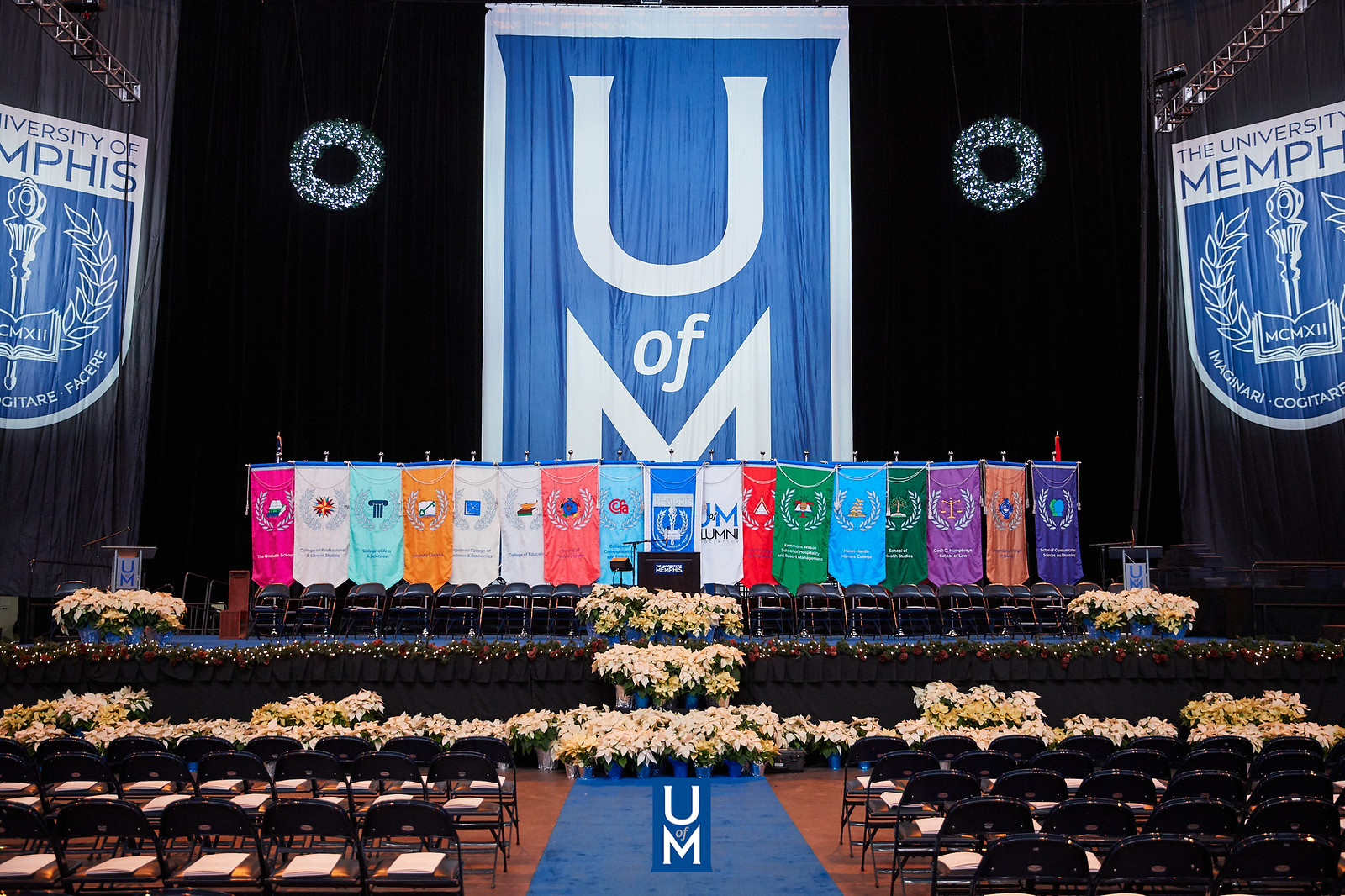
Electronic Theses and Dissertations
Date
2024
Document Type
Dissertation
Degree Name
Doctor of Philosophy
Department
Computer Science
Committee Chair
Myounggyu Won
Committee Member
Kan Yang
Committee Member
Sabyasachee Mishra
Committee Member
Weizi Li
Abstract
Traffic congestion is a serious problem in the USA that affects safety, economy, environments, and human lives. Autonomous vehicles (AVs) equipped with vehicle-to-everything (V2X) communication technology is emerging as a viable solution to mitigate traffic congestion. In this dissertation, we propose an advanced traffic control system for autonomous vehicles, utilizing machine learning techniques, to alleviate traffic congestion, and enhance traffic efficiency and safety. The proposed system consists of two key components: an intelligent adaptive cruise control system (ACC) and a cooperative lane-change system. To address the limitations of existing static model-based approaches, we introduce a novel AI-based ACC system that dynamically adjusts the ACC settings based on real-time traffic conditions. By adapting to changing situations, this system significantly improves traffic efficiency. However, we recognize that current intelligent ACC systems primarily focus on traffic flow enhancement, disregarding the influence of adaptive inter-vehicle gap adjustment on driving safety and comfort. To bridge this gap, we develop a Safety-Aware Intelligent ACC system, which effectively assesses driving safety by dynamically updating safety model parameters according to varying traffic conditions. This innovative approach ensures that driving safety and comfort are prioritized alongside traffic efficiency. Furthermore, we present a novel multi-agent reinforcement learning (MARL)-based intelligent lane-change system for autonomous vehicles. This system optimizes both local and global performance by incorporating a road-side unit (RSU) responsible for managing a specific road segment, as well as vehicle-to-everything (V2X) capabilities for the agents. This density-aware cooperative multi-agent framework enables efficient and safe lane changes, considering the overall traffic conditions and maximizing the benefits for all vehicles involved. Finally, we present a use case scenario of our proposed next-generation traffic control system by designing an intelligent adaptive motion control system for electric vehicles (EVs) which facilitates an EV to control its motion to align with the position where the electromagnetic strength is expected to be maximal to receive maximum charging efficiency. By combining the AI-based ACC system and the MARL-based intelligent lane-change system, our next-generation traffic control system for autonomous vehicles aims to revolutionize traffic management, offering improved efficiency and safety for autonomous vehicles on the roads of the future.
Library Comment
Dissertation or thesis originally submitted to ProQuest.
Notes
Embargoed until 07-10-2025
Recommended Citation
Das, Lokesh Chandra, "A Machine Learning-Driven Approach for Next-Generation Traffic Control System for Autonomous Vehicles" (2024). Electronic Theses and Dissertations. 3592.
https://digitalcommons.memphis.edu/etd/3592
Comments
Data is provided by the student.