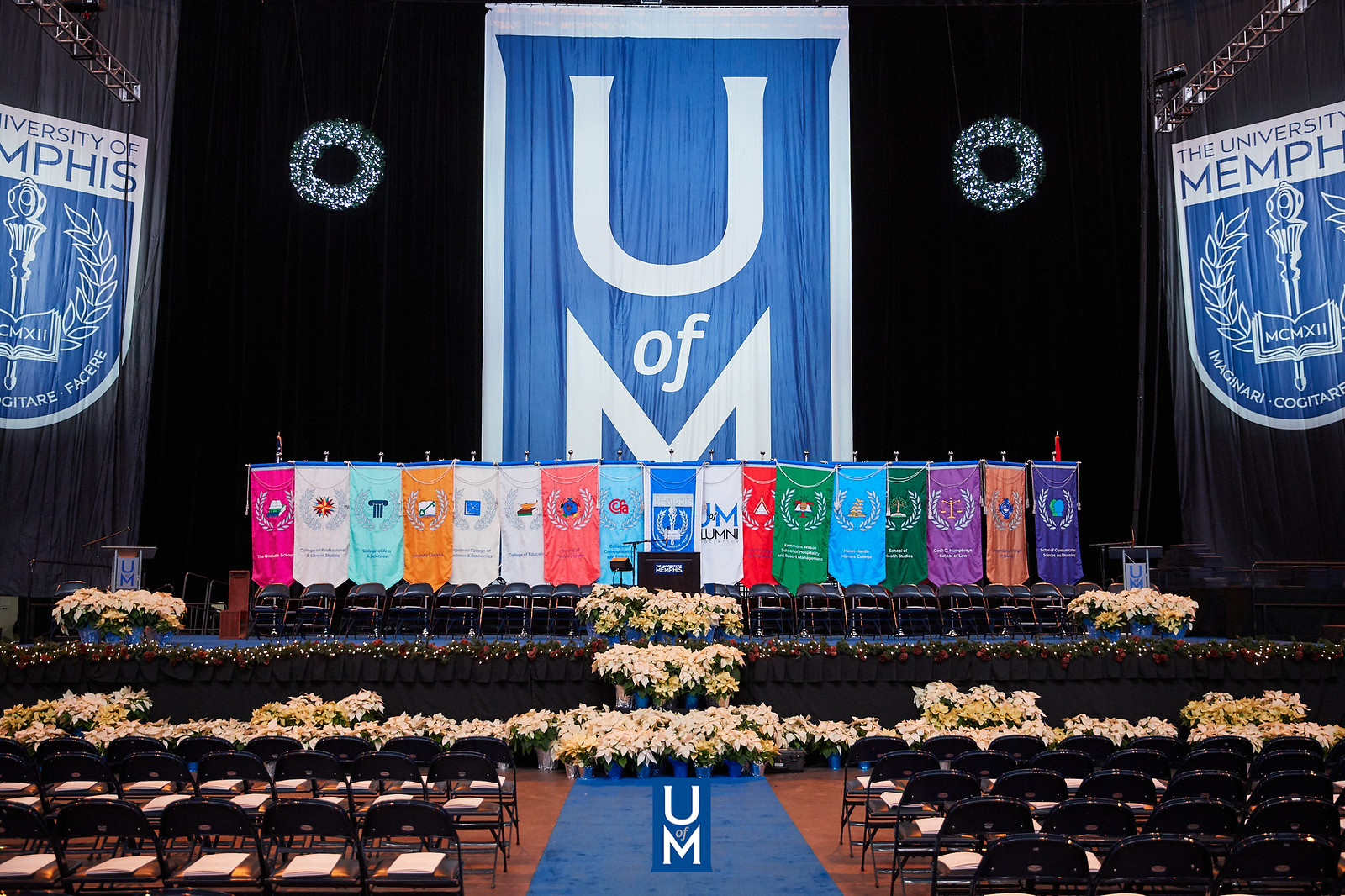
Electronic Theses and Dissertations
Date
2023
Document Type
Thesis
Degree Name
Master of Science
Department
Computer Science
Committee Chair
Xiaolei Wang
Committee Member
Xiaofei XZ Zhang
Committee Member
Deepak DV Venugopal
Abstract
This research addresses the challenges of class imbalance in unsupervised domain adaptation (UDA) and rating prediction in recommender systems. In the context of UDA, the class imbalance between source and target domains presents a significant hurdle for existing models, which tend to focus on domain-invariant representations and class-balanced data. We introduce an unsupervised reinforcement adaptation model (URAM), leveraging reinforcement learning to jointly consider feature variants and imbalanced labels across domains. In recommender systems, the issue of rating imbalance naturally arises, leading to biased predictions and suboptimal performance for tail ratings. Existing rating prediction approaches often rely on weighted cross-entropy and assume a balanced, normal distribution for ratings. In contrast, we present the Gumbel-based Variational Network (GVN) framework, which augments feature representations with Gumbel distributions to address rating imbalance. GVN incorporates a Gumbel-based variational encoder, a multi-scale convolutional fusion network, and a skip connection module for personalized rating predictions. We experiment with the text classification task on URAM and the rating prediction task on GVN to verify their effectiveness in imbalance learning. Experiments on text classification prove that UDA can effectively learn robust domain-invariant representations and successfully adapt text classifiers on imbalanced classes over domains. We evaluate errors- and ranking-based metrics on rating prediction task with GVN. The results prove that the GVN can effectively achieve state-of-the-art performance across the datasets and reduce the biased predictions of tail ratings. We compare with various distributions (e.g., normal and Poisson) and demonstrate the effectiveness of Gumbel-based methods on class-imbalance modeling.
Library Comment
Dissertation or thesis originally submitted to ProQuest.
Notes
Open Access
Recommended Citation
Wu, Yuexin, "Imbalance Learning Representation and Label Shift" (2023). Electronic Theses and Dissertations. 3333.
https://digitalcommons.memphis.edu/etd/3333
Comments
Data is provided by the student