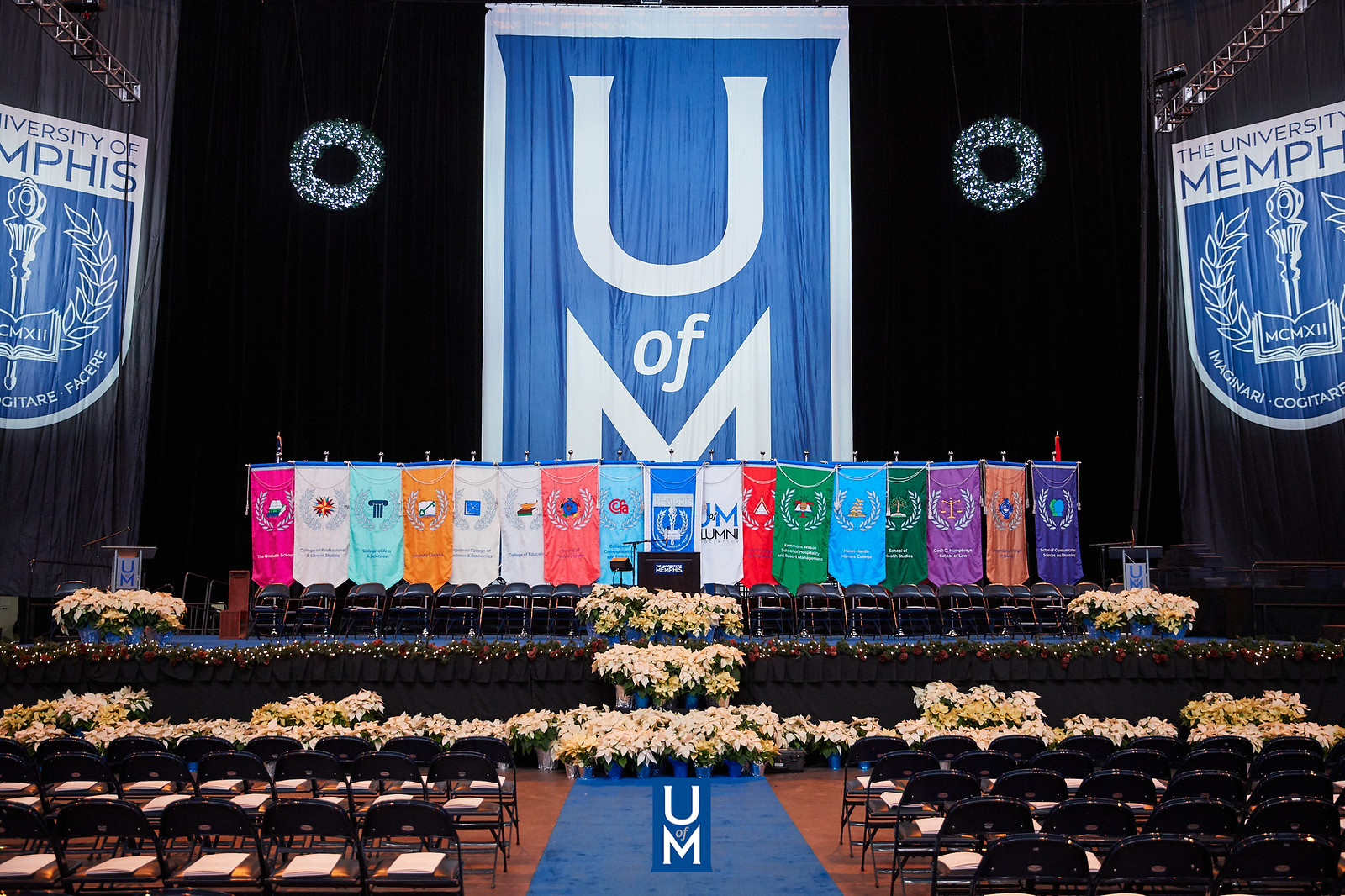
Electronic Theses and Dissertations
Identifier
6775
Date
2021
Document Type
Thesis
Degree Name
Master of Science
Major
Electrical and Computer Engr
Committee Chair
Ana Doblas
Committee Member
Madhu Balasubramanian
Committee Member
Aaron Robinson
Abstract
Glucose monitoring technologies allow users to monitor glycemic fluctuations (e.g., current glucose levels in their blood, also known as glycemia). This is particularly important for individuals who suffer from diabetes mellitus (DM), commonly referred to as diabetes. Traditional self-monitoring blood glucose (SMBG) devices require the user to prick their finger and extract a blood drop to measure the blood glucose based on chemical reactions with the blood. Unlike traditional glucometer devices, non-invasive continuous glucose monitoring (NICGM) devices aim to solve these issues by consistently monitoring users’ blood glucose levels (BGL) and without invasively acquiring a sample. This Master Thesis aims to investigate the feasibility of a novel approach to NICGM via the use of off-the-shelf wearable sensors and the integration of learning-based models (i.e., machine learning). Several sensors were purchased to generate our own dataset with an increased feature set for studying possible relationships between glucose and non-invasive biometric measurements. Two datasets were collected for this study: (1) the OhioT1DM dataset, which is a publicly available dataset that can be obtained by contacting Ohio University; and (2) the UofM dataset, which was created by this research team. Both the Ohio dataset and our UofM dataset are passed through a machine learning pipeline that tests several models to determine whether the features are sufficient for predicting blood glucose concentrations. While preliminary results seem optimistic, a larger dataset is required to make conclusions about the feasibility of this approach.
Library Comment
Dissertation or thesis originally submitted to the local University of Memphis Electronic Theses & dissertation (ETD) Repository.
Recommended Citation
Bogue Jimenez, Brian Allen, "Exploring Non-Invasive Features for Continuous Glucose Monitoring" (2021). Electronic Theses and Dissertations. 2360.
https://digitalcommons.memphis.edu/etd/2360
Comments
Data is provided by the student.