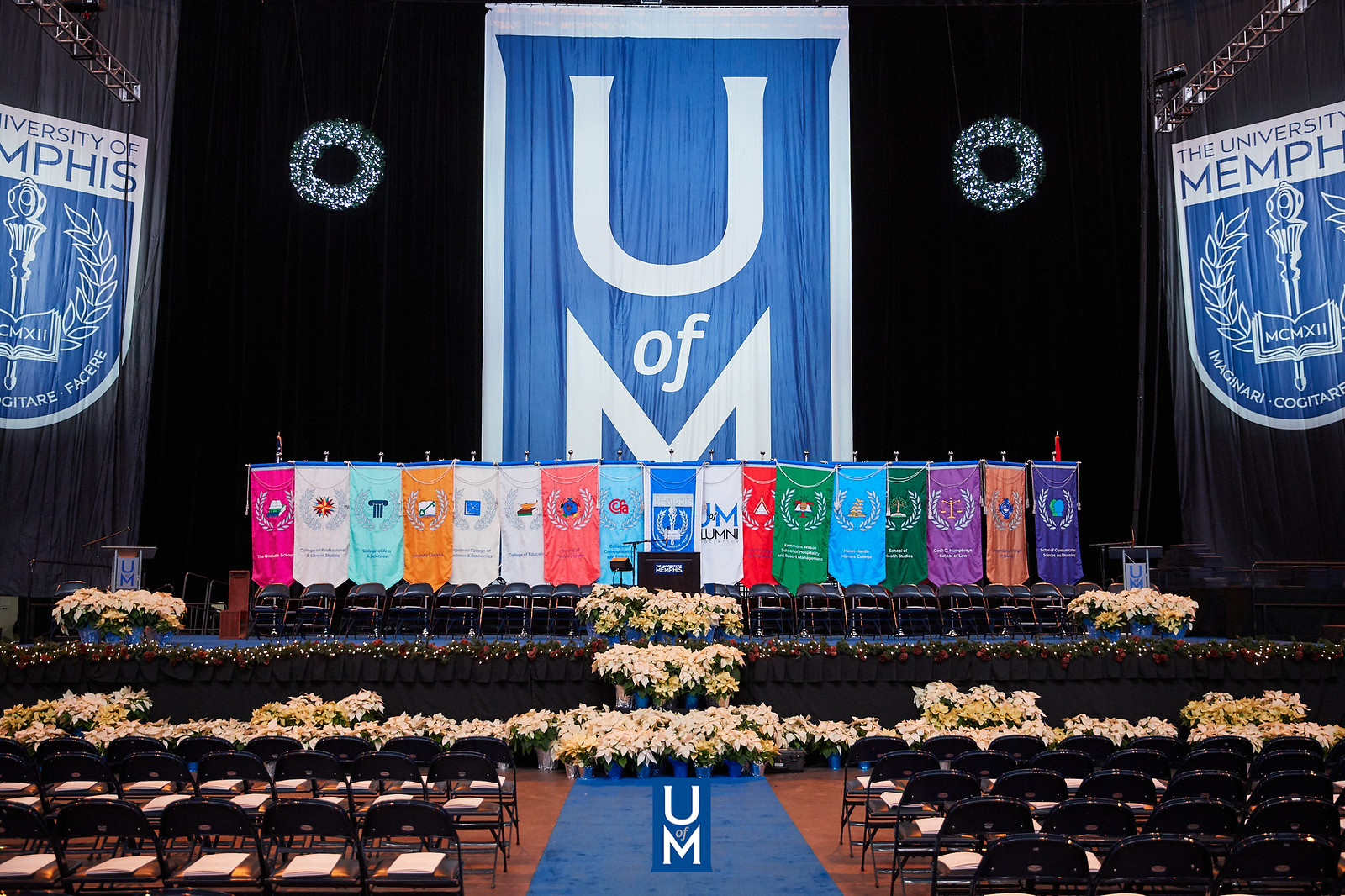
Electronic Theses and Dissertations
Date
2023
Document Type
Thesis
Degree Name
Master of Science
Department
Electrical & Computer Engineering
Committee Chair
Ana Doblas
Committee Member
Madhusudhanan Balasubramanian
Committee Member
Omar Skalli
Abstract
Confocal microscopy is a widely used tool that provides valuable morphological and functional information within cells and tissues. A major advantage of confocal microscopy is its ability to record multi-color and optically sectioned images. A major drawback to confocal microscopy is its diffraction-limited spatial resolution. Though techniques have been developed that break this limit in confocal microscopy, they require additional hardware or accurate estimates of the system’s impulse response (e.g., point spread function). Here we investigate two deep learning-based models, the cGAN and cycleGAN, trained with low-resolution (LR) and high-resolution (HR) confocal images to improve spatial resolution in confocal microscopy. Our findings conclude that the cGAN can accurately produce HR images if the training set contains images with a high signal-to-noise ratio. We have also found that the cycleGAN model has the potential to perform as the cGAN model but without the requirement of using paired inputs.
Library Comment
Dissertation or thesis originally submitted to ProQuest
Notes
Open Access
Recommended Citation
Patra, Shashwat, "EXPLORING DEEP LEARNING METHODS FOR LOW NUMERICAL APERTURE TO HIGH NUMERICAL APERTURE RESOLUTION ENHANCEMENT IN CONFOCAL MICROSCOPY" (2023). Electronic Theses and Dissertations. 3092.
https://digitalcommons.memphis.edu/etd/3092
Comments
Data is provided by the student.