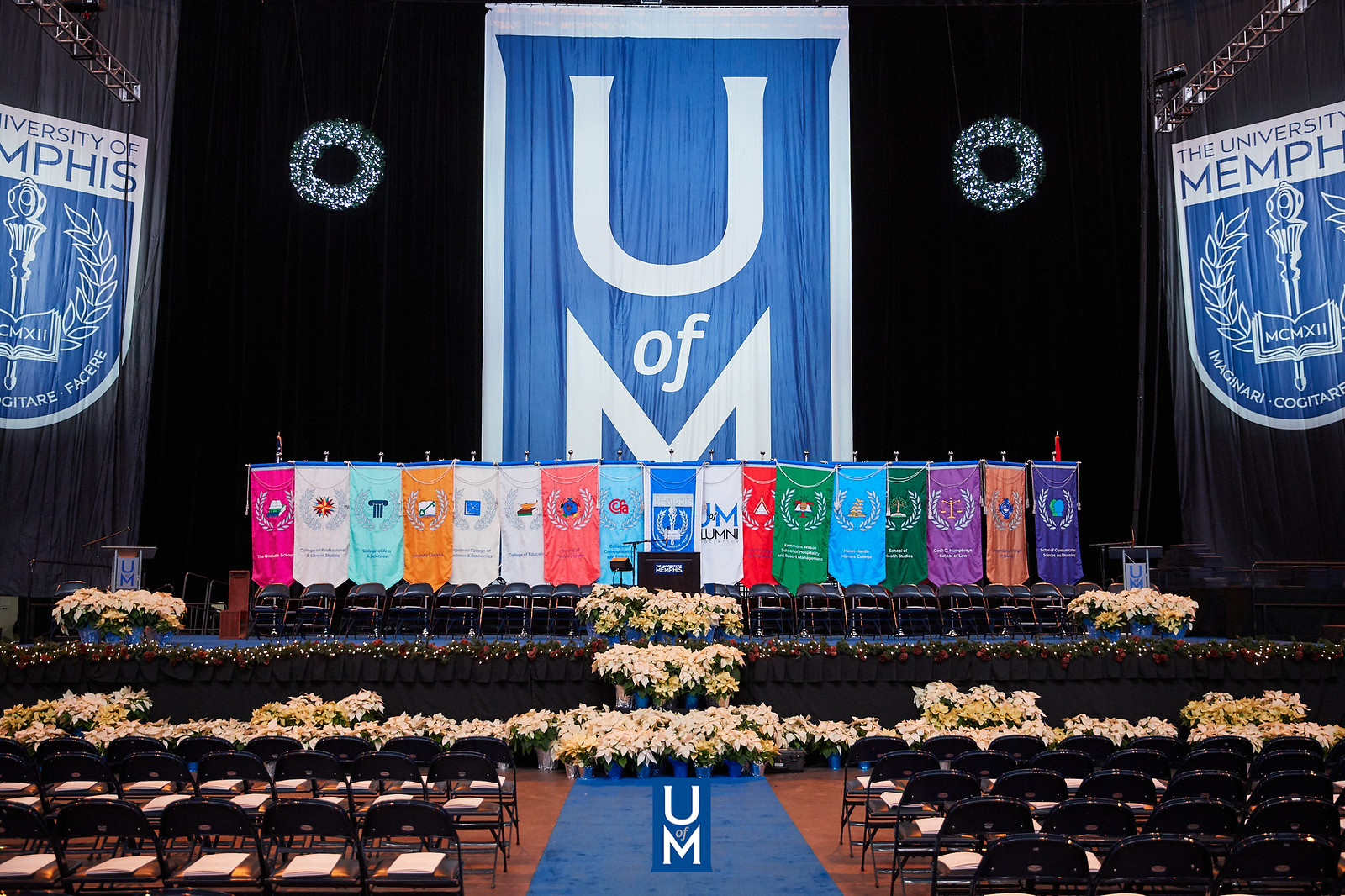
Electronic Theses and Dissertations
LRN: Limitless Routing Networks for Effective Multi-task Learning
Identifier
6737
Date
2021-07-22
Document Type
Thesis (Campus Access Only)
Degree Name
Master of Science
Major
Computer Science
Committee Chair
Xiaofei Zhang
Committee Member
Weizi Li
Committee Member
Deepak Venugopal
Abstract
Multi-task learning (MTL) is a field involved with learning multiple tasks simultaneously typically through using shared model parameters. The shared representation enables generalized parameters that are task invariant and assists in learning tasks with sparse data. However, the presence of unforeseen task interference can cause one task to improve at the detriment of another. A recent paradigm constructed to tackle these types of problems is the routing network, that builds neural network architectures from a set of modules conditioned on the input instance, task, and previous output of other modules. This approach has many constraints, so we propose the Limitless Routing Network (LRN) which removes the constraints through the usage of a transformer-based router and a reevaluation of the state and action space. We also provide a simple solution to the module collapse problem and display superior accuracy performance over several MTL benchmarks compared to the original routing network.
Library Comment
Dissertation or thesis originally submitted to the local University of Memphis Electronic Theses & dissertation (ETD) Repository.
Recommended Citation
Wickman, Ryan Patrick, "LRN: Limitless Routing Networks for Effective Multi-task Learning" (2021). Electronic Theses and Dissertations. 2329.
https://digitalcommons.memphis.edu/etd/2329
Comments
Data is provided by the student.