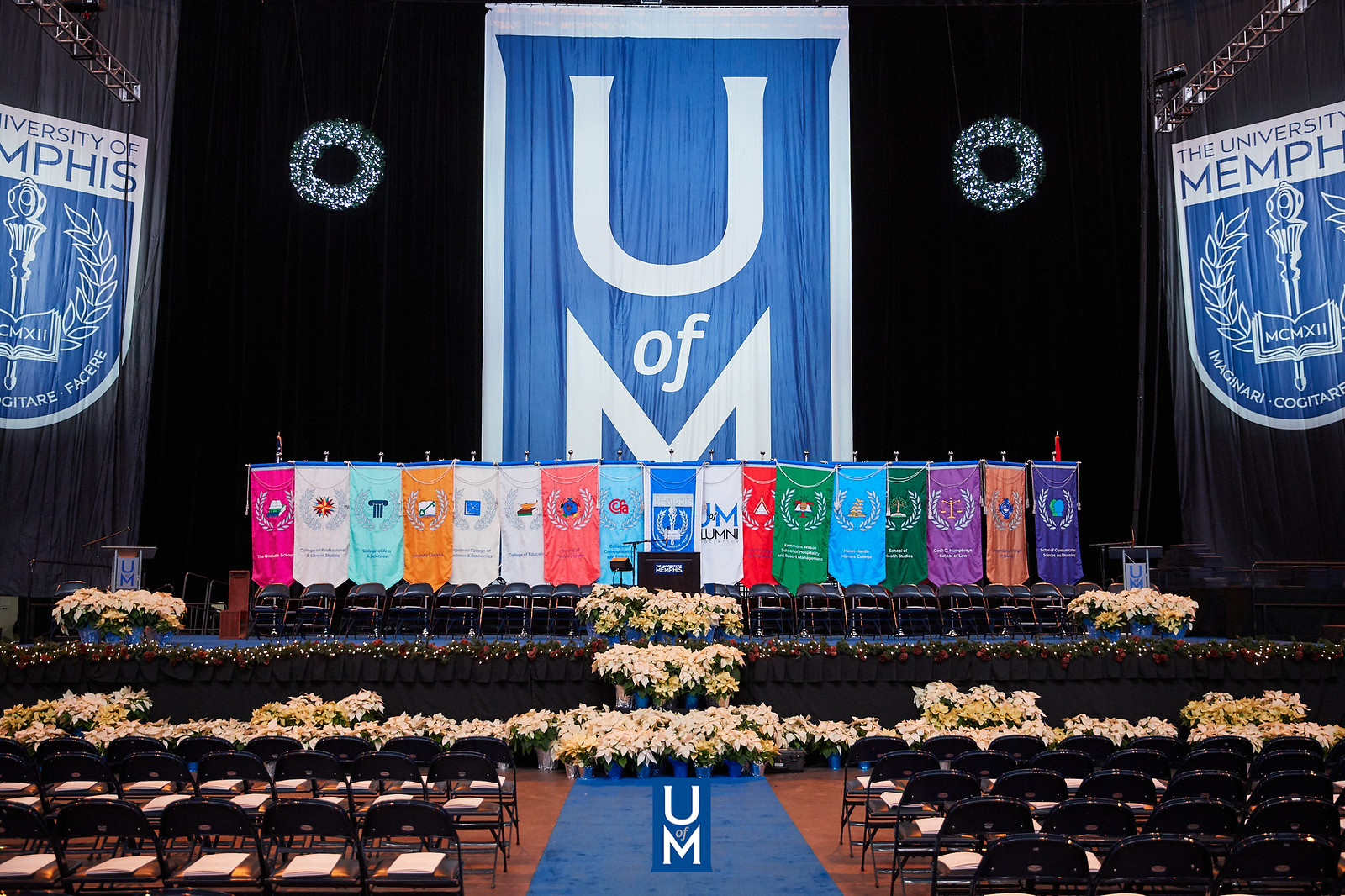
Electronic Theses and Dissertations
Date
2024
Document Type
Dissertation
Degree Name
Doctor of Philosophy
Department
Public Health
Committee Chair
Meredith Ray.
Abstract
Cluster analysis is a popular, well-utilized unsupervised machine learning technique to group individual subjects based on the similarity of their traits. In epidemiological and biomedical research, clustering individuals into groups in order to study their group patterns with respect to outcomes of interest is often useful. Popular clustering methods, including the k-means framework, are well suited to cluster individuals based on continuous, nominal, and mixed type variables. Gower’s similarity is an additional option for clustering based on mixed type data. Clustering of data that has an inherently nested structure poses unique challenges to the classic clustering methodologies, as these solutions do not have the ability to cluster individuals based on vector variables often present in high dimensional data. An example is seen in the problem of clustering individuals based on genetic/epi-genetic data which encompasses single nucleotide polymorphisms (SNPs) information, deoxyribonucleic acid methylation (DNAm) levels information, and level of expression information across multiple genes. At the person level, this data comes together to create a set of multidimensional variables. An appropriate clustering strategy called Vectors in Partitioning has been developed by the research team at the University of Memphis School of Public Health’s Epidemiology, Biostatistics, and Environmental Health division. This novel clustering strategy calculates a distance measure at the gene level, considering multiple input variables of mixed type, nested within the gene, which are summed and compared at the person level. A similar challenge is posed to the classic clustering methods by data containing grouped variables which, together, measure latent constructs. For example, epidemiological data is often structured such that various latent constructs are measures as the combination of multiple variables, representing factors that are likely to work in combination to predict health or social outcomes of interest. The aim of this dissertation is to develop two novel clustering methods which will account for this type of grouped data structure. To our knowledge, no clustering methods currently exist that account for grouped variable data structure. Our proposed methods are novel non-parametric approaches that will allow for assessment of the influence of data with grouped variable structure on various health outcomes.
Library Comment
Dissertation or thesis originally submitted to ProQuest.
Notes
Embargoed unitl 3/27/2026
Recommended Citation
Plaxco, Allison, "Extensions of Vectors in Partitioning: Analysis of Clustering for Grouped Data" (2024). Electronic Theses and Dissertations. 3459.
https://digitalcommons.memphis.edu/etd/3459
Comments
Data is provided by the student.”