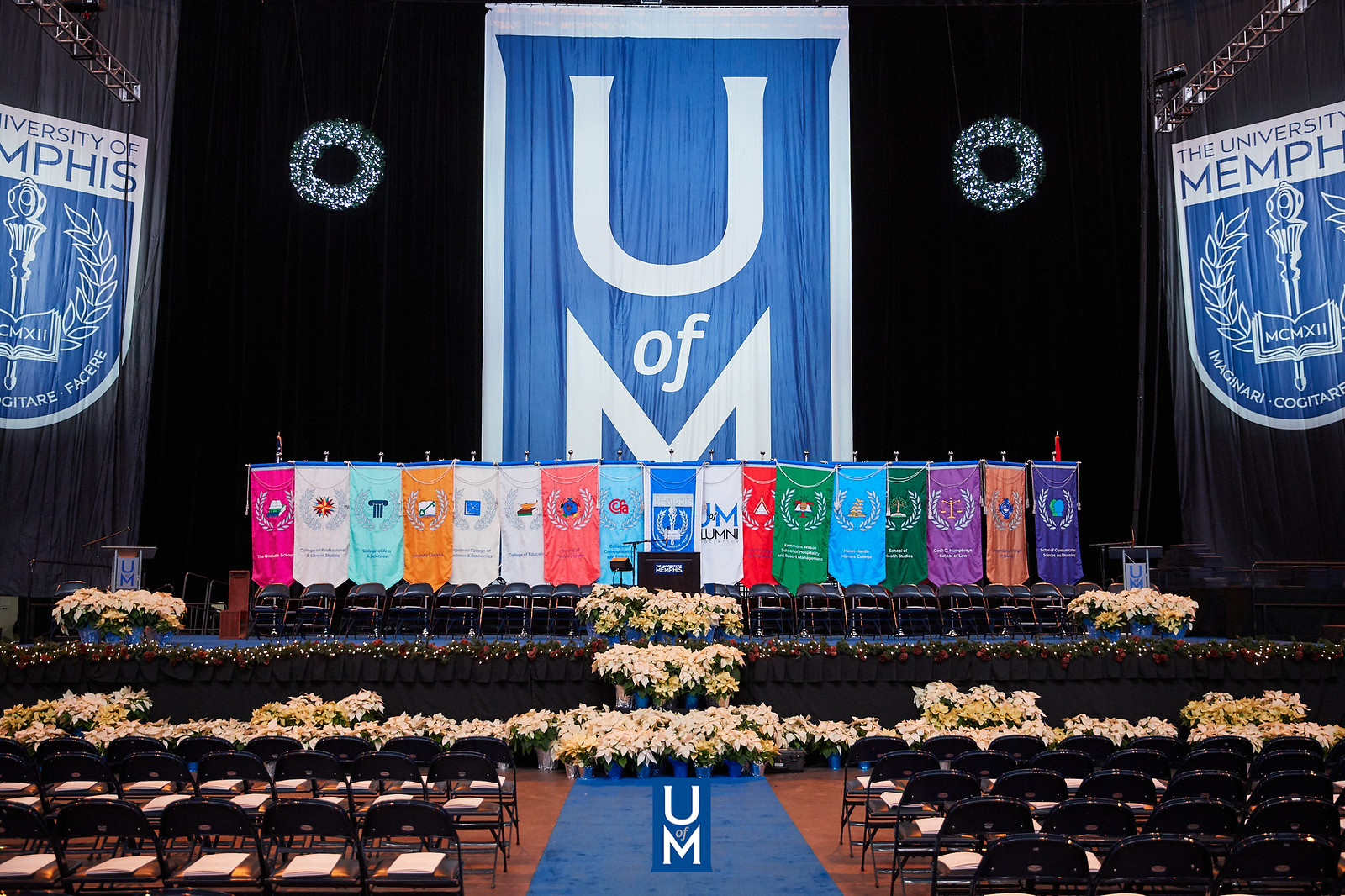
Electronic Theses and Dissertations
Date
2023
Document Type
Thesis
Degree Name
Master of Science
Department
Public Health
Committee Chair
Meredith Ray
Committee Member
Hongmei Zhang
Committee Member
Ching-Chi Yang
Abstract
Partitioning of data into clusters is a widely popular method of gaining insight into the similarities and differences of groups. Amongst the most popular approaches are the K-means and K-prototype methods, however, they fail to consider potential joint effects and interactions of the variables. The Vector in Partition (VIP) algorithm fills this gap with a distance measure designed to partition genetic and epigenetic data; specifically gene expression (GE), DNA methylation (CPG), and single nucleotide polymorphisms (SNP). This work focuses on an extension to the VIP method by furthering incorporating K-means and K-prototype framework within the novel distance measure to incorporate covariate data. This extension allows for another layer of combining complex joint effects of genetic/epi-genetic data and other health-related data to dictate clustering. The results from simulated data showed high accuracy, sensitivity, and specificity of cluster assignments across varying criteria and outperformance of the original VIP method.
Library Comment
Dissertation or thesis originally submitted to ProQuest
Notes
Open Access
Recommended Citation
Handwerker, Joseph, "Vector in Partition extension: Analysis of clustering when genetics distance is weighted by covariates." (2023). Electronic Theses and Dissertations. 3106.
https://digitalcommons.memphis.edu/etd/3106
Comments
Data is provided by the student.