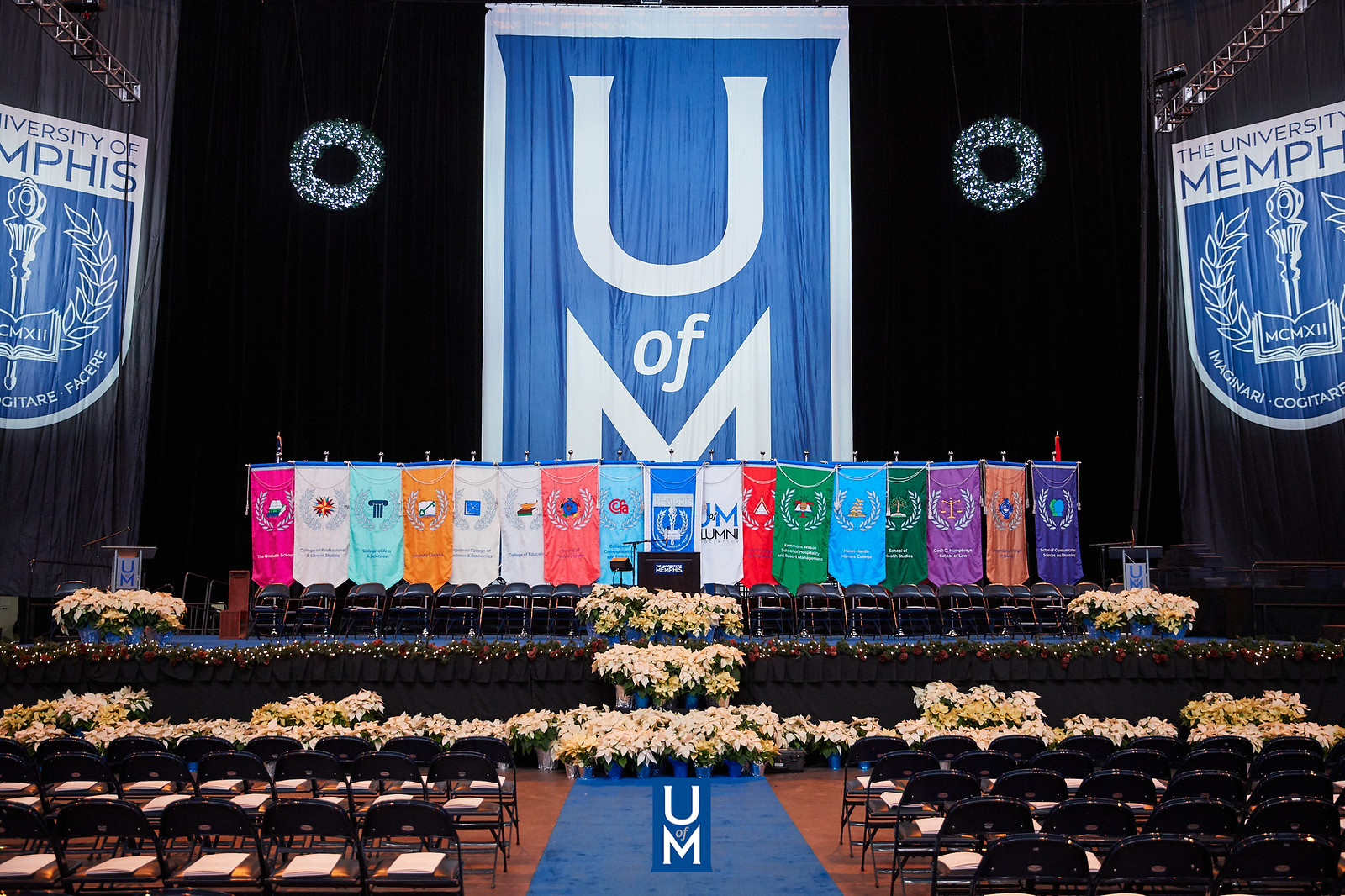
Electronic Theses and Dissertations
Identifier
4840
Date
2016
Document Type
Dissertation (Access Restricted)
Degree Name
Doctor of Philosophy
Major
Epidemiology
Committee Chair
Fawaz Mzayek
Committee Member
Vikki Nolan
Committee Member
Xinhua Yu
Committee Member
George Relyea
Abstract
Patients readmitted to the same or different healthcare facility within 30 days of initial inpatient discharge are of great concern to hospitals due to quality and financial implications. Prevention of unplanned hospital readmissions is one of the primary quality metrics mandated by the Centers for Medicare and Medicaid Services (CMS) under the Affordable Care Act (ACA). A retrospective longitudinal study was performed using medical records of the patients discharged from Methodist Le Bonheur Healthcare (MLH) between: January 1, 2007 and December 31, 2013. Textual data mining was performed on the unstructured data using SAS Enterprise Miner version 12.1® (SAS Institute, Cary, NC). Unstructured data elements were then created by using the keywords within the SCAN function in SAS version 9.4® (SAS Institute, Cary, NC). Descriptive statistics, bivariate and multivariable logistic regressions were used to determine the factors associated with unplanned readmission and multiple unplanned readmissions. Eight new variables created using text mining of unstructured data are: homeless, medication assistance, food stamps, travel vouchers/assistance, meal vouchers/assistance, morbid obesity, family support, and non-compliance to medications. We did not notice any improvement in the discrimination power or c-statistic for the enhanced multivariable logistic regression model (combination of structured and unstructured data elements) compared to the general model (only structured data elements). The c-statistic for general and enhanced models are: 0.74 (SA2: unplanned readmissions) and 0.62 (SA3: multiple unplanned readmissions). Even though “homelessness” and “family support” showed significance in bivariate results; none of these variables derived from the unstructured data stayed in the final enhanced model, highlighting that solely depending on unstructured data may not be a good research strategy.This study demonstrated the process of developing variables from unstructured data and how they can be added to the structured data to explore a complicated topic like unplanned and multiple (two or more) unplanned readmissions.
Library Comment
Dissertation or thesis originally submitted to the local University of Memphis Electronic Theses & dissertation (ETD) Repository.
Recommended Citation
Podila, Pradeep Srinivasa Brahma, "Exploring Opportunities Towards Reducing Unplanned Hospital Readmissions in Memphis" (2016). Electronic Theses and Dissertations. 2247.
https://digitalcommons.memphis.edu/etd/2247
Comments
Data is provided by the student.