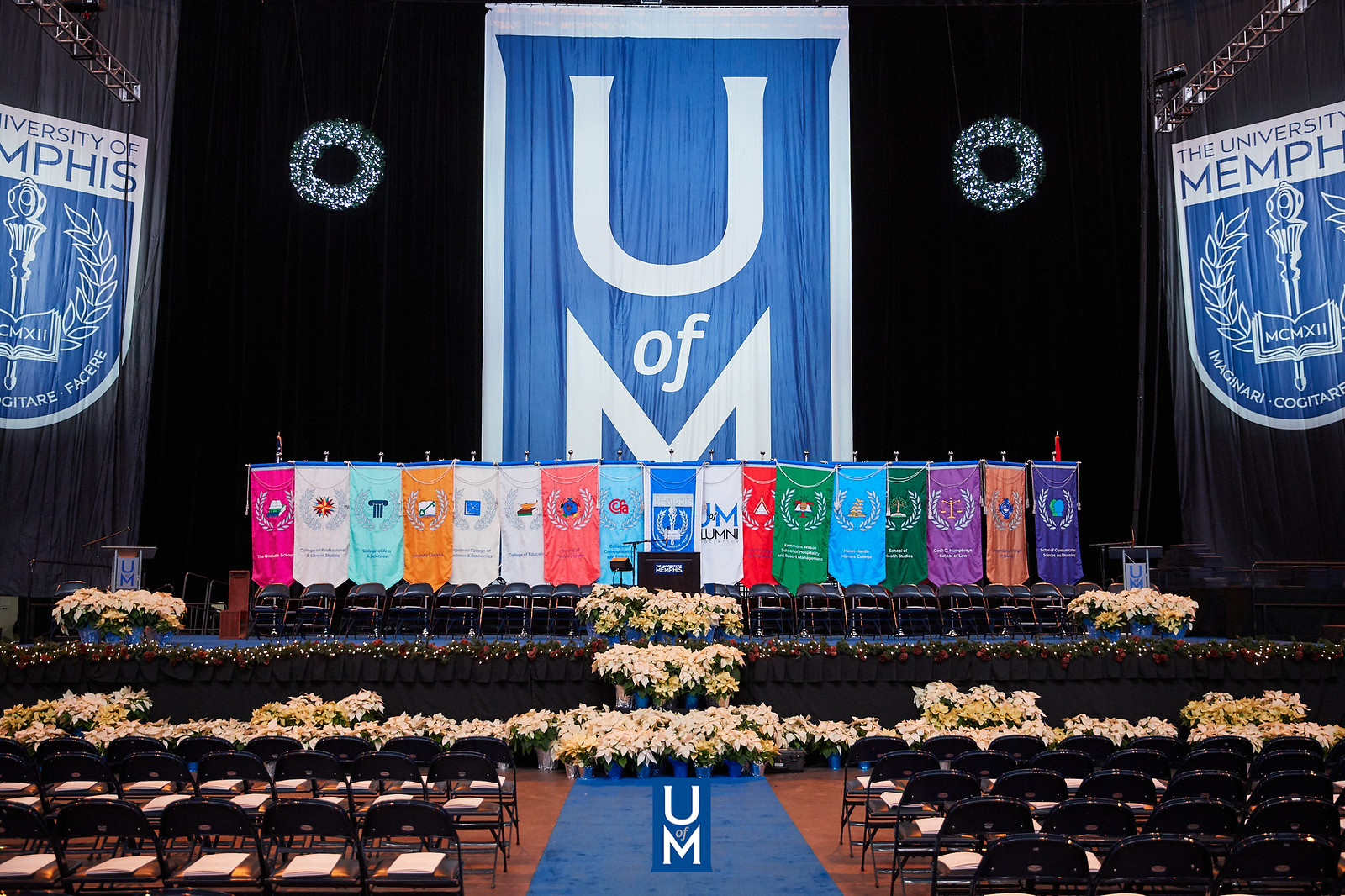
Electronic Theses and Dissertations
Date
2024
Document Type
Dissertation
Degree Name
Doctor of Philosophy
Department
Computer Science
Committee Chair
Myounggyu Won
Committee Member
Lan Wang
Committee Member
Xiaofei Zhang
Committee Member
Sabyasachee Mishra
Abstract
The last mile delivery problem has been one of the most significant challenges in modern logistics and supply chain management. It refers to the final part of the parcel-delivery process, where goods are transported from a distribution center to the individual homes. Frequently, this particular phase becomes the most inefficient and time-consuming segment of the entire delivery process, owing to diverse factors such as urban congestion, limited roadways, and the necessity for multiple stops in a limited area. Among many solutions to address this problem, drone-based last mile delivery is one of the most promising because of the drones’ potential to efficiently navigate through congested urban environments, bypassing obstacles and deliver packages directly to recipients, thus reducing delivery times and operational costs. Integrating drones into last-mile delivery systems can significantly enhance efficiency and reliability, opening new research frontiers in logistics and transportation. The advancement of autonomous vehicles (AVs) is provoking a significant evolution in the conventional drone-assisted last-mile delivery model. The integration of AVs unfolds unprecedented opportunities for complete automation of drone-centric delivery processes. Although in our earlier work we designed a drone-supported delivery system using crowd-sourced AVs for optimal routes, this dissertation proposes an innovative framework addressing three key aspects of integrating AVs into last-mile delivery. First, to further enhance the effectiveness of our proposed system, we consider the dynamically changing traffic conditions impacting the drone-enabled delivery processes. To ensure precise delivery time estimations, we develop a machine learning framework for traffic prediction and integrate it into our drone-based last-mile system. Secondly, we scrutinize the ideal sensor system framework that supports drone-based last-mile deliveries, with special emphasis on energy-efficient communication between roadside sensors and AVs engaged in parcel delivery. Lastly, We analyze the limitations of traditional delivery heuristics and develop a framework integrating Reinforcement Learning to enhance solution quality and computational efficiency in large-scale drone delivery scenarios. Our experimental results demonstrate the potential of machine learning-enhanced approaches to provide robust solutions for last-mile delivery challenges. The methodologies proposed in this dissertation pave the way for advancements in last-mile delivery research, potentially improving logistics and supply chain efficiency.
Library Comment
Dissertation or thesis originally submitted to ProQuest.
Notes
Embargoed until 07-16-2025
Recommended Citation
Imran, Navid Mohammad, "Towards Fully Automated Drone-Based Last-Mile Delivery" (2024). Electronic Theses and Dissertations. 3590.
https://digitalcommons.memphis.edu/etd/3590
Comments
Data is provided by the student.