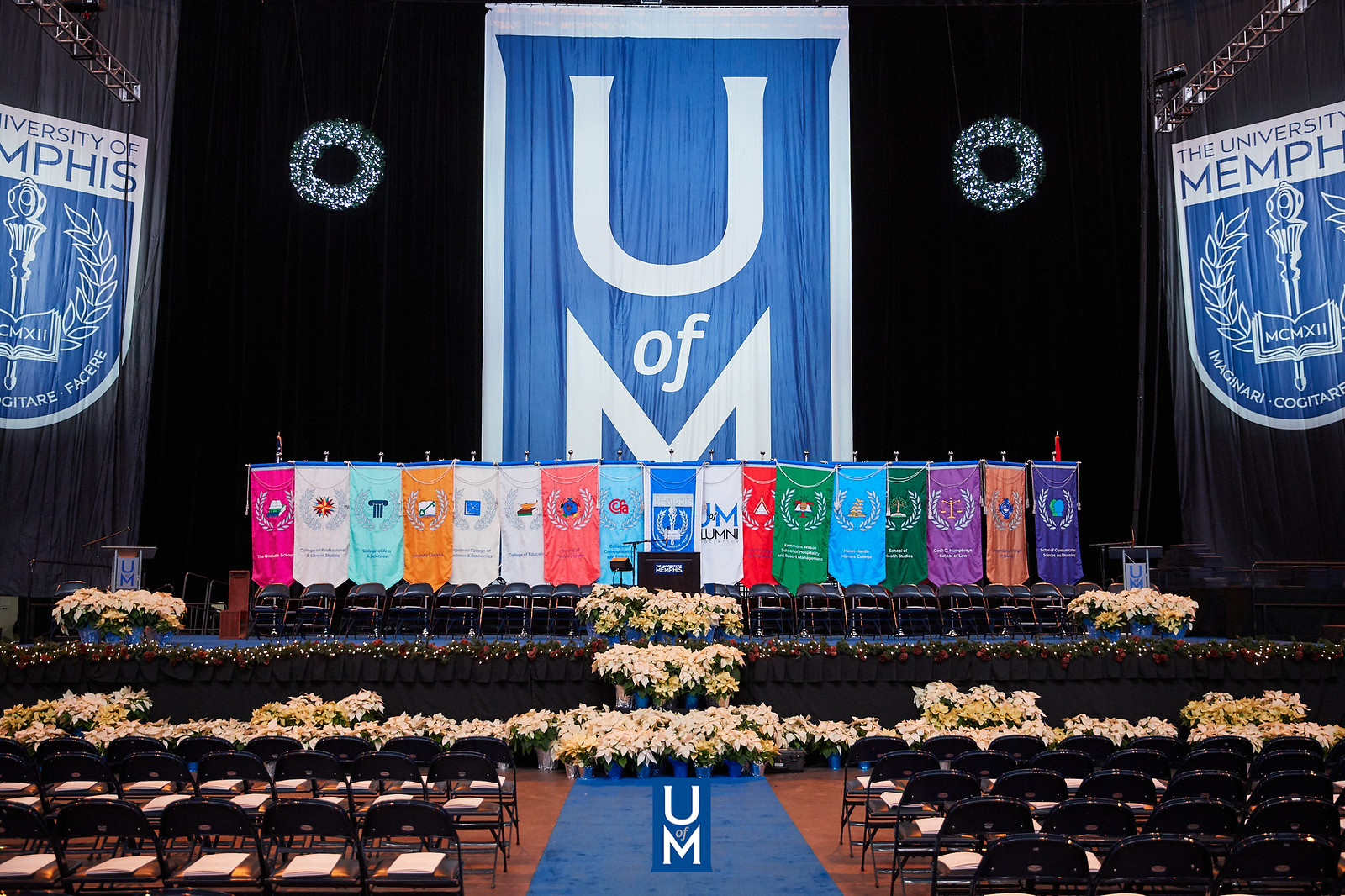
Electronic Theses and Dissertations
Identifier
6443
Date
2019
Document Type
Thesis
Degree Name
Master of Science
Major
Electrical and Computer Engr
Concentration
Computer Engineering
Committee Chair
Bashir I Morshed
Committee Member
Mohd Hasan Ali
Committee Member
Madhusudhanan Balasubramanian
Abstract
Irregularities in heartbeats and cardiac functioning outside of clinical settings are often not available to the clinicians, and thus ignored. But monitoring these with high-risk population might assist in early detection and continuous monitoring of Atrial Fibrillation(AF). Wearable devices like smart watches and wristbands, which can collect Electrocardigraph(ECG) signals, can monitor and warn users of unusual signs in a timely manner. Thus, there is a need to develop a real-time monitoring system for AF from ECG. We propose an algorithm for a simple beat-by-beat ECG signal multilevel classifier for AF detection and a quantitative severity scale (between 0 to 1) for user feedback. For this study, we used ECG recordings from MIT BIH Atrial Fibrillation, MIT BIH Long-term Atrial Fibrillation Database. All ECG signals are preprocessed for reducing noise using filter. Preprocessed signal is analyzed for extracting 39 features including 20 of amplitude type and 19 of interval type. The feature space for all ECG recordings is considered for Classification. Training and testing data include all classes of data i.e., beats to identify various episodes for severity. Feature space from the test data is fed to the classifier which determines the class label based on trained model. A class label is determined based on number of occurences of AF and other arrhythmia episodes such as AB(Atrial Bigeminy), SBR(Sinus Bradycardia), SVTA(Supra Ventricular Tacchyarrhythmia). Accuracy of 96.7764% is attained with Random Forest algorithm, Furthermore, precision and recall are determined based on correct and incorrect classifications for each class. Precision and recall on average of Random Forest Classifier are obtained as 0.968 and 0.968 respectievely. This work provides a novel approach to enhance existing method of AF detection by identifying heartbeat class and calculates a quantitative severity metric that might help in early detection and continuous monitoring of AF.
Library Comment
Dissertation or thesis originally submitted to the local University of Memphis Electronic Theses & dissertation (ETD) Repository.
Recommended Citation
Gollakota, Haritha, "Early Detection and Continuous Monitoring of Atrial Fibrillation from ECG Signals with a Novel Beat-Wise Severity Ranking Approach" (2019). Electronic Theses and Dissertations. 2009.
https://digitalcommons.memphis.edu/etd/2009
Comments
Data is provided by the student.