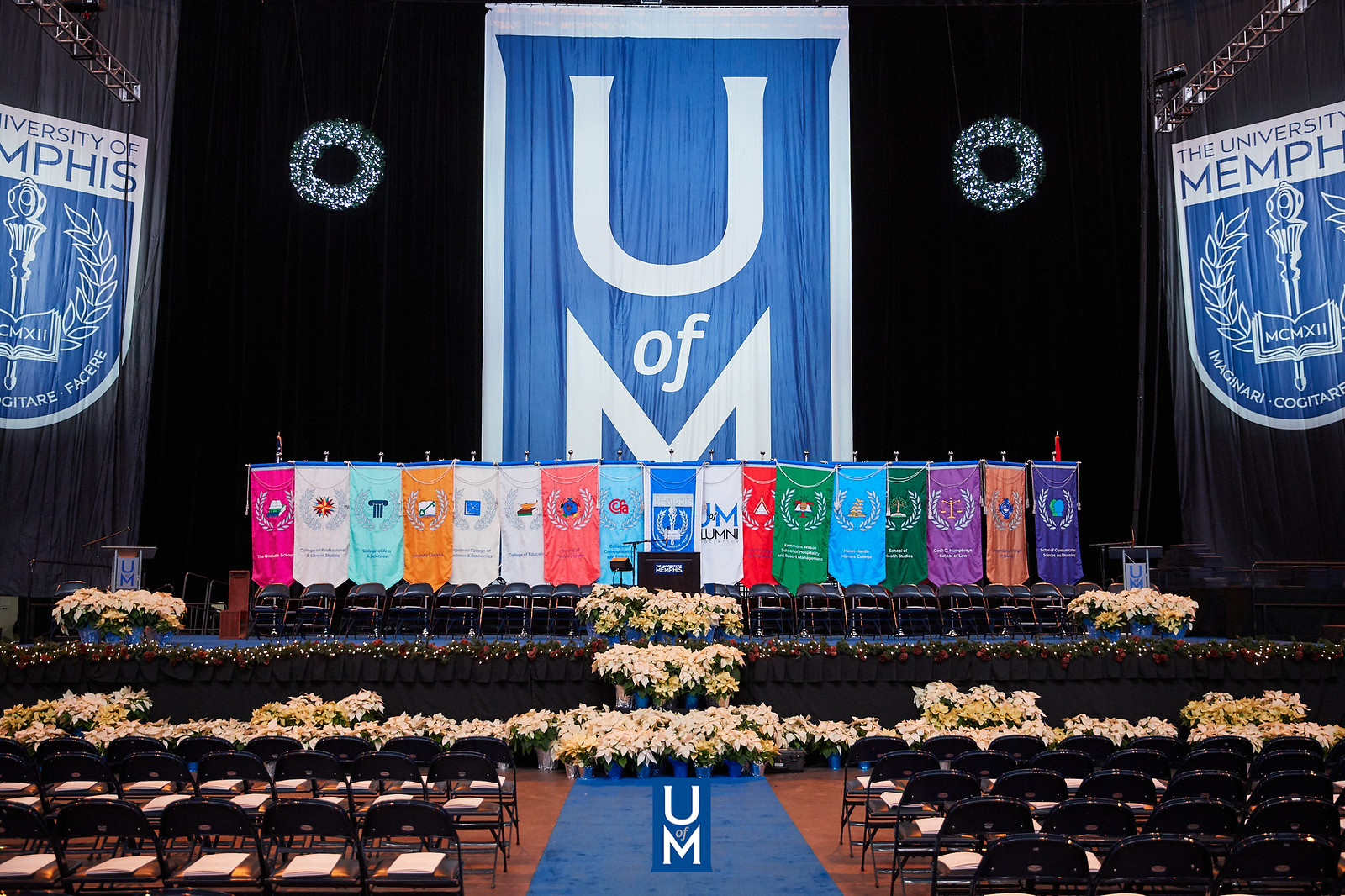
Electronic Theses and Dissertations
Identifier
6746
Date
2021
Document Type
Dissertation
Degree Name
Doctor of Philosophy
Major
Computer Science
Committee Chair
Dipankar Dasgupta
Committee Member
Nirman Kumar
Committee Member
Kan Yang
Committee Member
Deepak Venugopal
Abstract
Defenses against adversarial attacks are essential to ensure the reliability of machine learning models as their applications are expanding in different domains. Existing ML defense techniques have several limitations in practical use. I proposed a trustworthy framework that employs an adaptive strategy to inspect both inputs and decisions. In particular, data streams are examined by a series of diverse filters before sending to the learning system and then crossed checked its output through a diverse set of filters before making the final decision. My experimental results illustrated that the proposed active learning-based defense strategy could mitigate adaptive or advanced adversarial manipulations both in input and after with the model decision for a wide range of ML attacks by higher accuracy. Moreover, the output decision boundary inspection using a classification technique automatically reaffirms the reliability and increases the trustworthiness of any ML-Based decision support system. Unlike other defense strategies, my defense technique does not require adversarial sample generation, and updating the decision boundary for detection makes the defense systems robust to traditional adaptive attacks.
Library Comment
Dissertation or thesis originally submitted to the local University of Memphis Electronic Theses & dissertation (ETD) Repository.
Recommended Citation
Gupta, Kishor Datta, "Robust filtering schemes for machine learning systems to defend Adversarial Attacks" (2021). Electronic Theses and Dissertations. 2203.
https://digitalcommons.memphis.edu/etd/2203
Comments
Data is provided by the student.