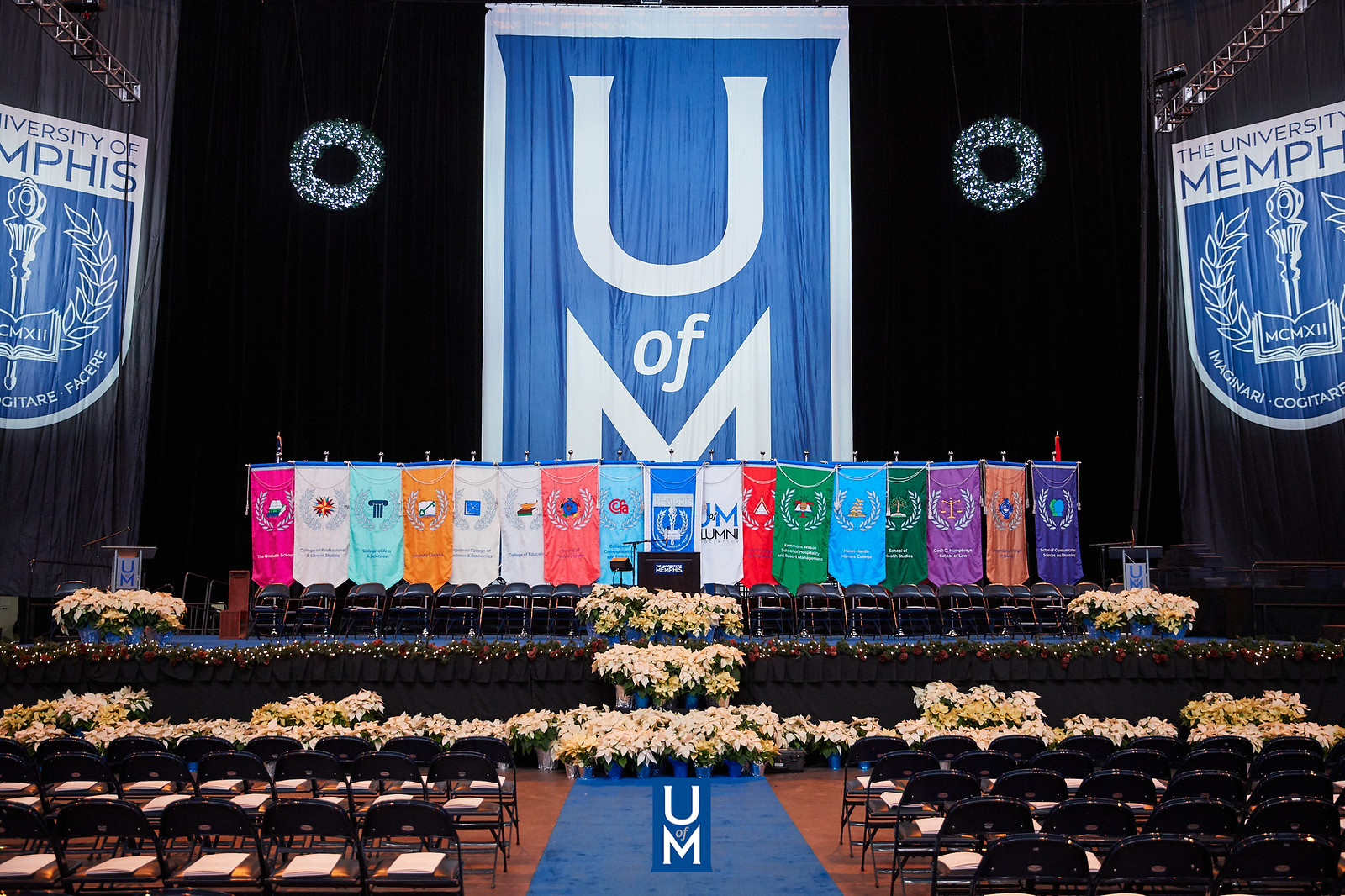
Electronic Theses and Dissertations
Date
2021
Document Type
Dissertation
Degree Name
Doctor of Philosophy
Department
Electrical & Computer Engineering
Committee Chair
Madhusudhanan Balasubramanian
Committee Member
Eddie Jacobs
Committee Member
Aaryani Tipirneni-Sajja
Committee Member
Deepak Venugopal
Abstract
Transformation of any generic scene including structural deformation and fluid flow can be estimated non-invasively from an image sequence using optical flow estimation techniques. Dense optical flow estimation is more challenging when there are large displacements in a scene with heterogeneous motion dynamics, occlusion, and scene homogeneity. Recently developed deep learning methods show significant promise in estimating optical flow with high accuracy. Majority of these methods, however, have been more commonly derived from architectures of related computer vision tasks such as semantic segmentation and require a large number of training parameters. Accuracy of these methods is also affected by the ghosting artifacts when images are warped across multiple resolutions and by the vanishing problem in regions with higher motion contrast. In this work, we present three key strategies for building a compact dense prediction network using standard network elements primarily guided by the effective receptive field (ERF) of the network. The design strategies are: 1) to preserve spatial information throughout the network, 2) to facilitate a large enough ERF for the output neurons, and 3) to cascade sub-networks with varying ERF spatial extent, shape, and smoothness characteristics to handle heterogeneous flow magnitudes and motion dynamics. We constructed deep dilated convolutional neural networks (DDCNets) based on these strategies and validated their performance using standard benchmark computer vision datasets. Our experiments demonstrate that DDCNets are compact with significantly fewer trainable parameters and provide optical flow estimates with accuracy comparable to similar lightweight learning-based methods. Further, we demonstrate an application of these learning-based optical flow methods in developing candidate biomarkers of glaucoma and its progression. The diagnostic accuracy of the biomarkers was validated using longitudinal confocal microscopy exams of 12 non-human primate eyes under controlled experimental conditions and of a cohort of 57 clinical subjects. DDCNets trained to estimate generic deformation were able to detect ONH deformation from confocal images and provided a higher diagnostic accuracy when compared to the legacy biomarkers of glaucoma progression.
Library Comment
Dissertation or thesis originally submitted to ProQuest
Notes
Embargoed until 4/4/2024
Recommended Citation
Salehi, Ali, "LEARNING MOTION ESTIMATION" (2021). Electronic Theses and Dissertations. 2954.
https://digitalcommons.memphis.edu/etd/2954
Comments
Data is provided by the student.