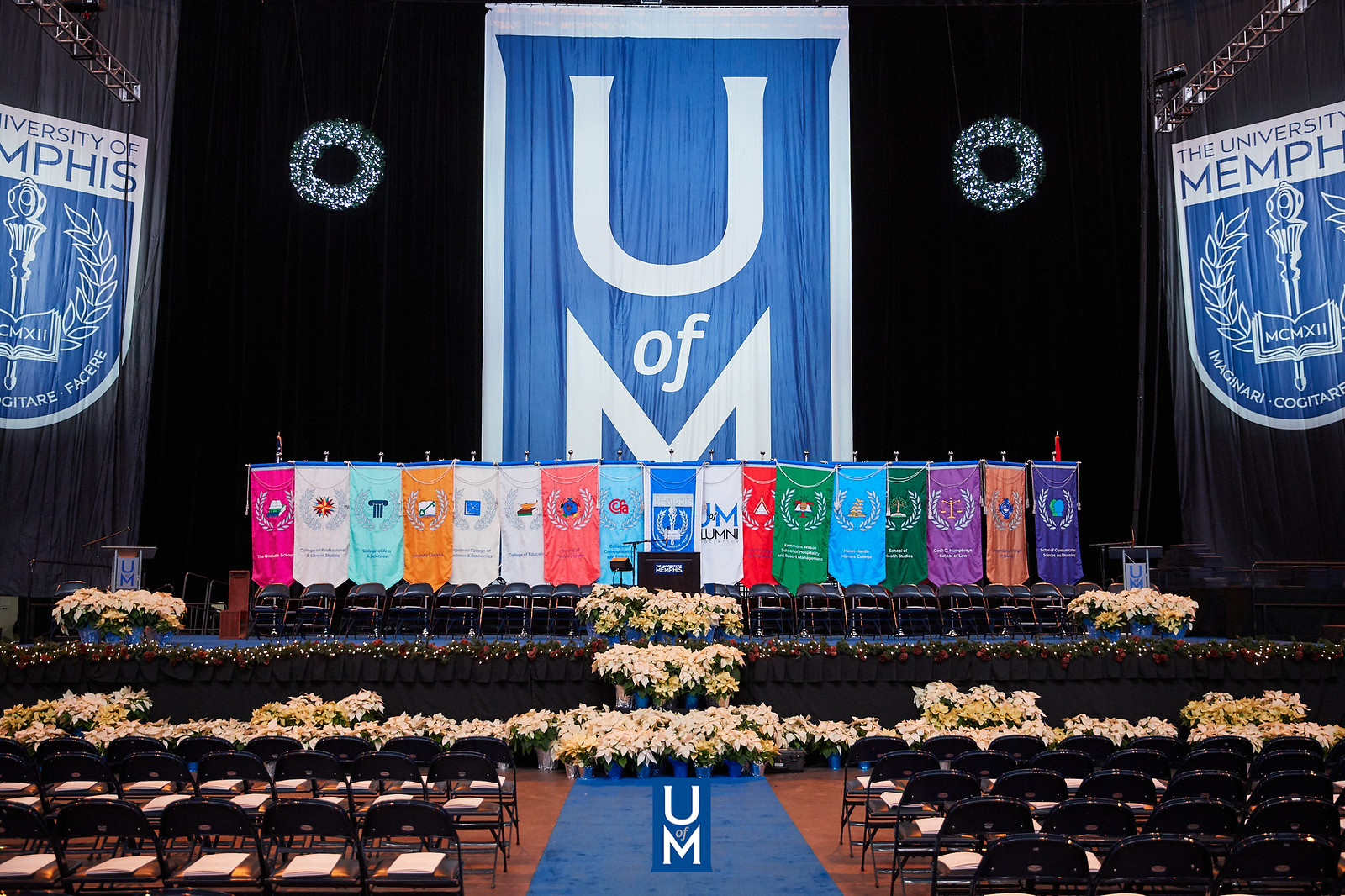
Electronic Theses and Dissertations
Date
2023
Document Type
Thesis
Degree Name
Master of Science
Department
Electrical & Computer Engineering
Committee Chair
Chrysanthe Preza
Committee Member
Aaron AR Robinson
Committee Member
Deepak DV Venugopal
Abstract
In three-dimensional structured illumination microscopy (3D-SIM) where the images are taken from the object through the point spread function (PSF) of the imaging system, data acquisition can result in images taken under undesirable aberrations that contribute to a model mismatch. The inverse imaging problem in 3D-SIM has been solved using a variety of conventional model-based techniques that can be computationally intensive. Deep learning (DL) approaches, as opposed to traditional restoration methods, tackle the issue without access to the analytical model. This research aims to provide an unrolled physics-informed generative adversarial network (UPIGAN) for the reconstruction of 3D-SIM images utilizing data samples of mitochondria and lysosomes obtained from a 3D-SIM system. This design makes use of the benefits of physics knowledge in the unrolling step. Moreover, the GAN employs a Residual Channel Attention super-resolution deep neural network (DNN) in its generator architecture. The results indicate that the addition of both physics-informed unrolling and GAN incorporation yield improvements in reconstructed results compared to the regular DL approach.
Library Comment
Dissertation or thesis originally submitted to ProQuest
Notes
Open Access
Recommended Citation
Dajkhosh, Seyedeh Parisa, "STRUCTURED ILLUMINATION MICROSCOPE IMAGE RECONSTRUCTION USING UNROLLED PHYSICS-INFORMED GENERATIVE ADVERSARIAL NETWORK (UPIGAN)" (2023). Electronic Theses and Dissertations. 2992.
https://digitalcommons.memphis.edu/etd/2992
Comments
Data is provided by the student.