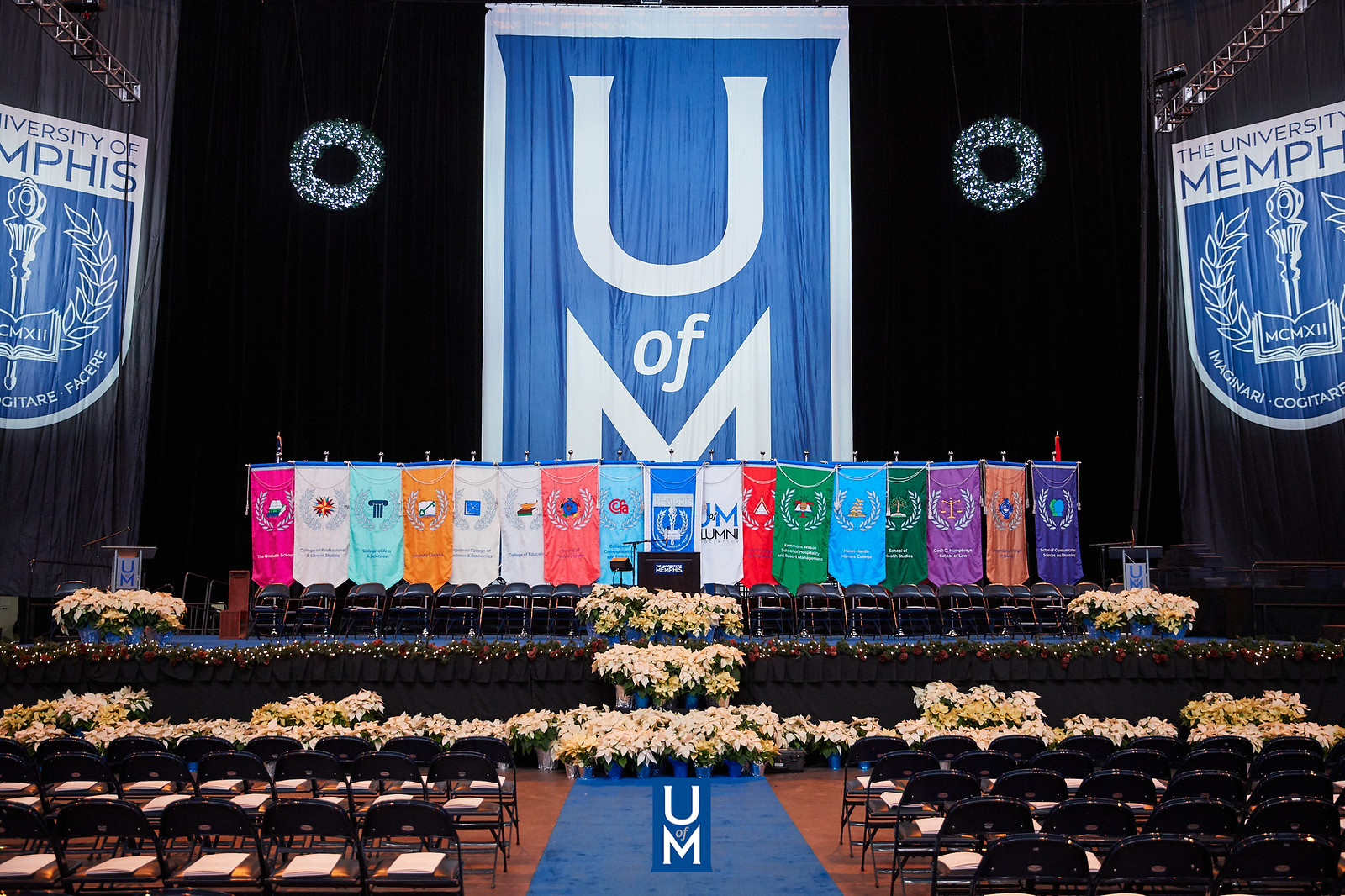
Electronic Theses and Dissertations
Date
2020
Document Type
Dissertation
Degree Name
Doctor of Philosophy
Department
Civil Engineering
Committee Chair
Charles Camp
Committee Member
Shahram Pezeshk
Committee Member
Farzad Naeim
Committee Member
Roger Meier
Abstract
The main objective of the performance-based earthquake engineering (PBEE) framework is to fully understand the scale of the problems due to earthquakes with respect to deaths, dollars, and downtime resulting from the quantification of the risk, which allows prioritization of investment and technical solutions. The key elements of PBEE are seismic hazard analysis, structural analysis, damage analysis, and loss analysis. This dissertation is aimed to implement machine learning, statistical, and probabilistic tools for ground motion (GM) selection that links the seismic hazard, the first element of PBEE, to the structural analysis, the second element of PBEE. The underlying goal is to ensure that the selected ground motions for seismic demand analysis are consistent with the seismic hazard of the considered site. Most of the current structural design and assessment practices consider pseudo-spectral acceleration in GM selection, while the severity of earthquake GMs is a function of amplitude, frequency content, and duration of GMs. This study evaluates the need to explicitly consider different features of earthquakes, in addition to pseudo-spectral acceleration, in structural design and assessment. To this end, this dissertation initially provides the analyst with an understanding regarding the degree to which different aspects of ground motions affect the structural responses. The findings enable the analyst to decide which properties of GMs should be considered in GM selection. The findings reveal that the importance of GM features changes over different earthquake intensity levels implying that different weight vectors are required over different earthquake levels for GM selection. Furthermore, the results demonstrate that, even in the case of shorter-duration GMs from shallow events, GM duration should be incorporated in GM selection as it has effects on the peak-based structural responses over rare earthquake intensity levels. It is found that in case of shallow events, unlike large magnitude earthquakes, the shorter the duration of GM, the more rapid release of energy and, consequently, the larger the peak-based structural responses.One area that remains a topic of debate is that no outcomes can be found concerning the effect of GM duration on structural responses when considering different choices of conditioning intensity measures (IMs). This dissertation examines the role of the conditioning IM in the degree that GM duration influences the structural responses. The results show that the importance of GM duration mainly depends on the considered conditioning IM. Specifically, GM duration does not substantially affect the structural responses in terms of probability of collapse if peak ground acceleration, peak ground velocity, spectrum intensity, spectral acceleration at higher modes are implemented as the conditioning IMs. On the other hand, in the case of cumulative absolute velocity and spectral acceleration at the fundamental and lengthened periods, the structural responses are considerably affected by GM duration. Moreover, it is found that the