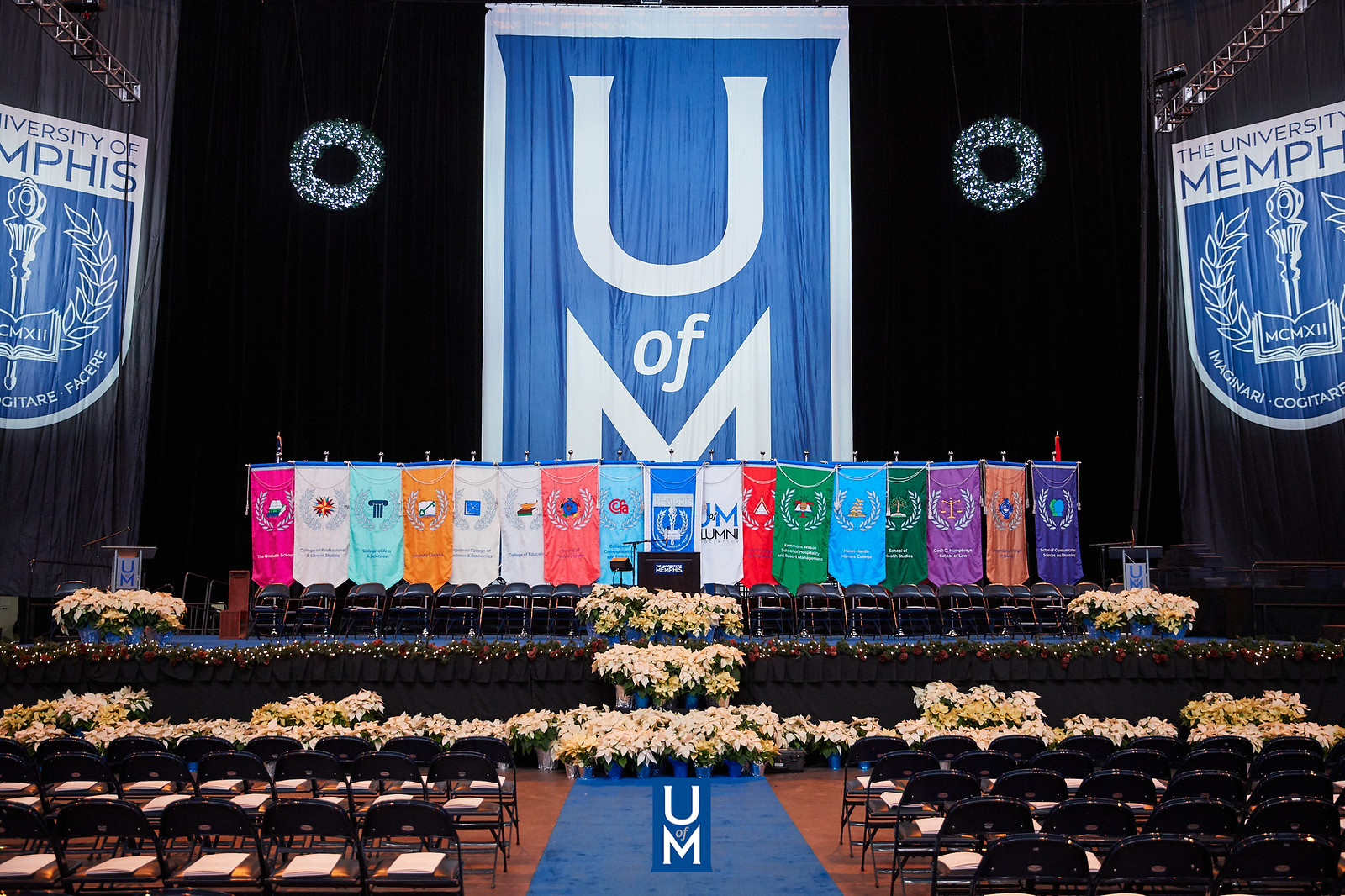
Electronic Theses and Dissertations
Identifier
6639
Date
2020
Document Type
Thesis
Degree Name
Master of Science
Major
Mechanical Engineering
Concentration
Power Systems
Committee Chair
Dr. Daniel Foti
Abstract
Wind turbine wakes are dominated by several energetic turbulent coherent structures that oscillate at specific Strouhal numbers. Implications on wind power harvesting of these dynamic, induced features require accurate unsteady modeling. Dynamic mode decomposition (DMD), a data-driven modal analysis, has demonstrated the ability to identify flow features based on specific frequencies. In this work, the selection of modes and data-driven DMD models pertaining to wakes with constant Strouhal number coherent structures are investigated using physically-informed criteria and sparse sampling. Both criteria are validated with a low Reynolds number flow behind a square cylinder. Next, the techniques are applied to data derived from the large-eddy simulation of a wind turbine wake. Modes related to tip vortices and hub vortex system are identified. Sparse identification shows remarkable ability to select the optimal modes for reduced-order modeling. Error becomes nearly independent of the number of modes when using fewer than 10% of the modes.
Library Comment
Dissertation or thesis originally submitted to the local University of Memphis Electronic Theses & dissertation (ETD) Repository.
Recommended Citation
Qatramez, Ala' Eyad, "Reduced-order Model Predictions of Wind Turbines via Mode Decomposition and Sparse Sampling" (2020). Electronic Theses and Dissertations. 2130.
https://digitalcommons.memphis.edu/etd/2130
Comments
Data is provided by the student.