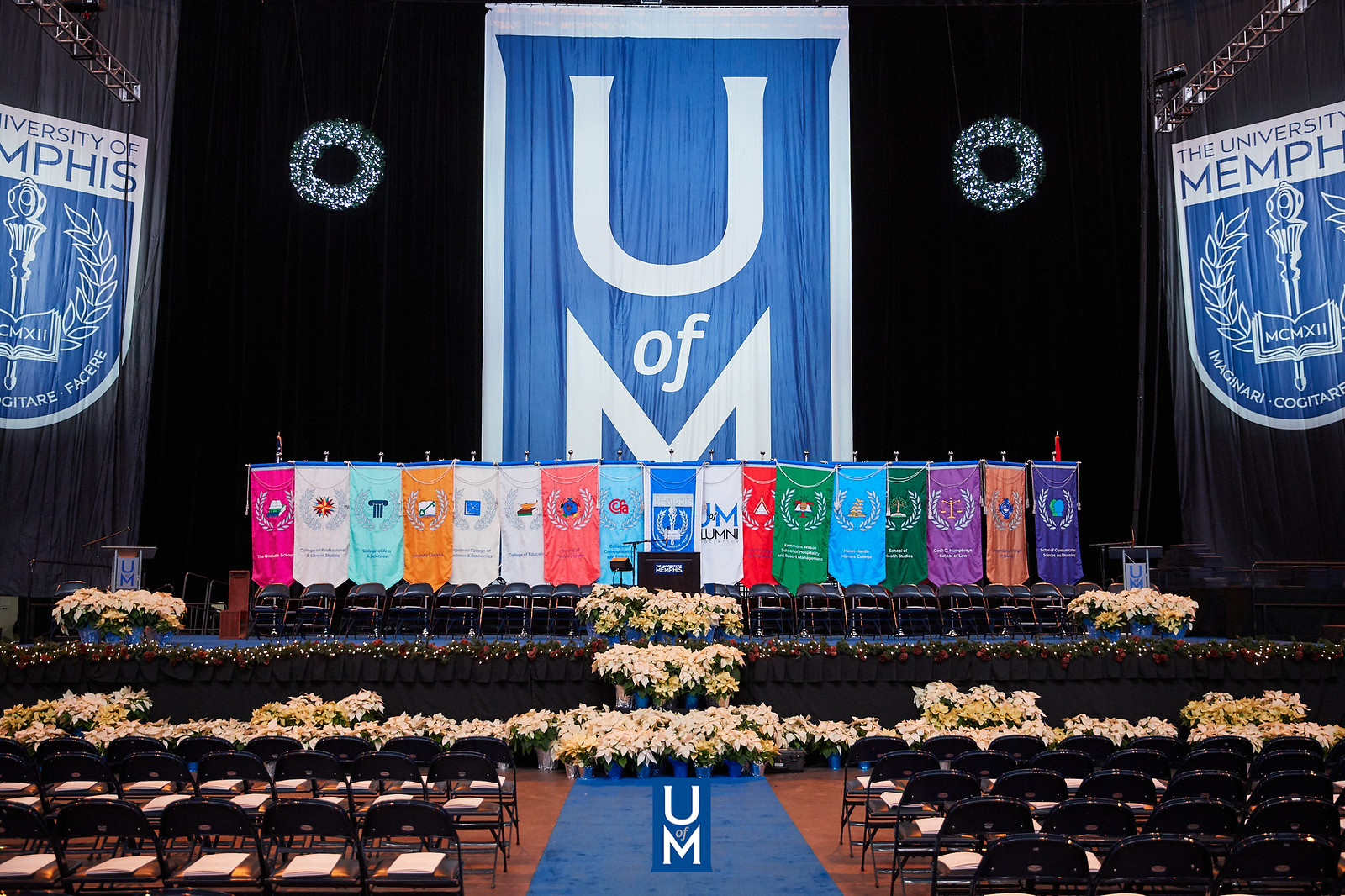
Electronic Theses and Dissertations
Date
2021
Document Type
Dissertation
Degree Name
Doctor of Philosophy
Department
Electrical & Computer Engineering
Committee Chair
Mohammed Yeasin
Committee Chair
Gavin Bidelman
Committee Member
Mohammed Yeasin
Committee Member
Gavin Bidelman
Committee Member
Eugene Eckstein
Committee Member
Madhusudhanan Balasubramanian
Abstract
Categorical perception (CP) of audio is critical to understand how the human brainperceives speech sounds despite widespread variability in acoustic properties. Most studiesthat examine cognitive speech processing have applied methodological approaches that focuson specific or a set of brain regions. Departing from hypothesis-driven approaches, in thisdissertation, we proposed multivariate data-driven approaches to identify the spatiotemporal(i.e., when in time and where in the brain) and spectral (i.e., frequency-band power levels)characteristics of auditory neural activity that reflects CP for speech (i.e., differentiatesphonetic prototypes from ambiguous speech sounds). We recorded 64-channel EEG aslisteners rapidly classified vowel sounds along an acoustic-phonetic continuum. We usedparameter optimized support vector machine (SVM), k-nearest neighbors (KNN) classifiers,and stability selection to determine spatiotemporal and spectral characteristics of neuralactivity that decode CP best via source-level neural activity. Using event-related potentials(ERPs), we found that early (120 ms) whole-brain data decoded speech categories (i.e.,prototypical vs. ambiguous speech tokens) with 95.16% accuracy [area under the curve(AUC) 95.14%; F1-score 95.00%]. Separate analyses on the left hemisphere (LH) and righthemisphere (RH) responses showed that LH decoding was more accurate and earlier than RH(89.03% vs. 86.45% accuracy; 140 ms vs. 200 ms). Stability (feature) selection identified 13regions of interest (ROIs) out of 68 brain regions (including auditory cortex, supramarginalgyrus, and inferior frontal gyrus (IFG)) that showed categorical representation duringstimulus encoding (0-260 ms). In contrast, 15 ROIs (including fronto-parietal regions, IFG,motor cortex) were necessary to describe later decision stages (later 300 to 800 ms) ofcategorization but these areas were highly associated with the strength of listenerscategorical hearing (i.e., slope of behavioral identification functions).viMoreover, our induced vs. evoked mode analysis shows that whole-brain evoked -bandactivity decoded prototypical from ambiguous speech sounds with ~70% accuracy. However,induced -band oscillations showed better decoding of speech categories with ~95% accuracycompared to evoked -band activity (~70% accuracy). Induced high frequency (-band)oscillations dominated CP decoding in the LH, whereas lower frequency (-band) dominateddecoding in the RH. In addition, feature selection identified 14 brain regions carryinginduced activity and 22 regions of evoked activity that were most salient in describingcategory-level speech representations. Among the areas and neural regimes explored, wefound that induced -band modulations were most strongly associated with listenersbehavioral CP.In sum, our data-driven multivariate models demonstrate that abstract categories emergesurprisingly early (~120 ms) in the time course of speech processing and are dominated byengagement of a relatively compact fronto-temporal-parietal brain network. In addition, thecategory-level organization of speech is dominated by relatively high frequency inducedbrain rhythms.
Library Comment
Dissertation or thesis originally submitted to ProQuest
Notes
Open Access
Recommended Citation
MAHMUD, MD SULTAN, "MULTIVARIATE ANALYSIS FOR UNDERSTANDING COGNITIVE SPEECH PROCESSING" (2021). Electronic Theses and Dissertations. 2657.
https://digitalcommons.memphis.edu/etd/2657
Comments
Data is provided by the student.