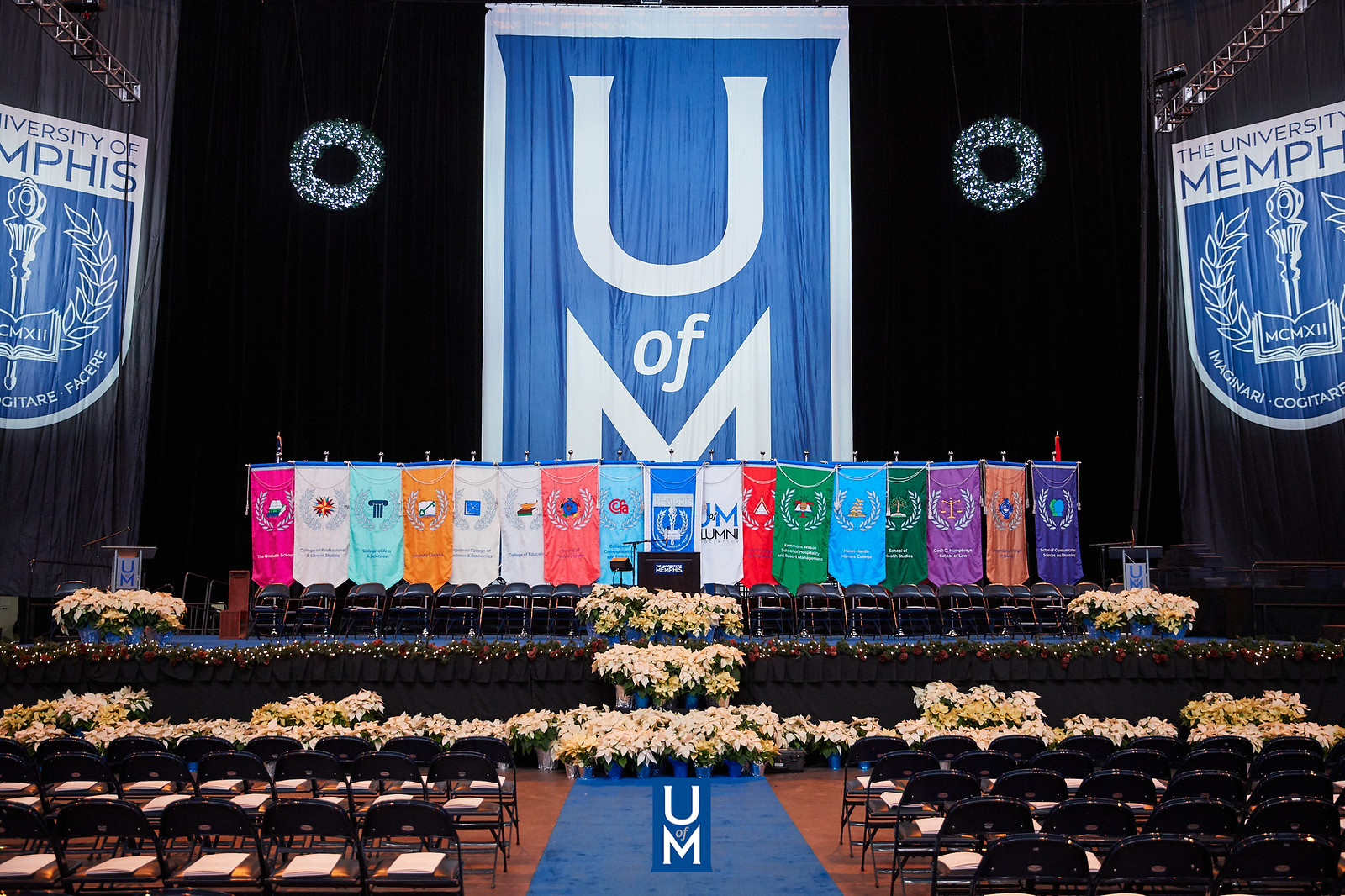
Electronic Theses and Dissertations
Date
2023
Document Type
Thesis
Degree Name
Master of Science
Department
Public Health
Committee Chair
Yu Jiang
Committee Member
Hongmei Zhang
Committee Member
Chunrong Jia
Abstract
Abstract As environmental data grows in complexity, machine learning presents an avenue to extract meaningful insights from such data. This study aimed to investigate the applicability and performance of various machine learning methods for multi-class classification problems, with a specific focus on complex environmental data, including Polycyclic Aromatic Hydrocarbons (PAHs). In the current study, we evaluated ten machine learning models to assess their performance in multivariate classification problems using simulation studies. The results showed that Regularized Multinomial Logistic Regression (RMLR) has higher classification accuracy when the independent variables are independent, while the Gradient Boosting Machine (GBM) outperformed others when the independent variables are highly correlated. Furthermore, the feature selection accuracy of three different methods was also evaluated. GBM and Random Forest (RF) showed a higher sensitivity compared to other methods across different data settings. Based on these findings, it appears that linear models such as RMLR and MLR may not achieve optimal performance when confronted with highly correlated independent variables. Instead, tree-based methods, such as GBM and RF, prove to be a better choice. Overall, it is crucial to choose the appropriate machine learning methods based on the complexity of environmental data and the specific requirements of the task.
Library Comment
Dissertation or thesis originally submitted to ProQuest
Notes
Open Access
Recommended Citation
Fu, Xianqiang, "Evaluation of Machine Learning Methods for Multivariate Classification with Application to Environmental Datasets" (2023). Electronic Theses and Dissertations. 3012.
https://digitalcommons.memphis.edu/etd/3012
Comments
Data is provided by the student.