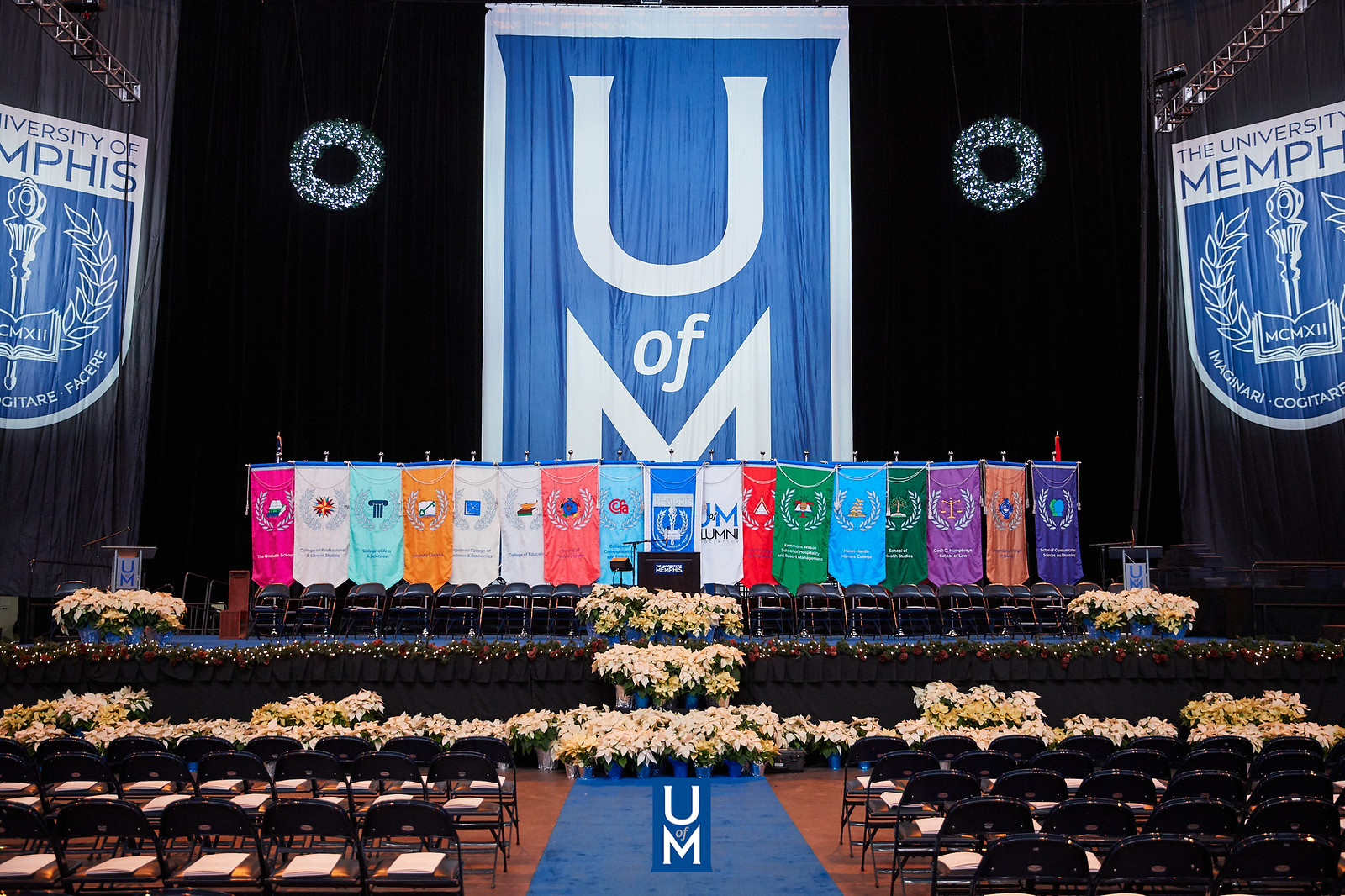
Electronic Theses and Dissertations
Date
2020
Document Type
Dissertation
Degree Name
Doctor of Philosophy
Department
Computer Science
Committee Chair
Santosh Kumar
Committee Member
Paul Balister
Committee Member
Nirman Kumar
Committee Member
Mani Srivastava
Abstract
Wrist-worn inertial sensors in activity trackers and smartwatches are increasingly being used for daily tracking of activity and sleep. Wearable devices, with their onboard sensors, provide appealing mobile health (mHealth) platform that can be leveraged for continuous and unobtrusive monitoring of an individual in their daily life. As a result, an adaptation of wrist-worn devices in many applications (such as health, sport, and recreation) increases. Additionally, an increasing number of sensory datasets consisting of motion sensor data from wrist-worn devices are becoming publicly available for research. However, releasing or sharing these wearable sensor data creates serious privacy concerns of the user. First, in many application domains (such as mHealth, insurance, and health provider), user identity is an integral part of the shared data. In such settings, instead of identity privacy preservation, the focus is more on the behavioral privacy problem that is the disclosure of sensitive behaviors from the shared sensor data. Second, different datasets usually focus on only a select subset of these behaviors. But, in the event that users can be re-identified from accelerometry data, different databases of motion data (contributed by the same user) can be linked, resulting in the revelation of sensitive behaviors or health diagnoses of a user that was neither originally declared by a data collector nor consented by the user. The contributions of this dissertation are multifold. First, to show the behavioral privacy risk in sharing the raw sensor, this dissertation presents a detailed case study of detecting cigarette smoking in the field. It proposes a new machine learning model, called puffMarker, that achieves a false positive rate of 1/6 (or 0.17) per day, with a recall rate of 87.5%, when tested in a field study with 61 newly abstinent daily smokers. Second, it proposes a model-based data substitution mechanism, namely mSieve, to protect behavioral privacy. It evaluates the efficacy of the scheme using 660 hours of raw sensor data collected and demonstrates that it is possible to retain meaningful utility, in terms of inference accuracy (90%), while simultaneously preserving the privacy of sensitive behaviors. Finally, it analyzes the risks of user re-identification from wrist-worn sensor data, even after applying mSieve for protecting behavioral privacy. It presents a deep learning architecture that can identify unique micro-movement pattern in each wearer's wrists. A new consistency-distinction loss function is proposed to train the deep learning model for open set learning so as to maximize re-identification consistency for known users and amplify distinction with any unknown user. In 10 weeks of daily sensor wearing by 353 participants, we show that a known user can be re-identified with a 99.7% true matching rate while keeping the false acceptance rate to 0.1% for an unknown user. Finally, for mitigation, we show that injecting even a low level of Laplace noise in the data stream can limit the re-identification risk. This dissertation creates new research opportunities on understanding and mitigating risks and ethical challenges associated with behavioral privacy.
Library Comment
Dissertation or thesis originally submitted to ProQuest
Notes
embargoed
Recommended Citation
Saleheen, Nazir, "Behavioral Privacy Risks and Mitigation Approaches in Sharing of Wearable Inertial Sensor Data" (2020). Electronic Theses and Dissertations. 2953.
https://digitalcommons.memphis.edu/etd/2953
Comments
Data is provided by the student.