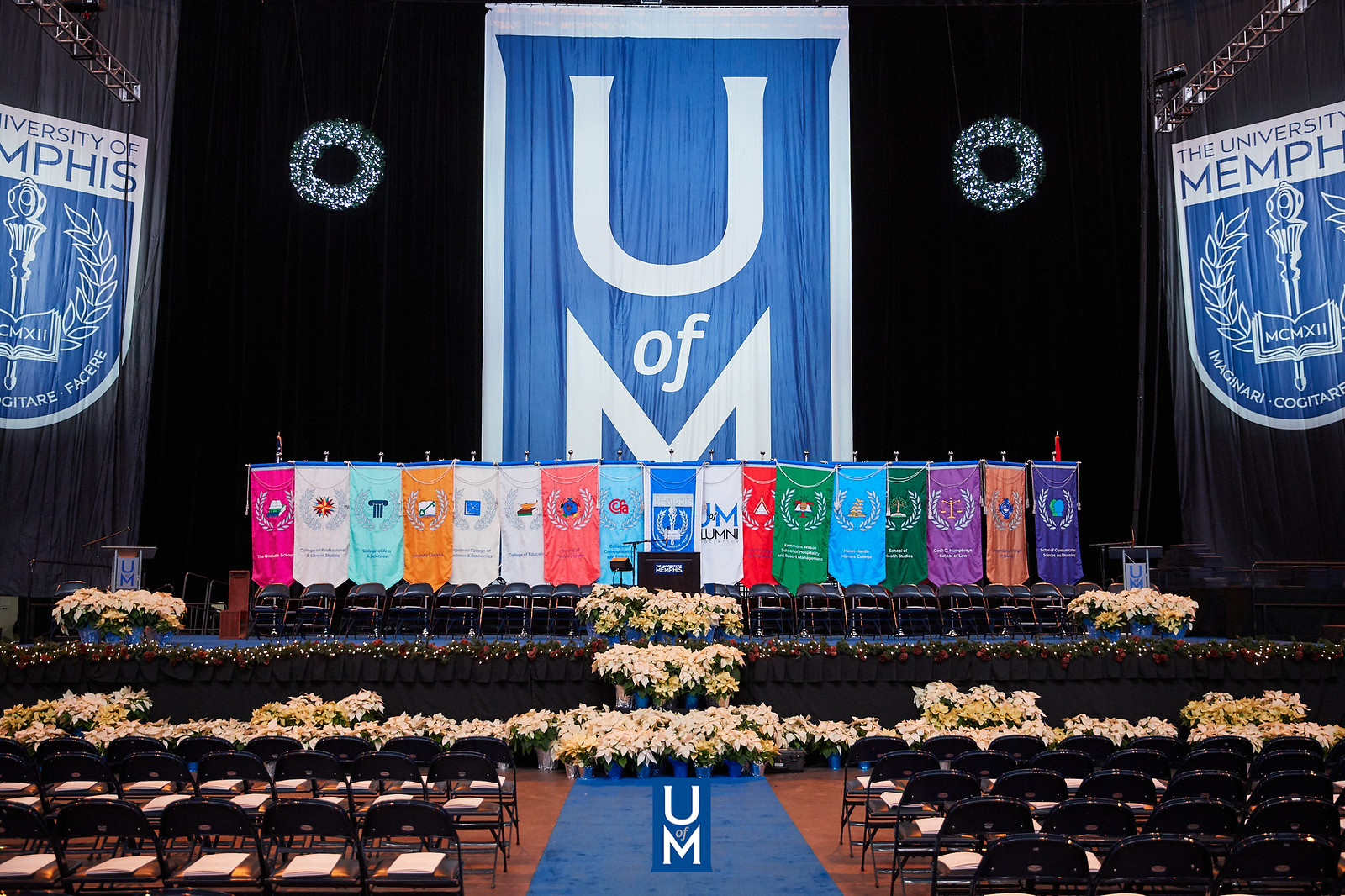
Electronic Theses and Dissertations
Date
2021
Document Type
Dissertation
Degree Name
Doctor of Philosophy
Department
Computer Science
Committee Chair
Santosh Kumar
Committee Member
Dipankar Dasgupta
Committee Member
James M. Rehg
Committee Member
Deepak Venugopal
Abstract
Researchers have developed machine learning models for detecting behaviors (e.g., smoking, eating, and drinking) and health states (e.g., stress) from wearable and mobile sensors. However, these models help detect events after they occur. The next frontier is the prediction of imminent risk of adverse health events.For mobile sensor-based prediction of imminent risk of impulsive behaviors, passive and continuous detection of risk factors of such behaviors is necessary. But, for human thoughts, perceptions, and contexts (e.g., suicidal ideation, craving/urge, desire for immediate gratification, and exposure to risky environmental cues), that are potential antecedents and precipitants of impulsive behaviors (e.g., suicide attempt, overeating, binge-drinking, and smoking lapse), it is challenging to obtain temporally-precise annotations. Furthermore, their manifestations in the underlying mobile sensor data are not yet known. Finally, due to their rapid variability and lack of clear separation of extreme values from well-established population norm, risk estimations of the target impulsive behavior becomes non-trivial.In this dissertation, we propose machine learning methods and models for predicting the imminent risk of impulsive behaviors. Specifically, we propose three key innovations. First, we propose temporal label propagation to address the mismatch between the temporal resolution of labels and the dynamism of the human state and context, determining specific `moments' when they are expected to change. Second, we develop context-specific temporally-precise features to address the lack of known correspondence between the target state and the underlying sensor data in the relevant scientific domains.Finally, we introduce spatio-behavioro-temporal precision features and events-of-interest encodings to represent the person-specific spatio-temporal dynamics of multiple risk factors. We demonstrate the utility of these innovations by developing a risk prediction model for a smoking lapse in newly abstinent smokers.Specifically, we first develop mCrave to estimate the state of cigarette craving during smoking abstinence. Second, we develop SmokingOpp to detect the exposure to environmental or situational cues conducive to a smoking lapse. Finally, we develop mRisk to predict the imminent risk of a smoking lapse. These prediction models can be used to deliver temporally precise sensor-triggered interventions that are both personalized and contextualized to each individual and their current context.
Library Comment
Dissertation or thesis originally submitted to ProQuest
Notes
Embargoed until 4/10/2024
Recommended Citation
Chatterjee, Soujanya, "Machine Learning Models For Predicting the Imminent Risk of Impulsive Behaviors Using mHealth Sensors" (2021). Electronic Theses and Dissertations. 2891.
https://digitalcommons.memphis.edu/etd/2891
Comments
Data is provided by the student.