induced bias in risk-based structural responses due to neglecting GM duration could vary up to about 40% over the rare hazard levels depending on the applied conditioning IM.This dissertation also investigates the number of real GMs required to accurately capture the structural responses when using code-, intensity-, and risk-based seismic assessment frameworks. A key consideration is the use of as-recorded hazard consistent GMs, which appropriately characterize the seismic hazard of the site, for performing response history analysis. The empirical results demonstrate that a risk-based assessment needs a larger number of response history analyses than an intensity-based assessment method to capture the seismic demand on the structural systems accurately. Also, given the same number of GMs, the structural responses from a risk-based assessment are more reliable and stable than those for an intensity-based method. Also, the results indicate that a significant bias in structural responses is likely when following the recommendations from several seismic design codes, which suggest utilizing the maximum structural responses if 3 GM pairs are used for response history analysis. Detailed statistical analyses show that the application of 7 GM pairs is sufficient to accurately and reliably estimate structural responses.Deriving the fragility curves is a key step in seismic risk assessment within the PBEE framework. The next objective of this dissertation is to implement machine learning tools for predicting the structural responses and fragility curves. The statistical results using the implemented dataset demonstrate that among applied methods, the random forest is preferable since it shows the highest efficiency in predicting the structural responses. Moreover, a detailed procedure is presented on how to derive the fragility curves based on the machine learning tools. Finally, the sensitivity of the applied machine learning methods to the size of the employed dataset is investigated. The results explain that logistic regression, lasso regression, and Nave Bayes are not sensitive to the size of the dataset (i.e., the number of performed time history analyses), while the performance of discriminant analysis significantly depends on the size of the applied dataset.Finally, this dissertation presents a step by step procedure for reducing the need for a large number of response history analyses (RHAs) in developing machine learning models to predict the structural responses. This study uses a pool-based query-by-committee active learning algorithm to choose probably the most informative GM samples for training the machine learning models. The results demonstrate that implementing active learning can considerably reduce the size of required training data and, consequently, the amount of RHAs while improving the performance of the prediction models. Specifically, the findings explain that active learning improves the average F-scores of ANNs by about 10% and significantly reduces their variations, which indicates its stable behavior.
Library Comment
Dissertation or thesis originally submitted to ProQuest
Notes
embargoed
Recommended Citation
Kiani, Jalal, "APPLICATION OF MACHINE LEARNING AND STATISTICAL TOOLS FOR GROUND MOTION SELECTION" (2020). Electronic Theses and Dissertations. 2920.
https://digitalcommons.memphis.edu/etd/2920
Comments
Data is provided by the student.