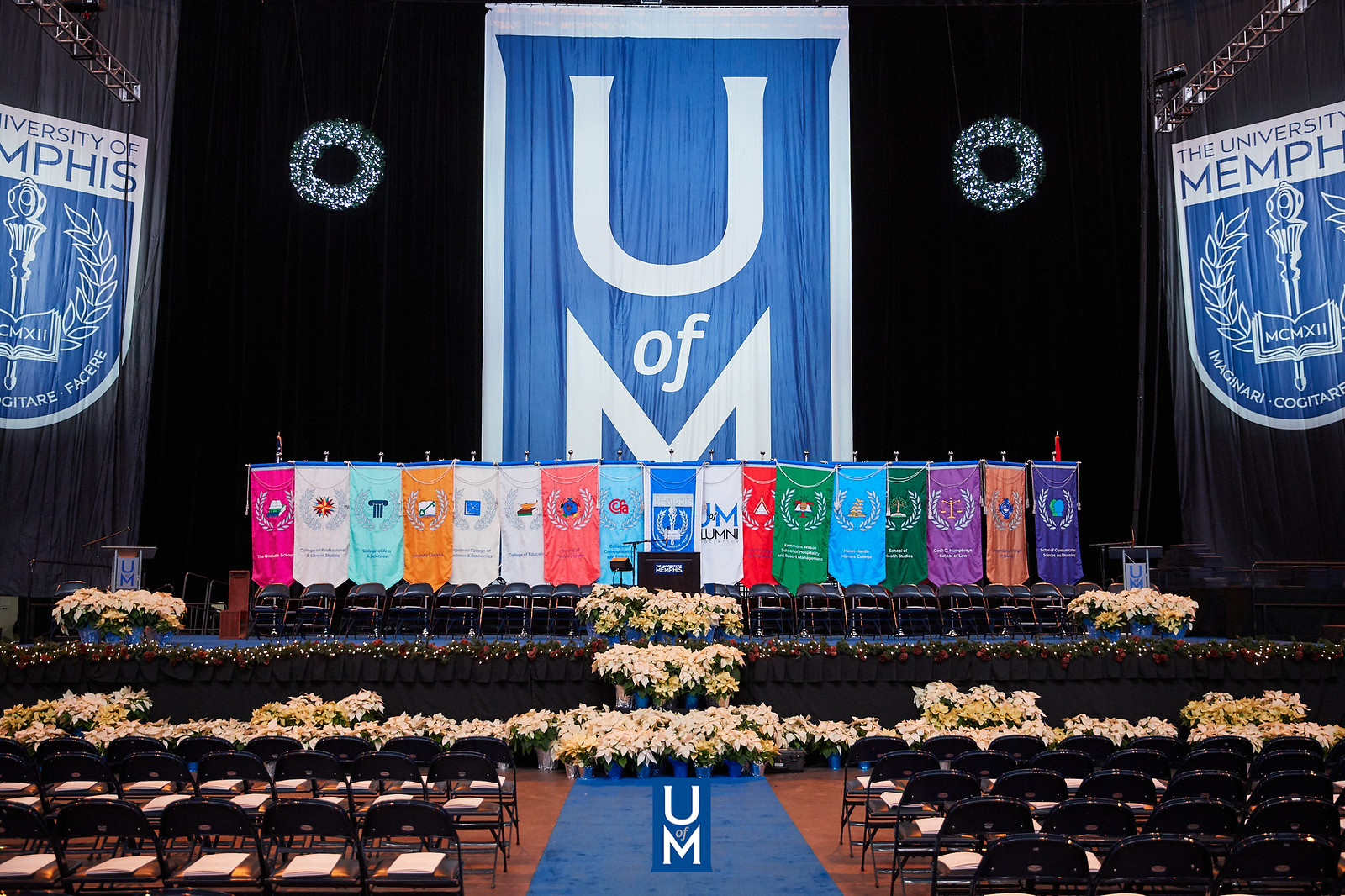
Electronic Theses and Dissertations
Identifier
1218
Date
2014
Document Type
Dissertation (Access Restricted)
Degree Name
Doctor of Philosophy
Major
Computer Science
Committee Chair
King-Ip Lin
Committee Member
Max M Louwerse
Committee Member
Vasile Rus
Committee Member
Scott Fleming
Abstract
Thematic role labeling has been an area of interest in several domains of natural language processing such as language generation and information retrieval. Extracting thematic roles efficiently and with minimal supervision affects all the above areas. Most of the existing computational methods for thematic role extraction are highly dependent on human annotated corpora, and are driven by the rules generated by supervised processes. In this work I extracted thematic roles using a data-driven approach. I built an unsupervised language generation model inspired from the ADIOS model, to learn recurring substructures from language, and with minimal supervision learned the rules that are needed to identify thematic roles. I tested the consistency of the sub-structures encoding thematic role information over PropBank annotated sentences. Results indicated that sub-structures consistently hold semantic role information, and the method robustly showed that thematic role information could be extracted with minimal supervision.
Library Comment
Dissertation or thesis originally submitted to the local University of Memphis Electronic Theses & dissertation (ETD) Repository.
Recommended Citation
Datla, Vivek Varma, "Date-Driven Approach For Thematic Role Extraction" (2014). Electronic Theses and Dissertations. 2231.
https://digitalcommons.memphis.edu/etd/2231
Comments
Data is provided by the student.