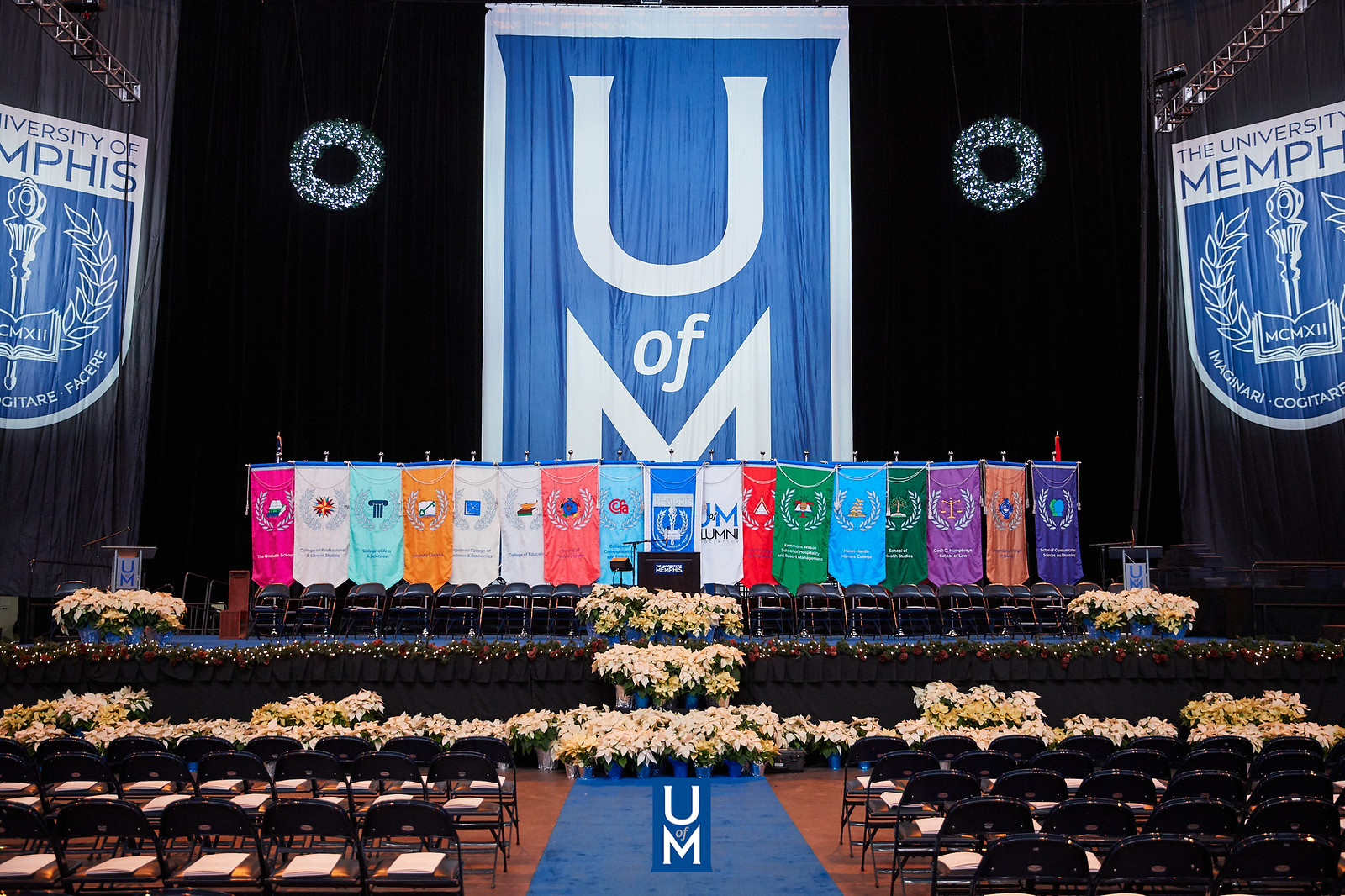
Electronic Theses and Dissertations
Identifier
252
Date
2011
Document Type
Dissertation
Degree Name
Doctor of Philosophy
Major
Mathematical Sciences
Concentration
Mathematics
Committee Chair
Maria Fernanda Botelho
Committee Member
James E. Jamison
Committee Member
John R. Haddock
Committee Member
Robert Kozma
Abstract
Learning is a feature of living organisms which is crucial in the process of adaptation. It is understood that a stimulus triggers a chain of neurophysiological reactions in an organism, and as a consequence we say that the organism is learning from that initial exposure. Several researchers have dealt with understanding and modeling learning through mathematical systems. Due to the complexity of the brain, scholars reduced the problem to a simpler mechanism consisting of neurons, which are processing additive units, interconnected with pathways, called synapses. A primary goal was to derive a system of equations that captures the changes synaptic parameters undergo in a learning process, and identify stimuli that generates a flow of changes that will converge over time. This would represent a stable reflection of the learning process. My dissertation explores generalizations of models for unsupervised learning proposed in literature. The first model is due to Oja and Karhunen and reflects the changes of a network connecting weights or synaptic parameters following a Hebbian principle and incorporating a forgetting term to allow convergence. The second model, due to Cox and Adams, generalizes the Oja-Karhunen model by introducing errors in the learning process. These paradigms are presented as systems of differential equations explored in three settings: 1. The finite dimensional Euclidean space over the reals; 2. Then infinite dimensional Hilbert space of square summable sequences equipped with the standard inner product; and 3. The infinite dimensional Banach space of bounded operators on a separable complex Hilbert space. In each setting the existence and uniqueness of local or global solutions is well established, a form for solutions is derived, and the asymptotic behavior is determined. In the third setting we use the polar factorization of operators to decompose the system into two components where an explicit form for solutions is given. In the Cox-Adams model we also explore the impact of the error factor in the long-term behavior of the solutions.
Library Comment
Dissertation or thesis originally submitted to the local University of Memphis Electronic Theses & dissertation (ETD) Repository.
Recommended Citation
Davis, Annita Thornton, "Models for Unsupervised Learning" (2011). Electronic Theses and Dissertations. 190.
https://digitalcommons.memphis.edu/etd/190
Comments
Data is provided by the student.