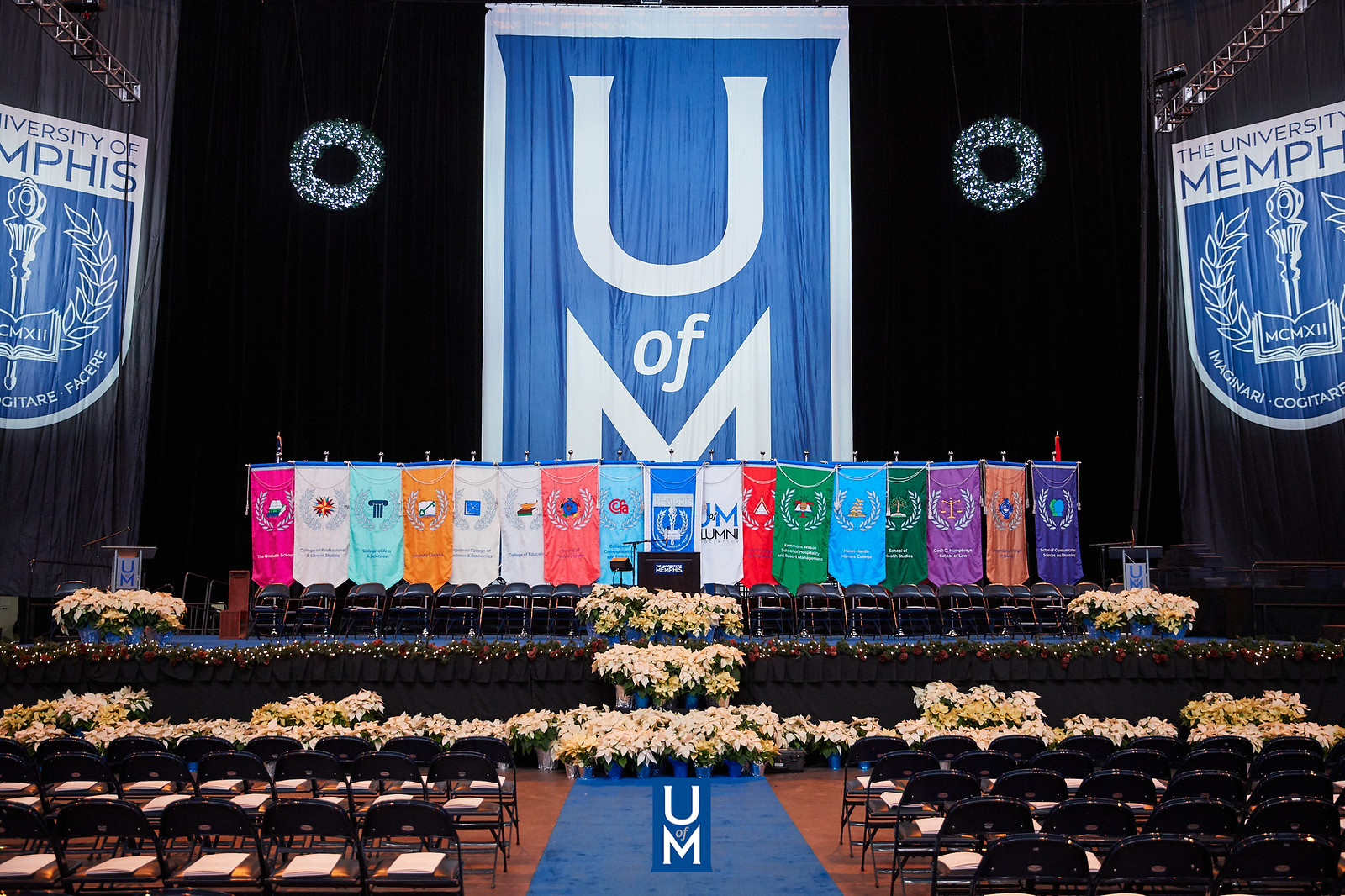
Electronic Theses and Dissertations
Identifier
6089
Date
2017-12-04
Document Type
Thesis (Campus Access Only)
Degree Name
Master of Science
Major
Electrical and Computer Engr
Concentration
Computer Engineering
Committee Chair
Xiangen Hu
Committee Member
Philip Pavlik
Committee Member
Madhusudhanan Balasubramanian
Abstract
Knowledge tracing is one of the major focus of modern artificial intelligence (AI) enabled intelligent tutoring systems (ITS). There have been a large variety of methods applied to modeling student knowledge within the educational data mining community. Not all of the models perform equally well in all varieties of datasets. However, there is no guidance for selecting a method for a particular situation. In this work, we attempted to understand the aspects of datasets that make them suitable for a particular knowledge tracing model, focusing specifically on the widely used Performance Factor Analysis models and current state-of-the-art, Deep Knowledge Tracing (DKT) model. Through recent developments in deep learning, DKT was explored as a method to improve upon traditional methods of analysis, but this method performs poorly with smaller dataset collected from a controlled experiment. We propose structural changes in Long short-term memory (LSTM) model used in DKT. The new LSTM model with deep autoencoder and other important recent performance features as input outperforms current DKT models. This new model is proven to be better capable of tracking learners’ knowledge level.
Library Comment
Dissertation or thesis originally submitted to the local University of Memphis Electronic Theses & dissertation (ETD) Repository.
Recommended Citation
Hossain, Md Iqbal, "Comparative Analysis of Knowledge Tracing Methods" (2017). Electronic Theses and Dissertations. 2311.
https://digitalcommons.memphis.edu/etd/2311
Comments
Data is provided by the student.