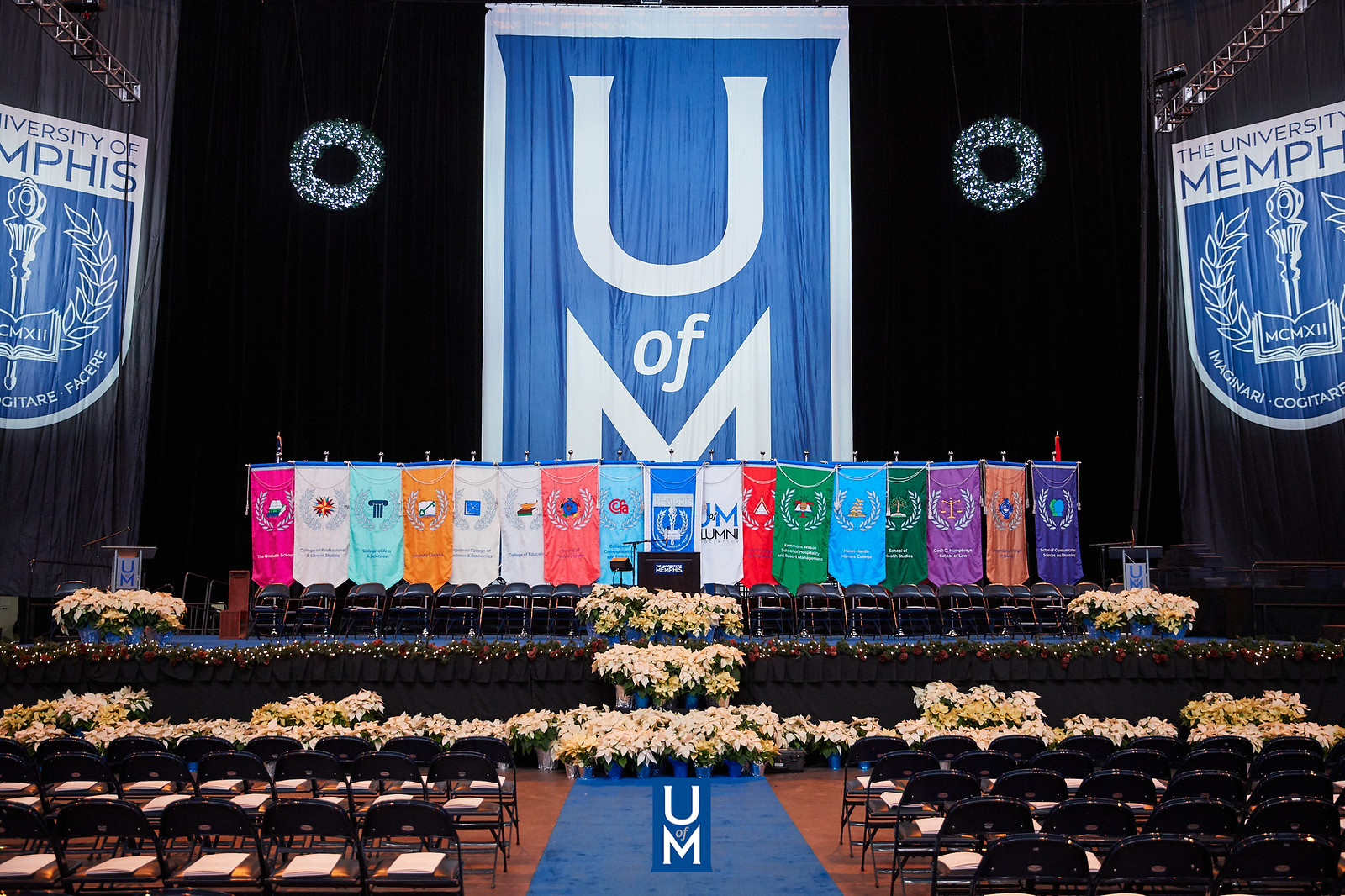
Electronic Theses and Dissertations
Identifier
389
Date
2011
Document Type
Dissertation
Degree Name
Doctor of Philosophy
Major
Electrical and Computer Engr
Concentration
Computer Engineering
Committee Chair
Khan M. Iftekharuddin
Committee Member
Pinakin G. Davey
Committee Member
Aaron L. Robinson
Committee Member
Ebenezer O. George
Abstract
Glaucoma is a leading cause of blindness worldwide. Since glaucomatous vision loss is irreversible, early detection is essential to prevent or manage this disease. Assessing retinal nerve fiber layer (RNFL) using imaging technologies plays an important role in early glaucoma detection. However, accurate evaluation of the RNFL using such technologies can be limited due to overlaps. In literature, feature-based techniques such as fast-Fourier analysis and wavelet-Fourier analysis have shown better diagnostic capability over the standard methods. Nevertheless, such techniques may not fully represent local variation and randomness in the RNFL.Similarly, progression prediction is also critical. Assessing visual field (VF) data using standard automated perimetry (SAP) has been widely used in diagnosing glaucomatous progression. However, use of VF data is functional and subjective and VF data analysis may not fully indicate true progression due to short-term and long-term fluctuation. Hence, diagnosis of progression in an objective and quantitative manner still remains challenging. For progression prediction, it is necessary to differentiate the patients according to the severity of progression. Consequently, progression prediction at different disease stages may require classifiers involving more than two classes.The primary contribution of this dissertation is investigation of feature-based techniques that exploit novel fractal features. These fractal features have shown better characterization of local variation and randomness in structural changes in the RNFL. Consequently, our novel fractal features offer improved glaucoma detection and progression prediction capability than the standard methods and other feature-based techniques. A novel multi-class classification is further proposed for enhanced progression prediction.Glaucoma is known to be characterized by both structural and functional changes. For a complete assessment of glaucoma, it is therefore essential to consider both structural and functional changes. In this work, we exploit a known topographic correspondence between structural and functional data for selective fusion of respective features from both data for improved glaucoma detection.Statistical analyses show that our novel fractal features, multi-class classification and selective fusion of structural and functional data have better diagnostic and predictive capability of glaucoma and glaucomatous progression, when compared with the existing standard and feature-based techniques.
Library Comment
Dissertation or thesis originally submitted to the local University of Memphis Electronic Theses & dissertation (ETD) Repository.
Recommended Citation
Kim, Young June June, "Novel Feature-Based Methods for Improved Glaucoma Detection and Progression Prediction" (2011). Electronic Theses and Dissertations. 302.
https://digitalcommons.memphis.edu/etd/302
Comments
Data is provided by the student.