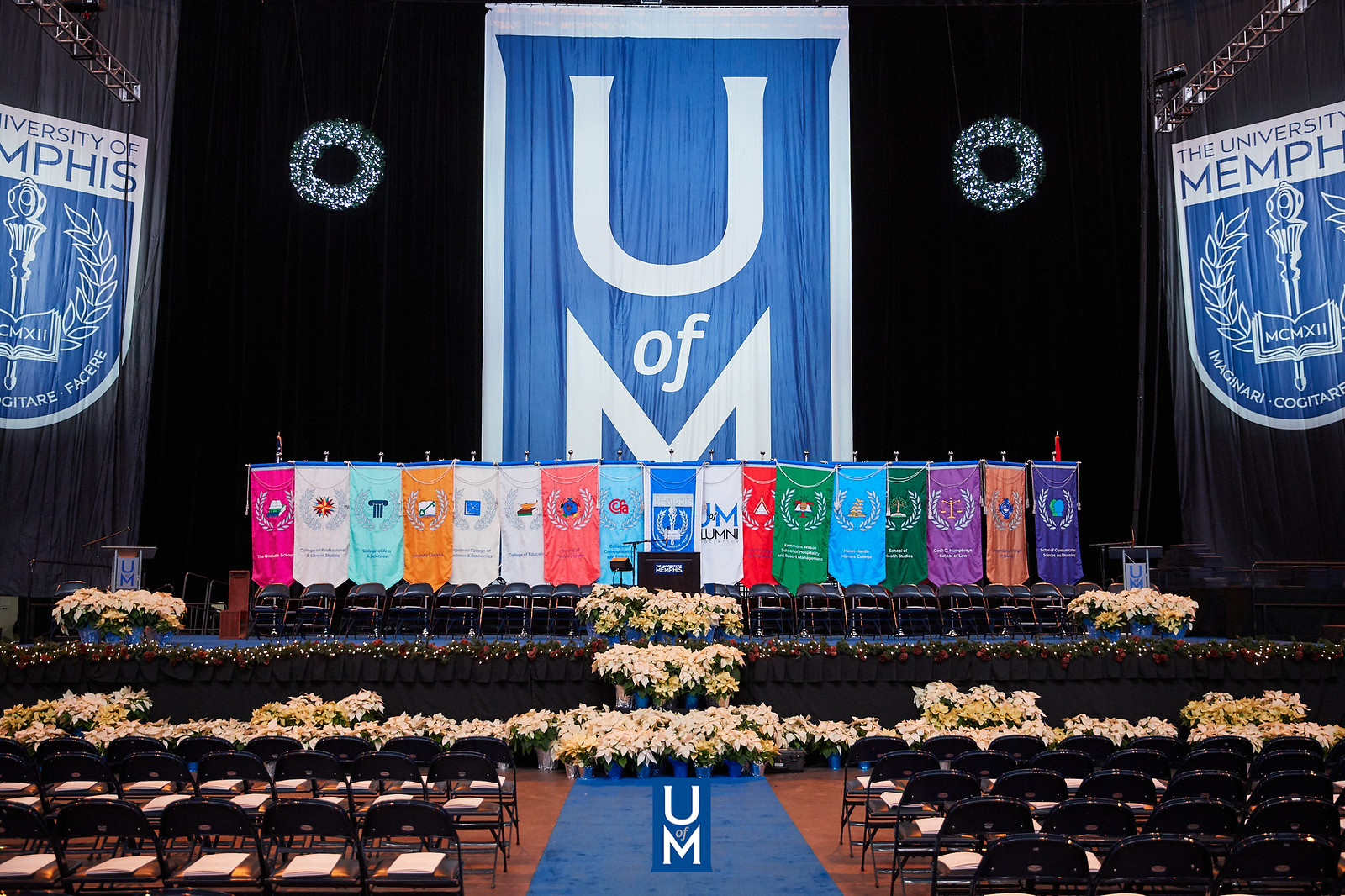
Electronic Theses and Dissertations
Date
2021
Document Type
Thesis
Degree Name
Master of Public Health
Department
Health Studies
Committee Chair
Meredith Ray
Committee Member
Tit-Yee Wong
Committee Member
Yu (Joyce) Jiang
Abstract
Factorial design is one of the best ways to screen different factors in a system of study. It allows the study of the main effects and their interactions at the same time. We used the 2k-factorial design to screen different factors that affect the bacterial sensitivity to antibiotics. Through simulation studies, we examined the sensitivity and specificity of four different approaches to analyze the factorial data under different scenarios. The parametric ANOVA showed higher sensitivity and specificity for normally distributed data, but it failed largely for non-normal data. Rank-ANOVA had very high sensitivity and specificity for both normal and non-normal data when replicates were higher. Aligned-rank approach also had higher sensitivity and specificity for normal data, but not for non-normal data. However, it was very sensitive to outliers and thus failed to detect the significant interactions in our settings. Similarly, permutation approach failed to detect the significant interactions largely for non-normal distributions and needed higher number of observations for larger number of factors to run without error. Overall, rank-based ANOVA was the robust approach to analyze the factorial data without losing the statistical power under our simulation settings. We also used the above-mentioned approaches on our real data obtained from 2k factorial design. Model trimming options are not available in some of the functions that we used and therefore only full models were compared. Based on root mean squared error (RMSE) values all four approaches, except the permutation test, were similar in predicting the response variable. Permutation test was not applicable with all 7 factors and their interactions in the model due to a smaller number of observations, so reduced model without the factor temperature was used for the analysis. All four approaches yielded comparable p-value for the highest order interaction with 6 factors in the model but when 7 factors were in the model, rank ANOVA had different p-value than other approaches. Since, factorial data with larger number of factors are difficult to run with multiple replicates, assumptions of parametric approach may hold true. Non-parametric approaches like rank-based and aligned-rank-based approaches are more suitable for the purpose.
Library Comment
Dissertation or thesis originally submitted to ProQuest
Recommended Citation
Shrestha, Prabin, "PARAMETRIC AND NON-PARAMETRIC APPROACHES TO FACTORIAL DATA ANALYSIS." (2021). Electronic Theses and Dissertations. 2777.
https://digitalcommons.memphis.edu/etd/2777
Comments
Data is provided by the student.