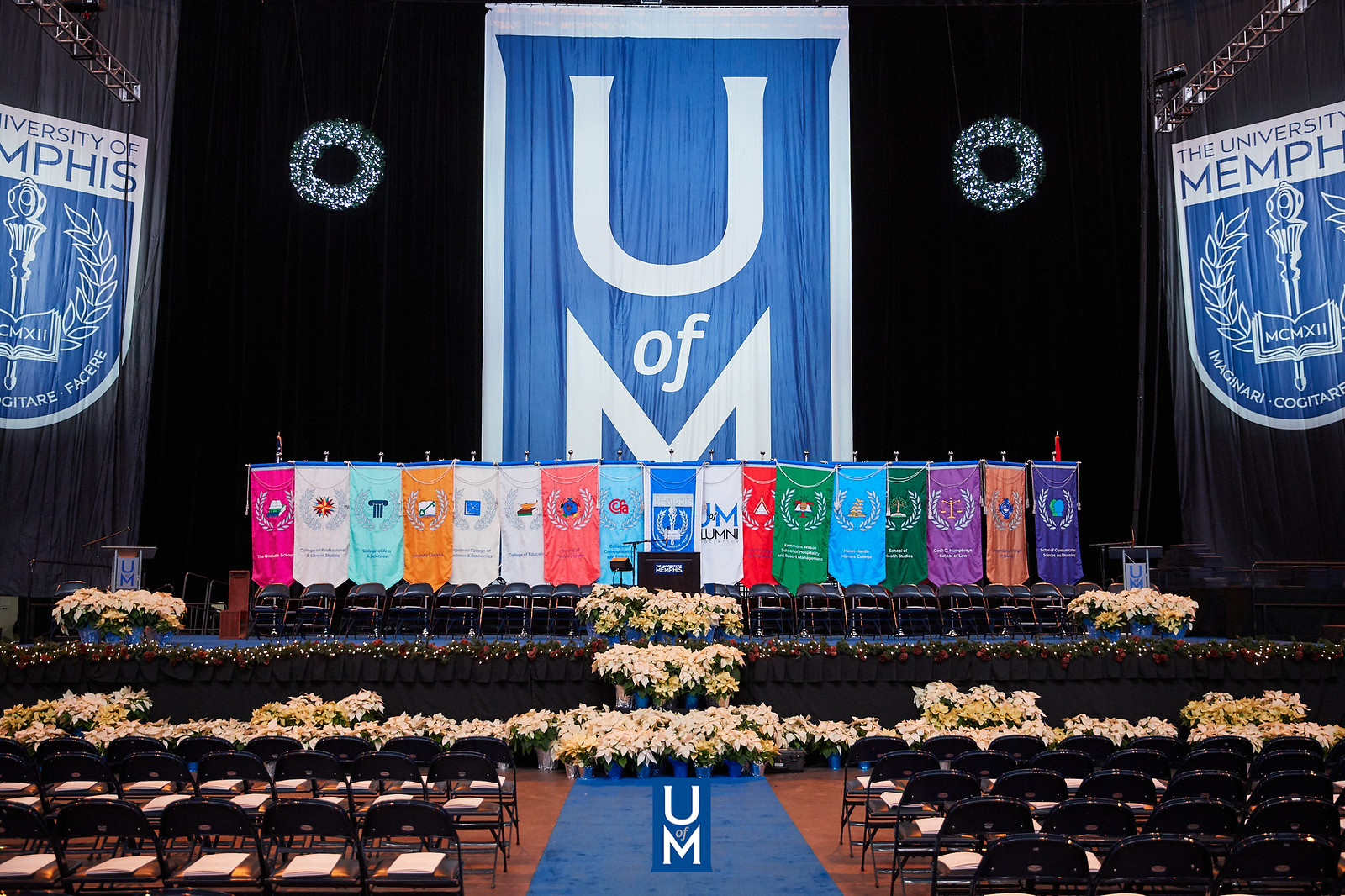
Electronic Theses and Dissertations
Date
2020
Document Type
Dissertation
Degree Name
Doctor of Philosophy
Department
Mathematical Sciences
Committee Chair
E. Olusegun George
Committee Member
Dale Bowman
Committee Member
Hongmei Zhang
Committee Member
Fridtjof Thomas
Abstract
In clinical trials and observational studies, clinicians often observe measurements on multiple causes of clinical progression or synthesize information from various sources. These measurements are collected because a single outcome is usually inadequate to describe the disease complexities or because the primary outcomes of interest are abstract constructs (e.g., quality of life, disease conditions) that cannot be measured directly. It is usually necessary to collect multiple endpoints in order to fully understand the true associations that exist among several clinical outcomes and how they jointly affect the primary outcomes. In addition, such datasets are often useful for characterizing treatment effectiveness, evaluate the risk-factors, or investigate the impact of health policy initiatives. Examples of multivariate mixed outcomes data are ubiquitous in biomedical and bio-pharmaceutical studies, psychometric, behavioral research, and pre-clinical teratology and developmental toxicity studies, among others. The different data structures of endpoints present interesting statistical and computational challenges. For example, there would be several levels of correlations inherent in the outcomes data, especially when dealing with a clustered or longitudinal design. The common modeling strategy of analyzing each endpoint separately in a univariate manner usually leads to misleading findings because such an approach ignores the correlations and interactions among the outcomes. The introduction of high-dimensional covariates such as gene expressions and large dimensional clinical information further exacerbates the modeling and analysis (the $ p \gg n $ problem), leading to a need for sophisticated variable selection strategy. While variable selection methods are well-developed for many statistical models, the procedure is underdeveloped for multivariate mixed endpoints. This dissertation is motivated by the statistical and computational challenges that arise from analyzing such data. The overarching goal of this dissertation is to develop statistical procedures for jointly modeling, estimation, and efficient identification of significant predictors in the analysis of multivariate clustered/longitudinal mixed endpoints datasets that are characterized by high-dimensional covariates. Specifically, we develop a procedure to guide both the model estimation and the efficient extraction of potential active predictors. We demonstrate the advantages of our procedure in terms of variable selection, prediction, and computational scalability via extensive simulations study and apply the method to two real-life datasets. In addition to other properties, we find that the estimates identified by dynamic posterior exploration in our procedure stabilize rapidly and very early in their trajectories, especially in the implementation of the dynamic weighted LASSO.
Library Comment
Dissertation or thesis originally submitted to ProQuest
Recommended Citation
Olufadi, Yunusa, "Approaches for Analyzing Multivariate Mixed Endpoints With High-Dimensional Covariates." (2020). Electronic Theses and Dissertations. 2702.
https://digitalcommons.memphis.edu/etd/2702
Comments
Data is provided by the student.