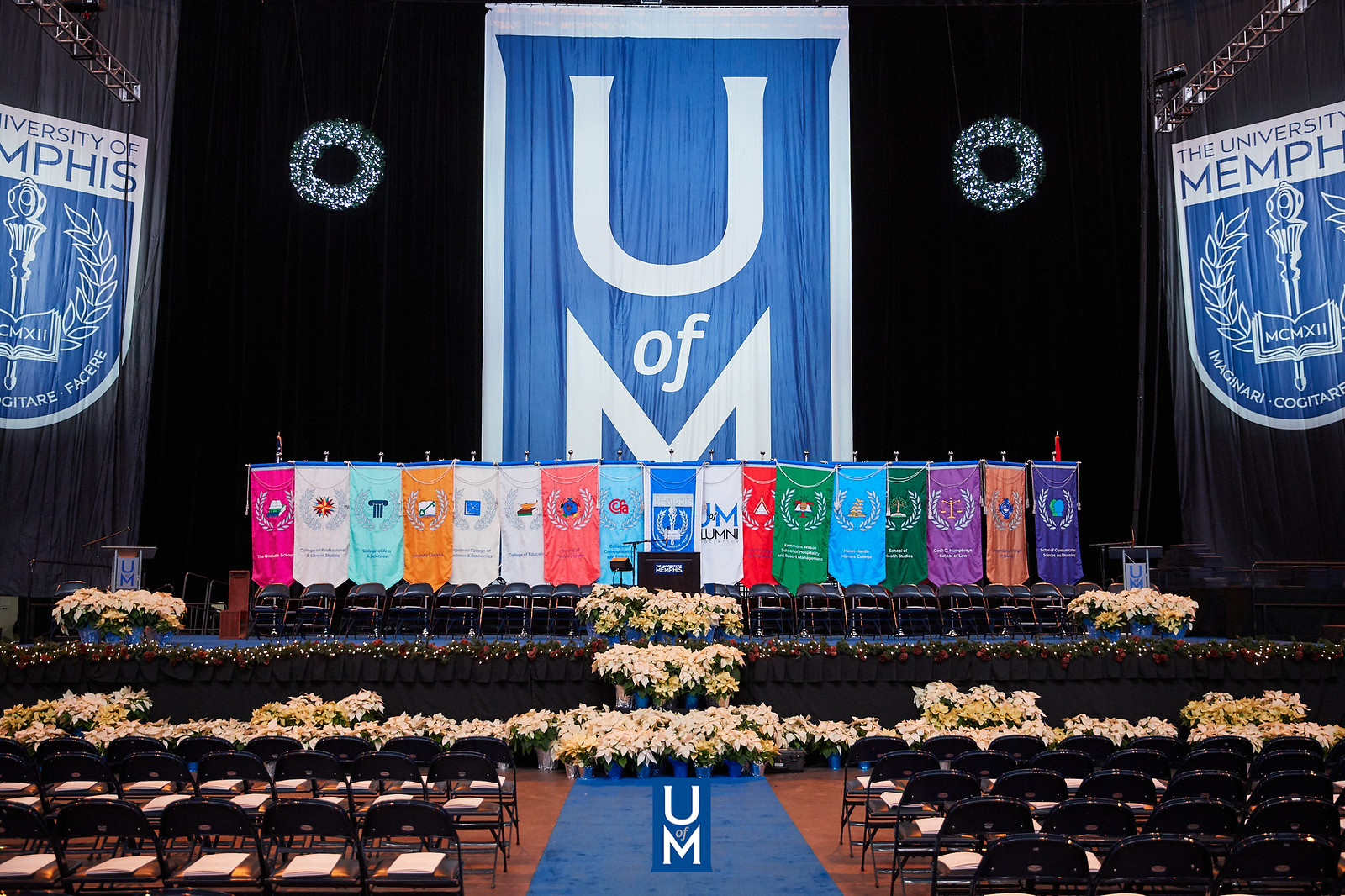
Electronic Theses and Dissertations
Identifier
663
Date
2012-07-20
Document Type
Thesis (Campus Access Only)
Degree Name
Master of Science
Major
Electrical and Computer Engr
Concentration
Computer Engineering
Committee Chair
Mohammed Yeasin
Committee Member
Aaron L Robinson
Committee Member
Bashir Morshed
Abstract
Significant progress has been made in applying text mining to named entity recognition, text classification, terminology extraction, relationship extraction and hypothesis generation. Businesses, government agencies, and researchers are gaining a competitive advantage through the use of text mining and content analytics from unstructured data. However, mining and classification of short-text with high accuracy remains a formidable challenge. To address this problem, a semantic-sensitive query expansion technique was developed and applied on a number of corpuses. The Query Expanded Latent Semantic Analysis (QE-LSA) model was developed from large short-text corpus with user defined, data driven and dynamic dictionary. Empirical analyses on two datasets obtained from different domains were conducted to illustrate the efficacy of QE-LSA. A number of experiments were conducted to understand the role of various tuning of parameters in maximizing the performance. The results suggest that this model performs robustly on both corpuses.
Library Comment
Dissertation or thesis originally submitted to the local University of Memphis Electronic Theses & dissertation (ETD) Repository.
Recommended Citation
Nag, Abhijit Kumar, "Semantic-sensitive Query Expansion Method for Short-text Analytics and Mining" (2012). Electronic Theses and Dissertations. 2339.
https://digitalcommons.memphis.edu/etd/2339
Comments
Data is provided by the student.