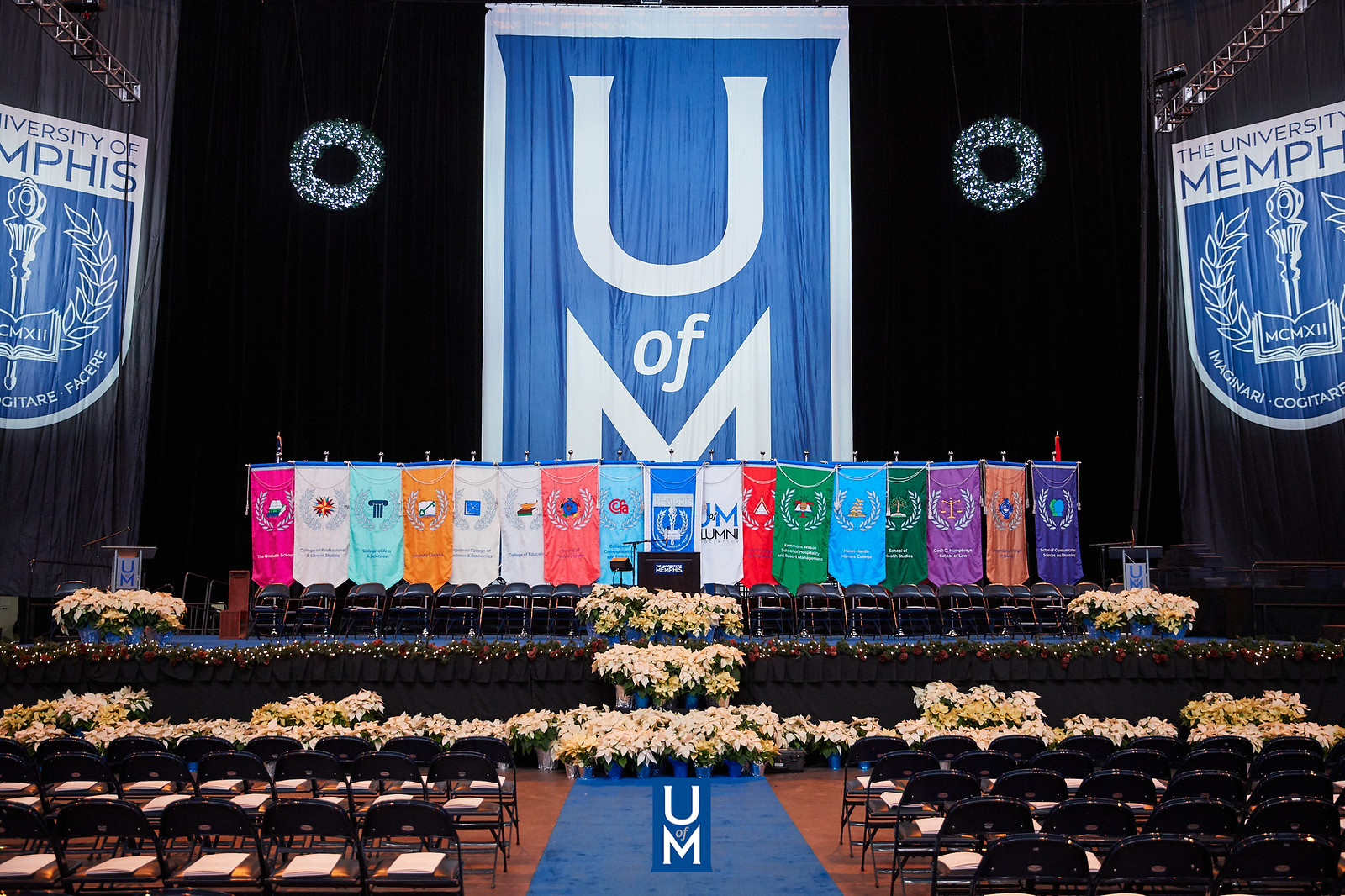
Electronic Theses and Dissertations
Date
2023
Document Type
Thesis
Degree Name
Master of Science
Department
Electrical & Computer Engineering
Committee Chair
Dr. Mohammed Yeasin
Committee Member
Dr. Faruk Ahmed
Committee Member
Dr. Vida Abedi
Committee Member
Dr. Aaron L. Robinson
Abstract
Performances of Deep Learning (DL) based methods for 3D reconstruction are becoming at par or better than classical computer vision techniques. Learning requires data with proper annotations. While images have a standardized representation, there is currently no widely accepted format for efficiently representing 3D output shapes. The challenge lies in finding a format that can handle the high-resolution geometry of any shape while also being memory and computationally efficient. Therefore, most advanced learning-based 3D reconstructions are restricted to a certain domain. In this work, we compare the performance of different output representations for 3D reconstruction in different contexts including objects or natural scenes, full human body to human body parts reconstruction. Despite substantial progress in the semantic understanding of the visual world, there are few methods that can reconstruct from a single view for a s large set of objects. Our the objective is to investigate methods to reconstruct a wider variety of object categories in 3D and aim to achieve accurate 3D reconstruction at both object and scene levels. In this work, we compare the performance of different output representations for 3D reconstruction in such a way that will give us implicit and smooth output representation of complex geometry of 3D from RGB images, DICOM (Digital Imaging and Communications in Medicine) formatted MRI breast images and images from a wild environment in terms of input using the Deep Learning methods and available 3D processing applications (MeshLab, 3D Slicer, and Mayavi).
Library Comment
Dissertation or thesis originally submitted to ProQuest
Notes
Open Access
Recommended Citation
Ahmed, Md Kaisar, "Measurement and Evaluation of Deep Learning Based 3D Reconstruction" (2023). Electronic Theses and Dissertations. 2993.
https://digitalcommons.memphis.edu/etd/2993
Comments
Data is provided by the student.