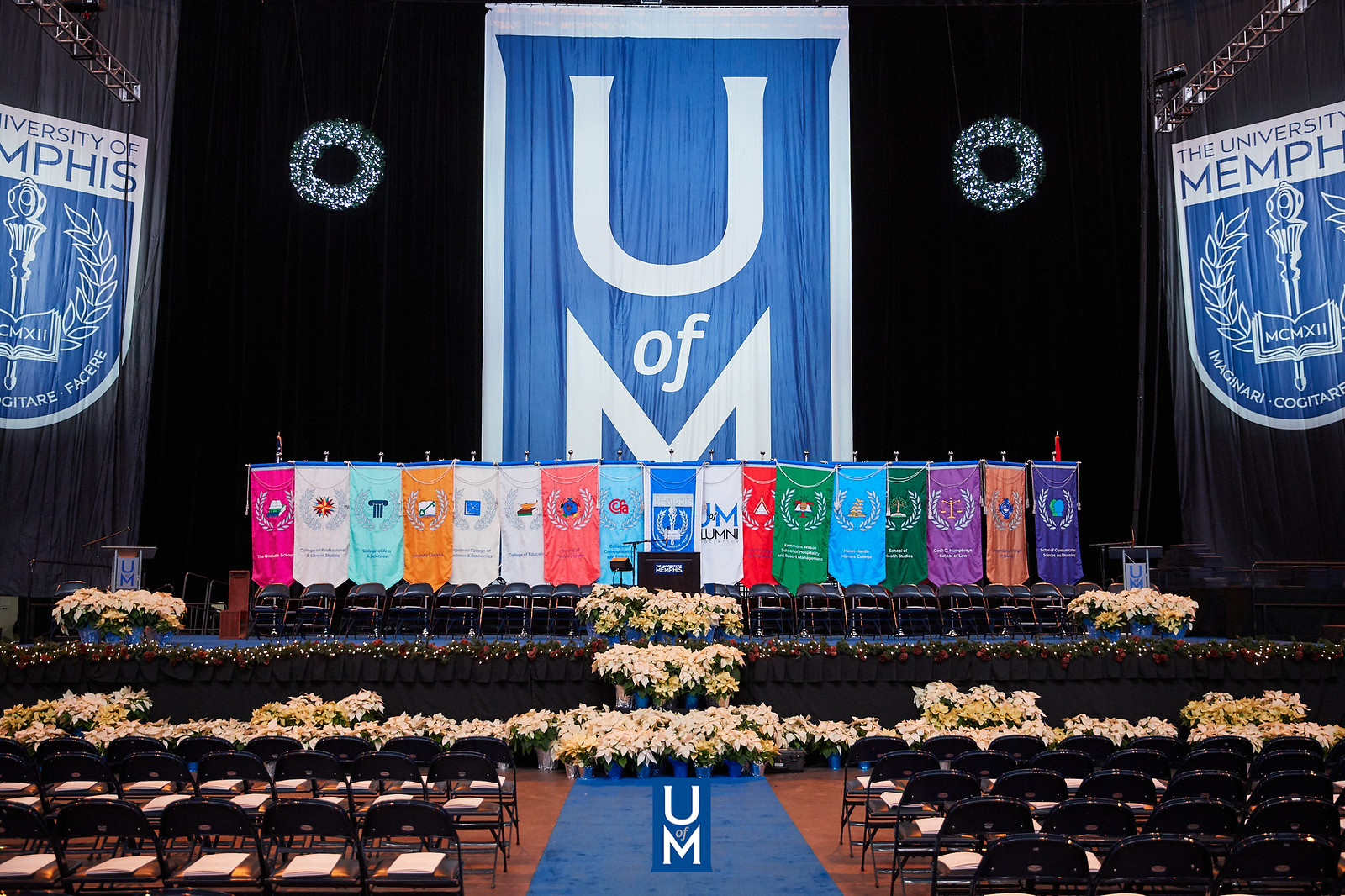
Electronic Theses and Dissertations
Identifier
6533
Date
2020
Document Type
Thesis
Degree Name
Master of Science
Major
Public Health
Concentration
Biostatistics
Committee Member
Hongmei Zhang
Committee Member
Yu Joyce Jiang
Committee Member
Dale Bowman
Abstract
The selection of variables in regression problems has occupied the minds of many statisticians. Several Bayesian variable selection methods have been developed, and we concentrate on the following method.This thesis which is a continuation of a project by Zhang textit{et al.} cite{Zha} published in Bayesian Analysis in 2016, first describes the general idea behind Bayes Inference, various sampling methods based on Bayes theorem.Then we present a Bayesian variable selection method based on an extension of the Zellner’s g-prior in linear models. More specifically, we propose a two-component G-prior, wherein a tuning parameter, calibrated by use of pseudo variables, is introduced to adjust the distance between the two components. We Assess the impact of tuning parameter b, the distance between important and unimportant variables, on the selection of variables by controlling Bayesian false model selection rate with respect to unimportant variables based on creating pseudo variables. We show that implementing the proposed prior in variable selection is more efficient than using the Zellner’s g-prior.
Library Comment
Dissertation or thesis originally submitted to the local University of Memphis Electronic Theses & dissertation (ETD) Repository.
Recommended Citation
Solatikia, Farnaz, "Understanding and assessment of a two-component G-prior in variable selection" (2020). Electronic Theses and Dissertations. 2060.
https://digitalcommons.memphis.edu/etd/2060
Comments
Data is provided by the student.